Two-layer networks with the ReLUk activation function: Barron spaces and derivative approximation
NUMERISCHE MATHEMATIK(2024)
摘要
We investigate the use of two-layer networks with the rectified power unit, which is called the ReLUk activation function, for function and derivative approximation. By extending and calibrating the corresponding Barron space, we show that two-layer networks with the ReLUk activation function are well-designed to simultaneously approximate an unknown function and its derivatives. When the measurement is noisy, we propose a Tikhonov type regularization method, and provide error bounds when the regularization parameter is chosen appropriately. Several numerical examples support the efficiency of the proposed approach.
更多查看译文
关键词
41A25,42B35,42C40,65D15,65D25
AI 理解论文
溯源树
样例
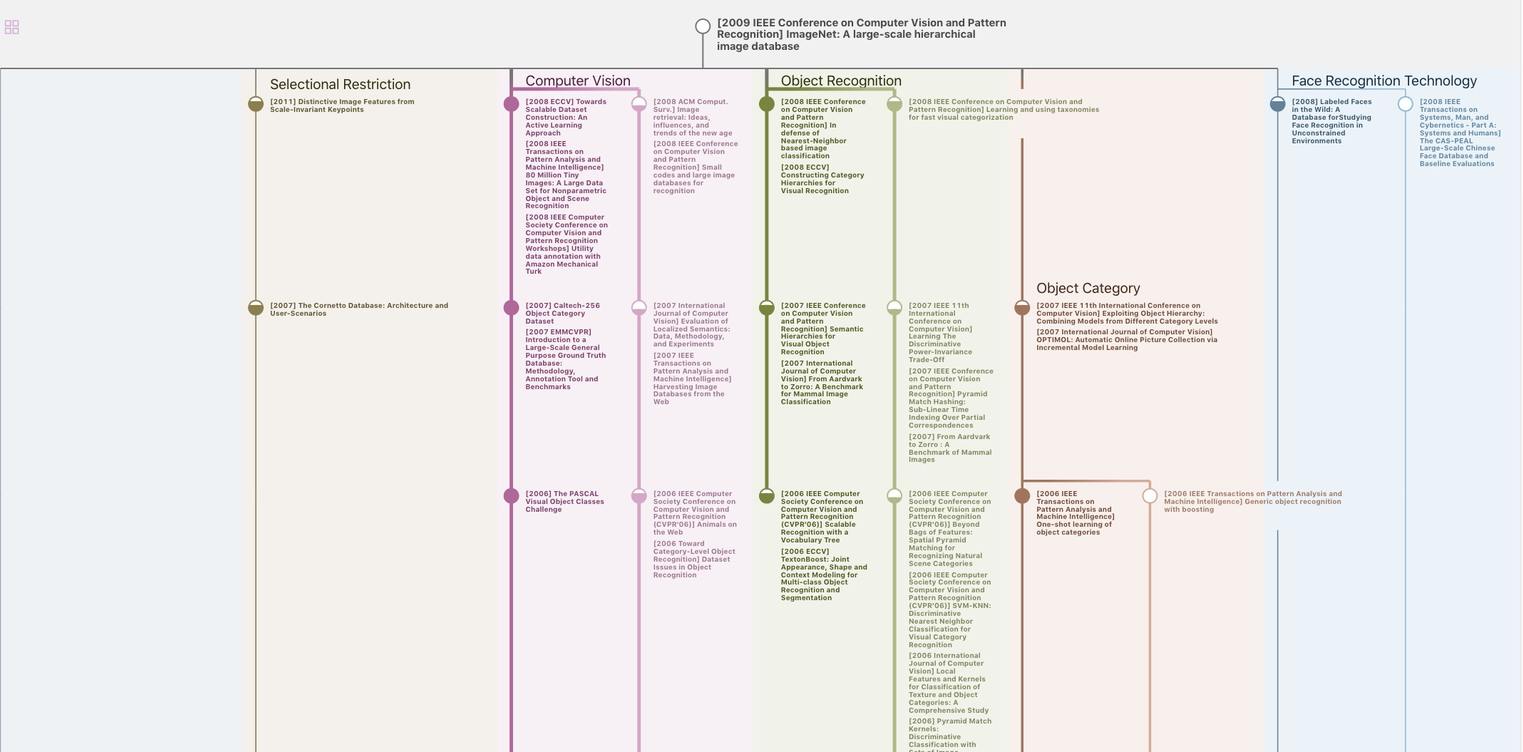
生成溯源树,研究论文发展脉络
Chat Paper
正在生成论文摘要