Platform-independent estimation of human physiological time from single blood samples
PROCEEDINGS OF THE NATIONAL ACADEMY OF SCIENCES OF THE UNITED STATES OF AMERICA(2024)
摘要
Abundant epidemiological evidence links circadian rhythms to human health, from heart disease to neurodegeneration. Accurate determination of an individual's circadian phase is critical for precision diagnostics and personalized timing of therapeutic interventions. To date, however, we still lack an assay for physiological time that is accurate, minimally burdensome to the patient, and readily generalizable to new data. Here, we present Time Machine, an algorithm to predict the human circadian phase using gene expression in peripheral blood mononuclear cells from a single blood draw. Once trained on data from a single study, we validated the trained predictor against four independent datasets with distinct experimental protocols and assay platforms, demonstrating that it can be applied generalizably. Importantly, Time Machine predicted circadian time with a median absolute error ranging from1.65 to 2.7 h, regardless of systematic differences in experimental protocol and assay platform, without renormalizing the data or retraining the predictor. This feature enables it to be flexibly applied to both new samples and existing data without limitations on the transcriptomic profiling technology (microarray, RNAseq). We benchmark Time Machine against competing approaches and identify the algorithmic features that contribute to its performance.
更多查看译文
关键词
circadian rhythms,machine learning,transcriptomics,cross-platform prediction
AI 理解论文
溯源树
样例
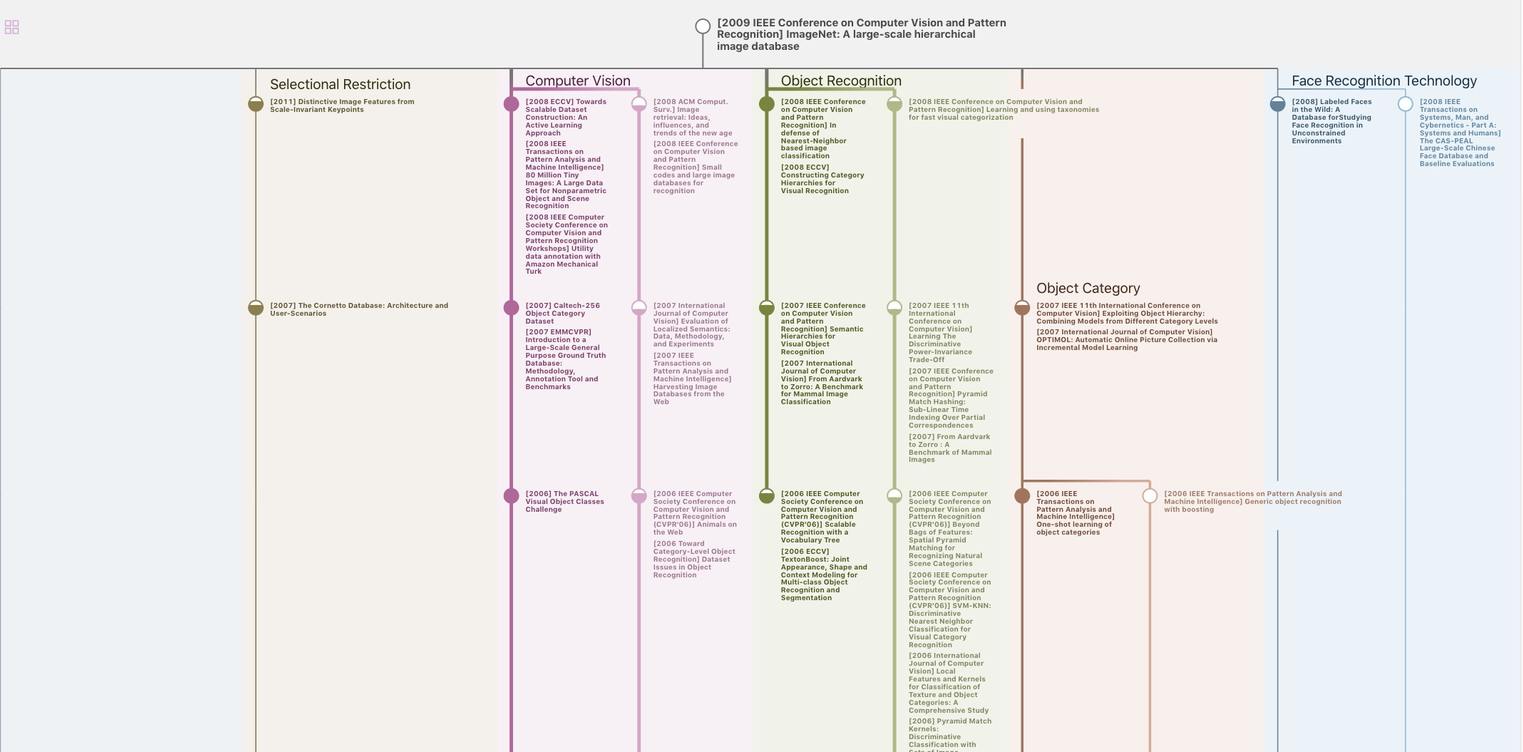
生成溯源树,研究论文发展脉络
Chat Paper
正在生成论文摘要