Synergetic Learning Neuro-Control for Unknown Affine Nonlinear Systems With Asymptotic Stability Guarantees
IEEE TRANSACTIONS ON NEURAL NETWORKS AND LEARNING SYSTEMS(2024)
摘要
For completely unknown affine nonlinear systems, in this article, a synergetic learning algorithm (SLA) is deve-loped to learn an optimal control. Unlike the conventional Hamilton-Jacobi-Bellman equation (HJBE) with system dynamics, a model-free HJBE (MF-HJBE) is deduced by means of off-policy reinforcement learning (RL). Specifically, the equivalence between HJBE and MF-HJBE is first bridged from the perspective of the uniqueness of the solution of the HJBE. Furthermore, it is proven that once the solution of MF-HJBE exists, its corresponding control input renders the system asymptotically stable and optimizes the cost function. To solve the MF-HJBE, the two agents composing the synergetic learning (SL) system, the critic agent and the actor agent, can evolve in real-time using only the system state data. By building an experience reply (ER)-based learning rule, it is proven that when the critic agent evolves toward the optimal cost function, the actor agent not only evolves toward the optimal control, but also guarantees the asymptotic stability of the system. Finally, simulations of the F16 aircraft system and the Van der Pol oscillator are conducted and the results support the feasibility of the developed SLA.
更多查看译文
关键词
Approximate dynamic programming (ADP),neural network,off-policy,optimal control,reinforcement learning (RL)
AI 理解论文
溯源树
样例
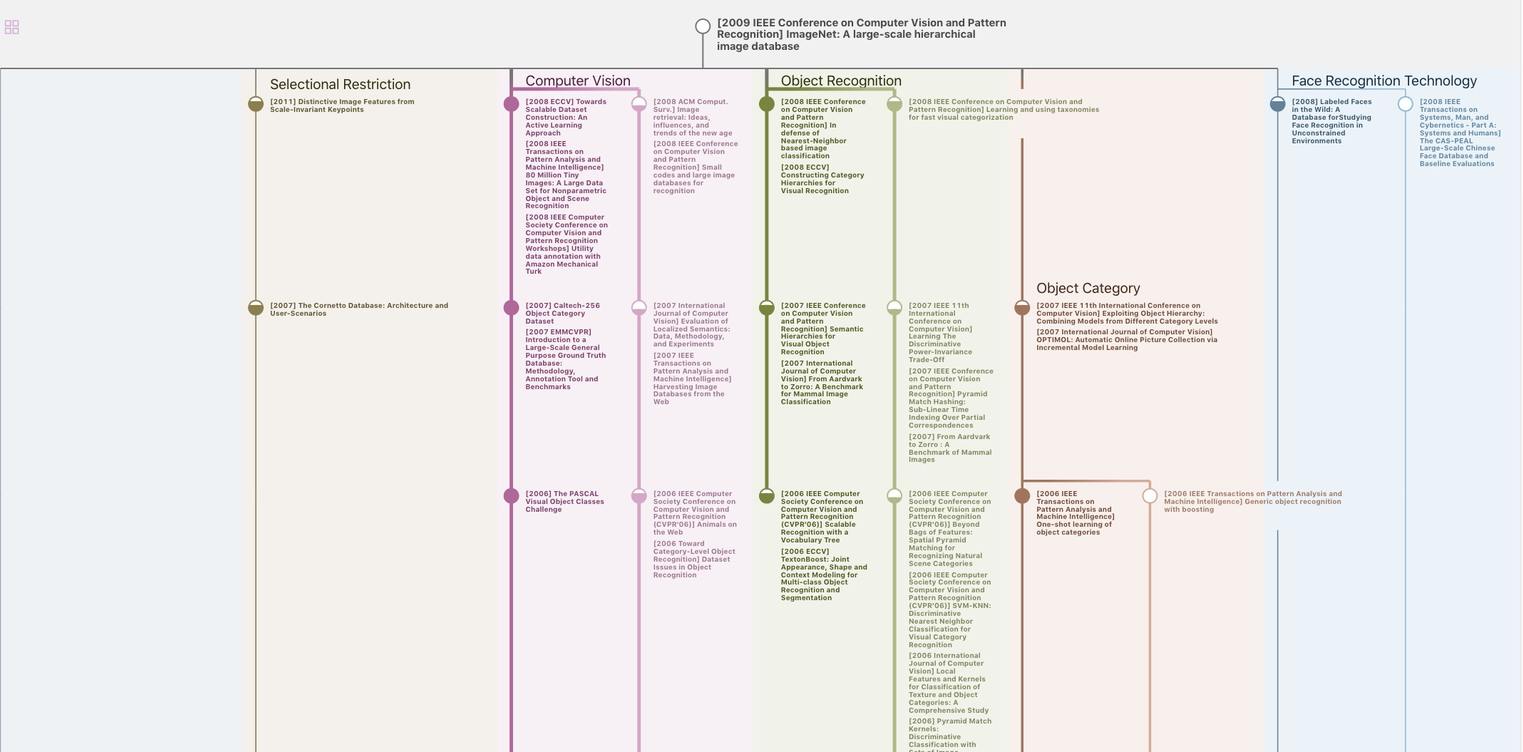
生成溯源树,研究论文发展脉络
Chat Paper
正在生成论文摘要