RiteWeight: Randomized Iterative Trajectory Reweighting for Steady-State Distributions Without Discretization Error
arxiv(2024)
摘要
Molecular dynamics (MD) and enhanced sampling simulations have become fundamental tools for studying biomolecular events. A significant challenge in these simulations is ensuring that sampled configurations and transitions converge to the stationary distribution of interest, whether equilibrium or nonequilibrium. Lack of convergence constrains the estimation of mechanisms, free energy, and rates of complex molecular events. Here, we introduce the "Randomized Iterative Trajectory Reweighting" (RiteWeight) algorithm to estimate a stationary distribution from unconverged simulation data. This method iteratively reweights trajectories in a self-consistent way by solving for the stationary distribution using a discrete-state transition matrix, employing a new random clustering in each iteration. The iterative random clustering mitigates the phase-space discretization error inherent in existing trajectory reweighting techniques based on one-shot clustering and ultimately yields numerically unbiased, quasi-continuous configuration-space distributions and estimates of observables. We demonstrate the efficacy of RiteWeight using Trp-Cage synthetic MD trajectories.
更多查看译文
AI 理解论文
溯源树
样例
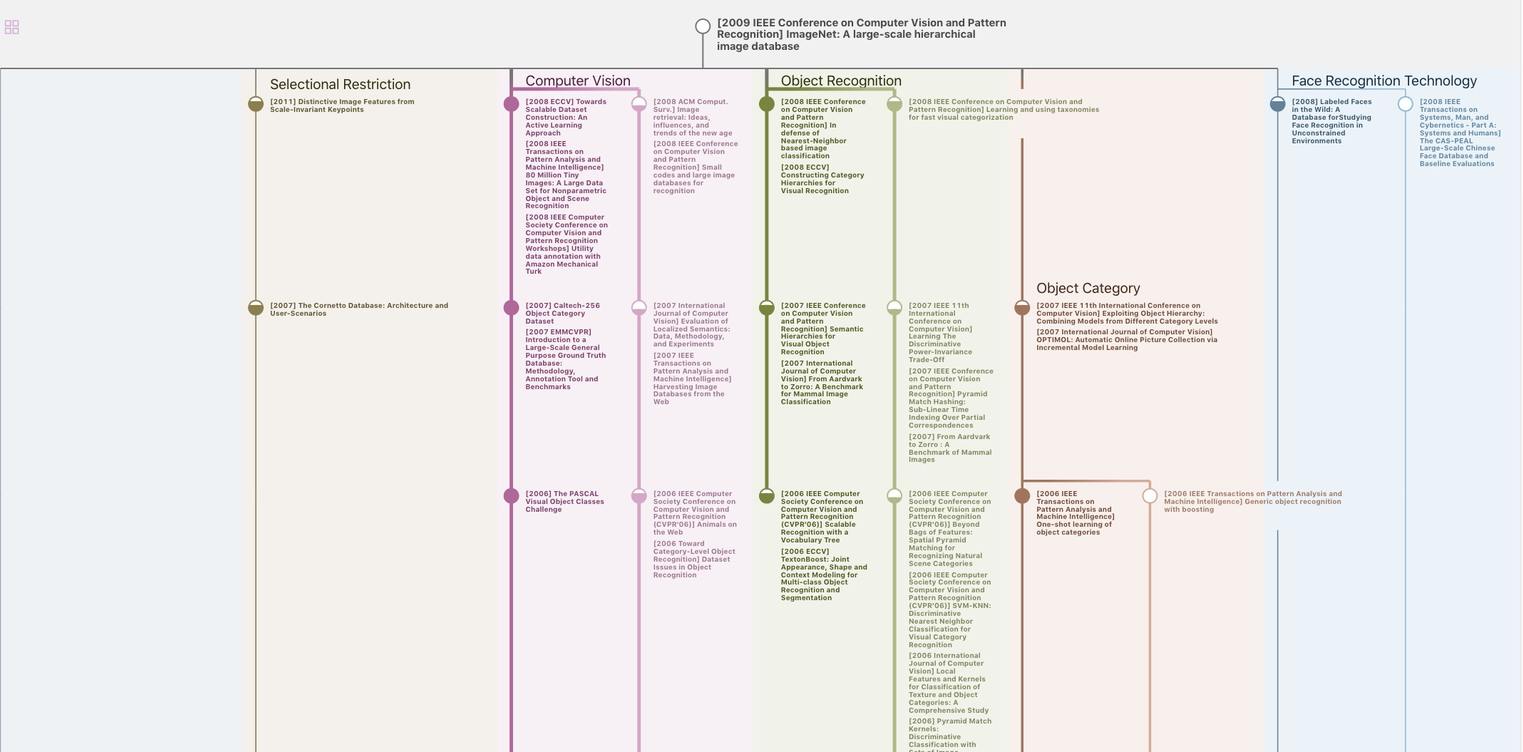
生成溯源树,研究论文发展脉络
Chat Paper
正在生成论文摘要