Chain of History: Learning and Forecasting with LLMs for Temporal Knowledge Graph Completion
CoRR(2024)
摘要
Temporal Knowledge Graph Completion (TKGC) is a challenging task of
predicting missing event links at future timestamps by leveraging established
temporal structural knowledge. Given the formidable generative capabilities
inherent in LLMs (LLMs), this paper proposes a novel approach to conceptualize
temporal link prediction as an event generation task within the context of a
historical event chain. We employ efficient fine-tuning methods to make LLMs
adapt to specific graph textual information and patterns discovered in temporal
timelines. Furthermore, we introduce structure-based historical data
augmentation and the integration of reverse knowledge to emphasize LLMs'
awareness of structural information, thereby enhancing their reasoning
capabilities. We conduct thorough experiments on multiple widely used datasets
and find that our fine-tuned model outperforms existing embedding-based models
on multiple metrics, achieving SOTA results. We also carry out sufficient
ablation experiments to explore the key influencing factors when LLMs perform
structured temporal knowledge inference tasks.
更多查看译文
AI 理解论文
溯源树
样例
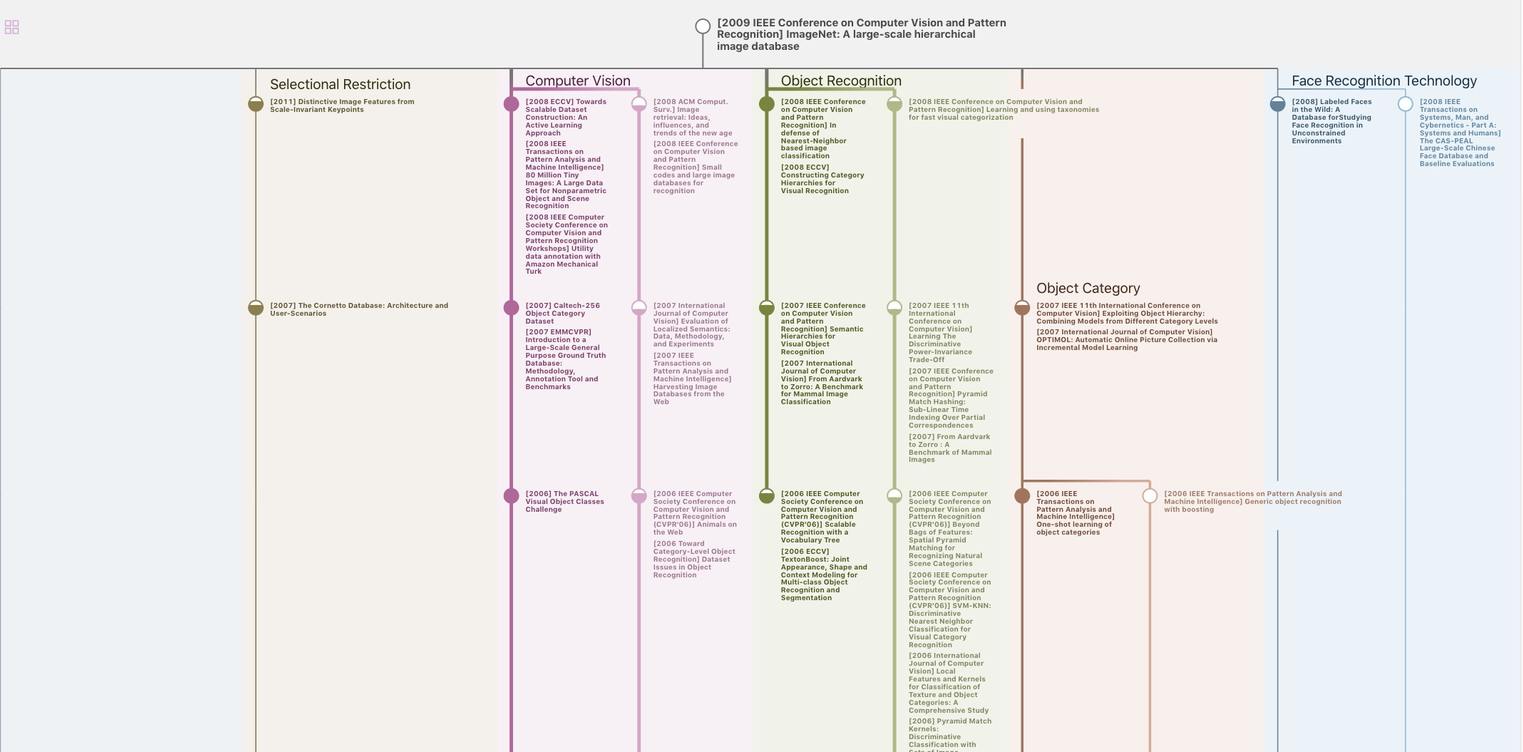
生成溯源树,研究论文发展脉络
Chat Paper
正在生成论文摘要