Temporal Attention Source-Free Adaptation for Chemical Processes Fault Diagnosis
IEEE TRANSACTIONS ON INDUSTRIAL INFORMATICS(2023)
摘要
Recently, domain adaptation (DA)-based fault diagnosis approaches have been actively studied in chemical processes to build a reliable fault diagnosis model for a new operating mode (i.e., target domain) by making use of labeled data from a historical mode (i.e., source domain). However, this raises privacy concerns, such as data leakage, since industrial data contains sensitive production information. Moreover, preprocessed source and target data used to train an effective target model will result in additional computational costs. Therefore, it is crucial to develop a novel privacy preserving DA-based fault diagnosis approach that can improve the diagnosis performance for a new mode and protect the privacy of a historical mode simultaneously. To this end, fault diagnosis is formulated as the source-free DA problem and proposes a temporal attention source-free adaptation (TASFA) algorithm, which only utilizes the pretrained source model and unlabeled target data to learn a diagnosis model. Specifically, for the time-series process, an attention mechanism is designed to capture and leverage the temporal correlations between source and target domains by extracting the most transferable information from the target time series. Empirical results on both the Tennessee Eastman process and the continuous stirred tank reactor demonstrate the effectiveness and efficiency of TASFA.
更多查看译文
关键词
Adaptation models,Data models,Feature extraction,Fault diagnosis,Time series analysis,Chemical processes,Chemicals,Chemical process fault diagnosis,privacy-preserving,source-free domain adaptation (SFDA),temporal attention
AI 理解论文
溯源树
样例
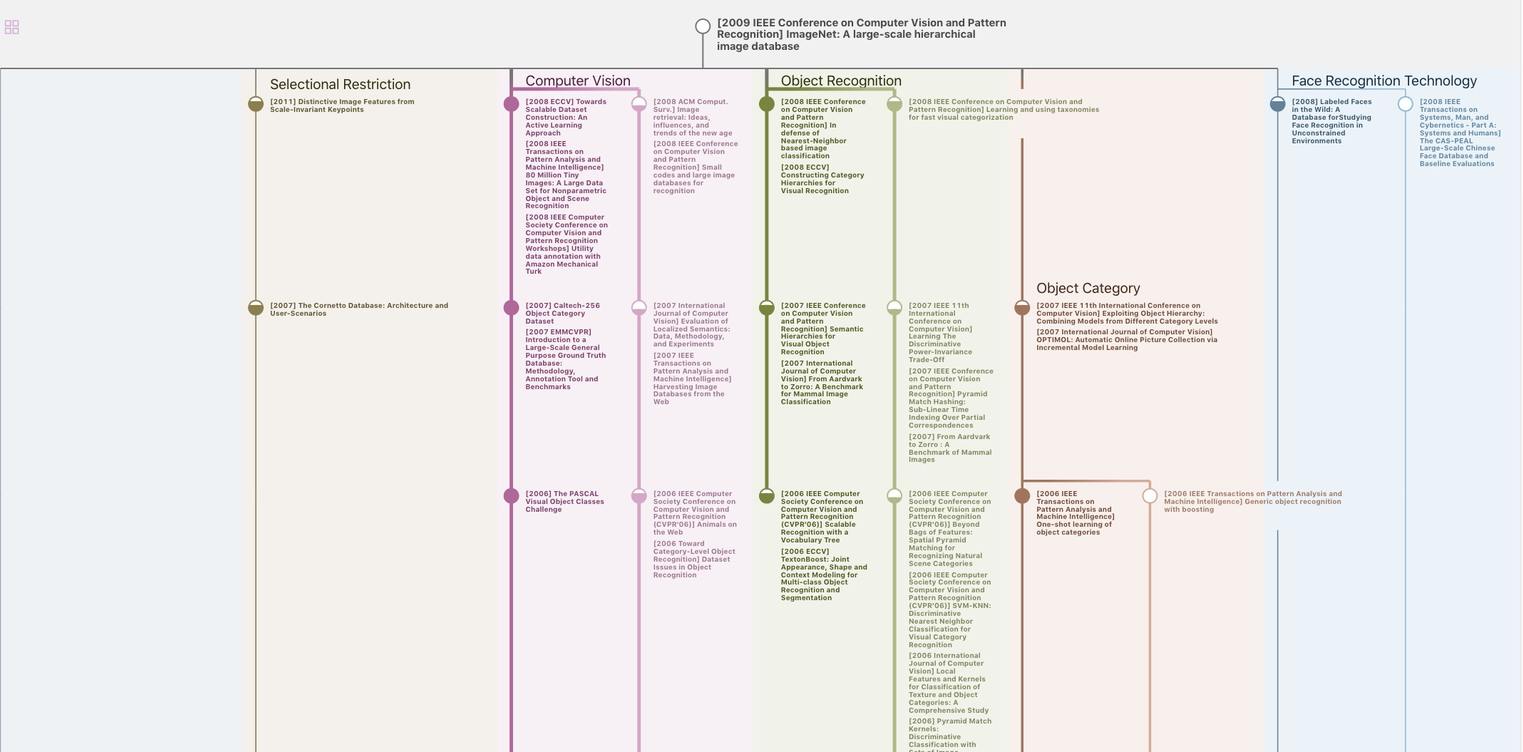
生成溯源树,研究论文发展脉络
Chat Paper
正在生成论文摘要