Twitter Disaster Prediction Using Different Deep Learning Models
SN Computer Science(2024)
摘要
In emergencies, Twitter has become an essential way for people to communicate. Since many people use smartphones, they can easily report events they see. Because of this, more organizations are using Twitter to monitor what is happening digitally. Twitter and similar online platforms provide a place to share their personal experiences and local events with others. People often post about their daily lives, things happening in their neighborhoods, and their activities to keep others informed. In this study, we focus on predicting disasters on Twitter using an improved Bidirectional Encoder Depiction from the Transformers (BERT) model. Twitter has become a crucial communication tool during emergencies, allowing people to report events they witness quickly. As a result, organizations are increasingly using Twitter for digital monitoring. Our research utilizes a modified version of the BERT model, a powerful language representation technique. This modified BERT model enables us to understand better tweets related to disasters and improve the accuracy of our predictions. Using Twitter data, we train our improved BERT model to recognize patterns and signals associated with different types of disasters, such as natural calamities or accidents. We aim to efficiently detect and respond to potential disasters in real time by doing so. Our experiments' results demonstrate our BERT effectiveness, showing improved disaster prediction performance compared to traditional models like the long short-term memory model (LSTM) and gated recurrent unit model (GRU). This research contributes valuable insights into disaster management strategies and allows leveraging social media data in disaster preparedness and response efforts.
更多查看译文
关键词
Long short-term memory model,Gated recurrent unit model,Bidirectional encoder depiction from the transformers
AI 理解论文
溯源树
样例
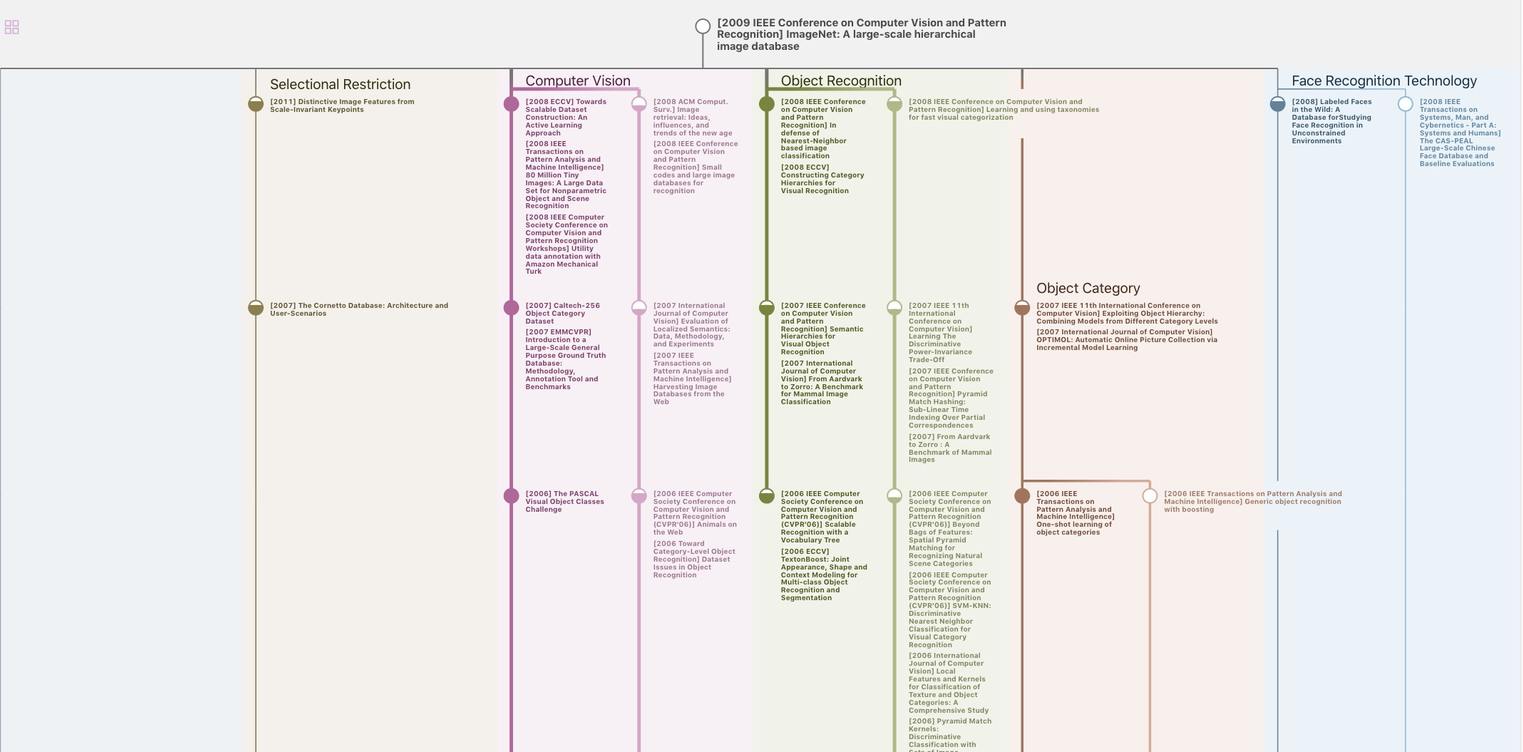
生成溯源树,研究论文发展脉络
Chat Paper
正在生成论文摘要