Multi-object Tracking with Spatial-Temporal Tracklet Association.
ACM Trans. Multim. Comput. Commun. Appl.(2024)
摘要
Recently, the tracking-by-detection methods have achieved excellent performance in Multi-Object Tracking (MOT), which focuses on obtaining a robust feature for each object and generating tracklets based on feature similarity. However, they are confronted with two issues: (1) unstable features in short-term occlusion and (2) insufficient matching in long-term occlusion. Specifically, the unstable feature is caused by the appearance variation under occlusion, and the association with the current unstable feature will lead to insufficient matching in long-term occlusion. To address the above issues, we propose a two-stage tracklet-level association method, Spatial-Temporal Tracklet Association (STTA), to effectively combine spatial-temporal context between feature extraction and data association. In the first stage, we propose the Tracklet-guided Spatial-Temporal Attention network (TSTA) to generate robust and stable features. Specifically, TSTA captures spatial-temporal context to obtain the most salient regions between the current and previous clips. In the second stage, we design the Bi-Tracklet Spatial-Temporal association (BTST) module to fully exploit the spatial-temporal context in data association. Specifically, we leverage BTST to merge different tracklets into long-term trajectories by jointly learning visual feature and spatial-temporal context and designing a bidirectional interpolation to recover the missed objects between matched tracklets. Extensive experiments of public and private detections on four benchmarks demonstrate the robustness of STTA. Furthermore, the proposed method is a model-agnostic method, which can be plugged and played with existing methods to boost their performance, e.g., obtain 11.0%, 10.1%, 2.9%, 3.2%, and 7.8% improvement on IDF1 in the MOT16 validation dataset for Tracktor, CenterTrack, Deepsort, JDE, and CTracker, respectively.
更多查看译文
关键词
Multi-object tracking,spatial-temporal tracklet association,tracklet-guided spatial-temporal attention network,bi-tracklet spatial-temporal association
AI 理解论文
溯源树
样例
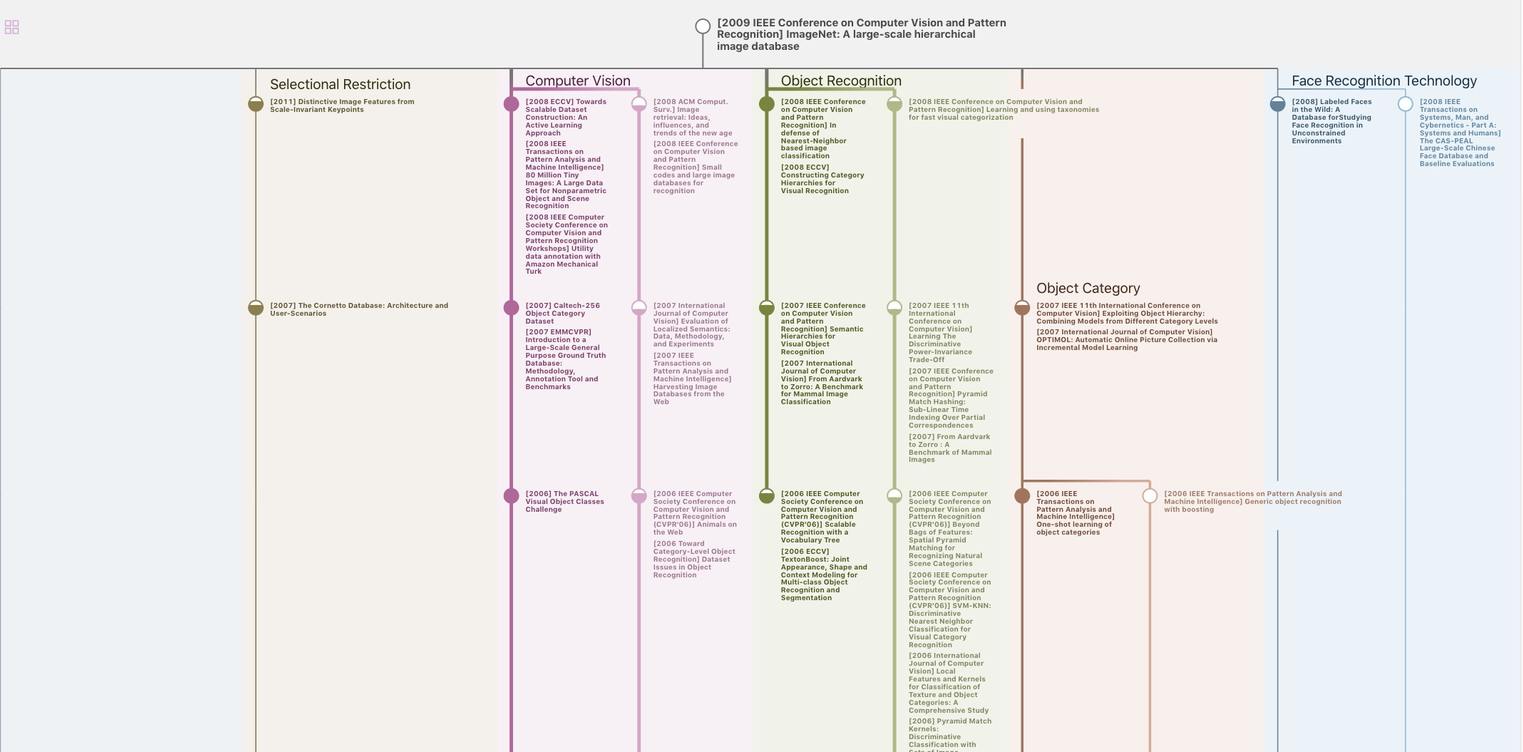
生成溯源树,研究论文发展脉络
Chat Paper
正在生成论文摘要