Brain tumor grade classification using multi-step pre-training
INTERNATIONAL JOURNAL OF IMAGING SYSTEMS AND TECHNOLOGY(2023)
摘要
Medical images offer a non-invasive method to diagnose different diseases, but using them manually produces unreliable results. Modern deep learning architectures and techniques are computed and data-intensive, making them difficult to use for relatively smaller datasets of medical images. Transfer learning has been used as a remedy for the problem mentioned above. However, the domain difference between the datasets used for pre-training (e.g., ImageNet) and the target datasets, like medical images, negatively impacts the transfer learning results. Recently, many researchers have used additional pre-training called domain-adaptive pre-training (DAPT) using the data from the target domain (e.g., medical images) before using the model on the target tasks to achieve superior performance. This study proposes a variant of DAPT by performing it on a subset of the architecture. It has achieved state-of-the-art performance for brain tumor grading on the BraTS 2019 while being computationally efficient.
更多查看译文
关键词
brain tumor,computational efficiency,domain adaptive pre-training,transfer learning
AI 理解论文
溯源树
样例
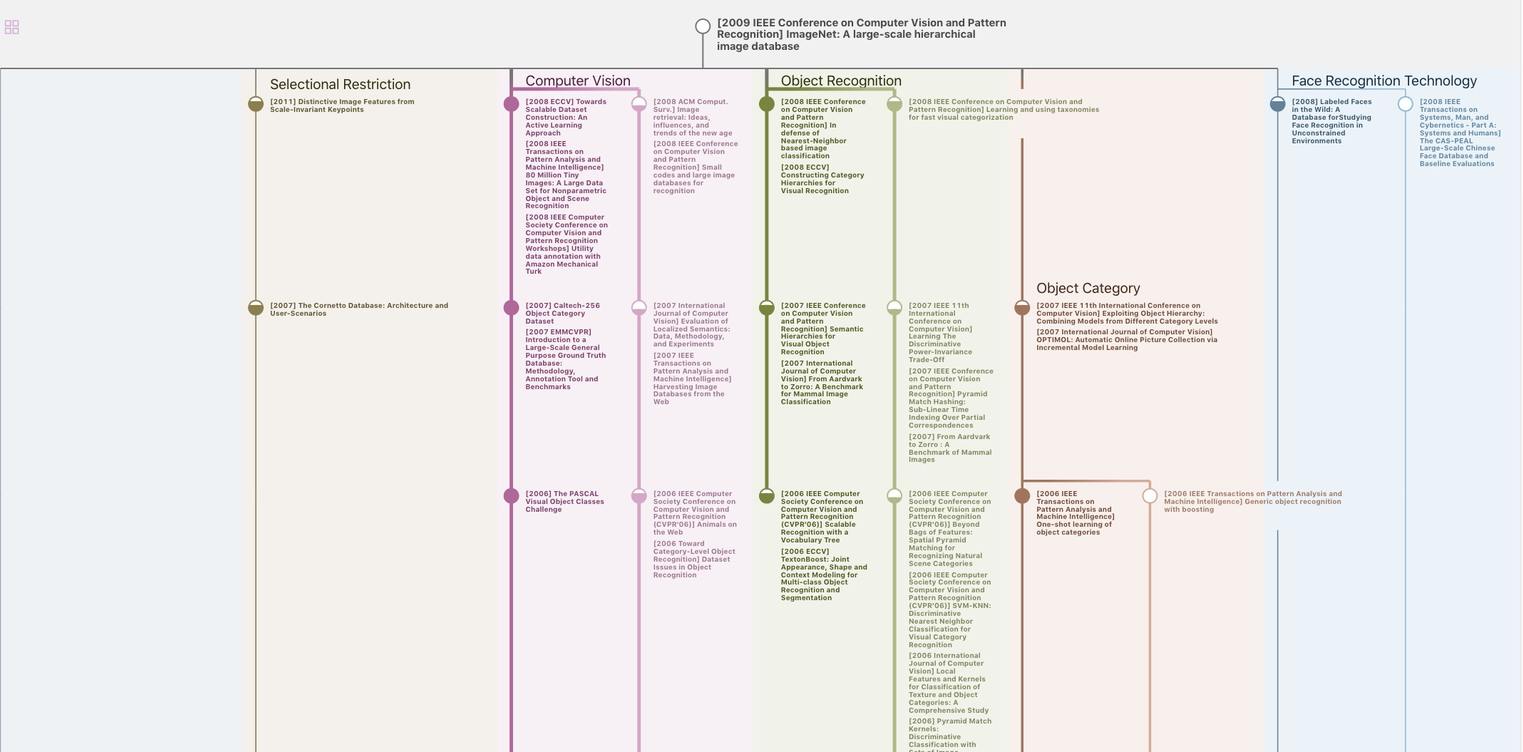
生成溯源树,研究论文发展脉络
Chat Paper
正在生成论文摘要