Temporal convolutional approach with residual multi-head attention mechanism for remaining useful life of manufacturing tools
ENGINEERING APPLICATIONS OF ARTIFICIAL INTELLIGENCE(2024)
摘要
Predicting the remaining useful life of manufacturing tools based on condition maintenance is a critical task in prognostics and health management systems. Existing methods have limitations in modeling variable-length sequence data and extracting sufficient local and global dependencies. To address these issues, a temporal convolutional approach with residual multi-head attention mechanism is proposed. The temporal convolution efficiently models variable-length sequence data and extracts long-term dependency features in the time dimension. The residual multi-head attention module captures local and global dependency features in the sequence features and extracts the tool key degradation features, which further improves the performance of the model. To validate the predictive performance of the temporal convolutional approach with residual multi-head attention mechanism, two manufacturing tool datasets are used in the remaining useful life experiments. The results demonstrate the effectiveness and superiority of the proposed method compared to other advanced methods.
更多查看译文
关键词
Deep learning,Temporal convolutional modeling,Residual multi-head attention mechanism,Manufacturing tool,Remaining useful life
AI 理解论文
溯源树
样例
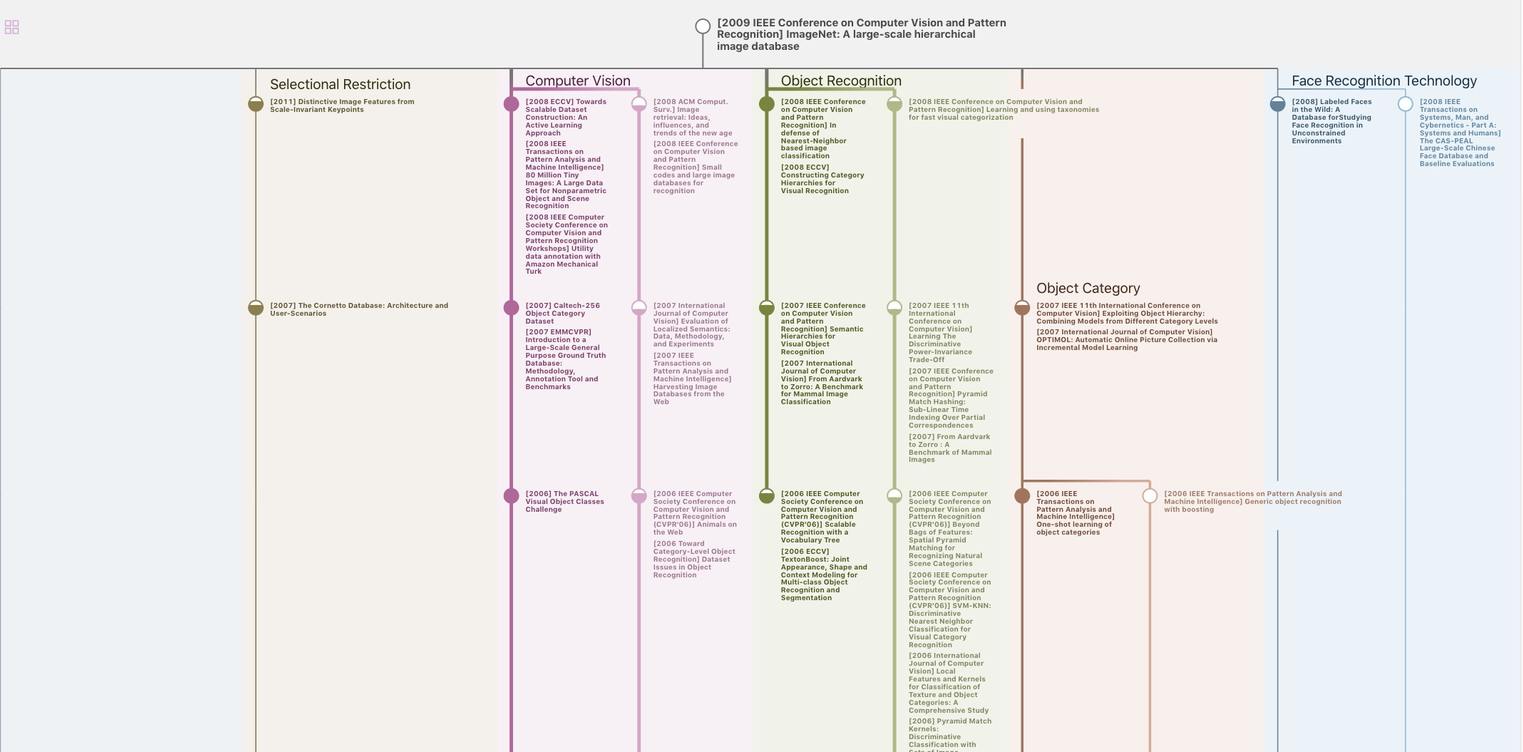
生成溯源树,研究论文发展脉络
Chat Paper
正在生成论文摘要