Imbalance Mitigation for Continual Learning via Knowledge Decoupling and Dual Enhanced Contrastive Learning
IEEE TRANSACTIONS ON NEURAL NETWORKS AND LEARNING SYSTEMS(2024)
摘要
Continual learning (CL) aims at studying how to learn new knowledge continuously from data streams without catastrophically forgetting the previous knowledge. One of the key problems is catastrophic forgetting, that is, the performance of the model on previous tasks declines significantly after learning the subsequent task. Several studies addressed it by replaying samples stored in the buffer when training new tasks. However, the data imbalance between old and new task samples results in two serious problems: information suppression and weak feature discriminability. The former refers to the information in the sufficient new task samples suppressing that in the old task samples, which is harmful to maintaining the knowledge since the biased output worsens the consistency of the same sample's output at different moments. The latter refers to the feature representation being biased to the new task, which lacks discrimination to distinguish both old and new tasks. To this end, we build an imbalance mitigation for CL (IMCL) framework that incorporates a decoupled knowledge distillation (DKD) approach and a dual enhanced contrastive learning (DECL) approach to tackle both problems. Specifically, the DKD approach alleviates the suppression of the new task on the old tasks by decoupling the model output probability during the replay stage, which better maintains the knowledge of old tasks. The DECL approach enhances both low-and high-level features and fuses the enhanced features to construct contrastive loss to effectively distinguish different tasks. Extensive experiments on three popular datasets show that our method achieves promising performance under task incremental learning (Task-IL), class incremental learning (Class-IL), and domain incremental learning (Domain-IL) settings.
更多查看译文
关键词
Task analysis,Knowledge engineering,Feature extraction,Streams,Maintenance engineering,Data models,Adaptation models,Catastrophic forgetting,continual learning (CL),experience replay (ER),image classification
AI 理解论文
溯源树
样例
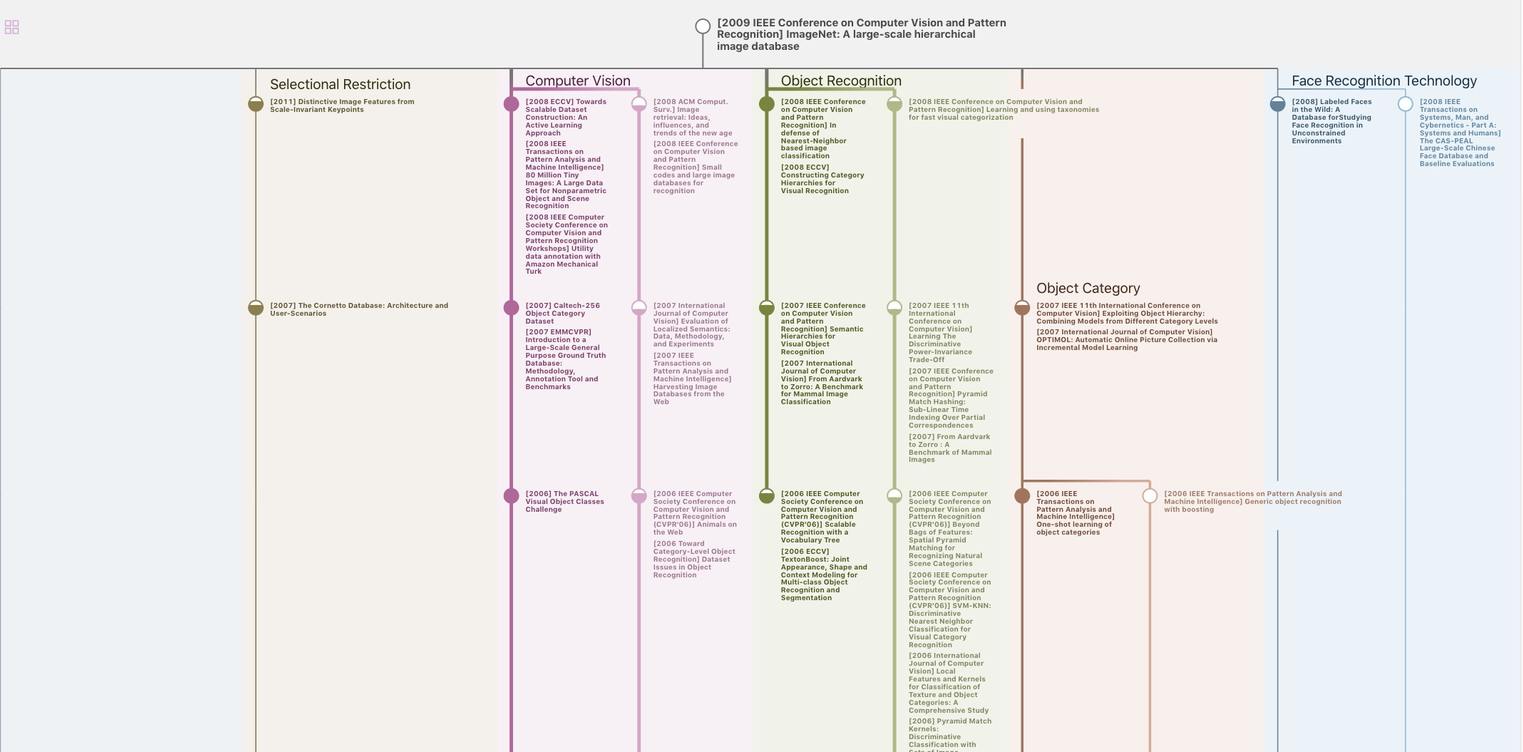
生成溯源树,研究论文发展脉络
Chat Paper
正在生成论文摘要