Knowledge-aware Graph Transformer for Pedestrian Trajectory Prediction
CoRR(2024)
摘要
Predicting pedestrian motion trajectories is crucial for path planning and motion control of autonomous vehicles. Accurately forecasting crowd trajectories is challenging due to the uncertain nature of human motions in different environments. For training, recent deep learning-based prediction approaches mainly utilize information like trajectory history and interactions between pedestrians, among others. This can limit the prediction performance across various scenarios since the discrepancies between training datasets have not been properly incorporated. To overcome this limitation, this paper proposes a graph transformer structure to improve prediction performance, capturing the differences between the various sites and scenarios contained in the datasets. In particular, a self-attention mechanism and a domain adaption module have been designed to improve the generalization ability of the model. Moreover, an additional metric considering cross-dataset sequences is introduced for training and performance evaluation purposes. The proposed framework is validated and compared against existing methods using popular public datasets, i.e., ETH and UCY. Experimental results demonstrate the improved performance of our proposed scheme.
更多查看译文
关键词
Trajectory Prediction,Pedestrian Trajectory,Pedestrian Trajectory Prediction,Predictive Performance,Domain Adaptation,Self-attention Mechanism,Social Interaction,Average Error,Temporal Dimension,Recurrent Neural Network,Spatial Dimensions,Attention Mechanism,Spatial Domain,Nodes In The Graph,Transformer Model,Graph Convolutional Network,Spatial Interaction,Graph Neural Networks,Bivariate Distribution,Graph Convolution,Maximum Mean Discrepancy,Temporal Graph,Graph Operations,Mixed Loss,Weighted Adjacency Matrix,Variance Of The Gaussian Distribution,Convolution Operation,Neural Network,Time Step,Loss Function
AI 理解论文
溯源树
样例
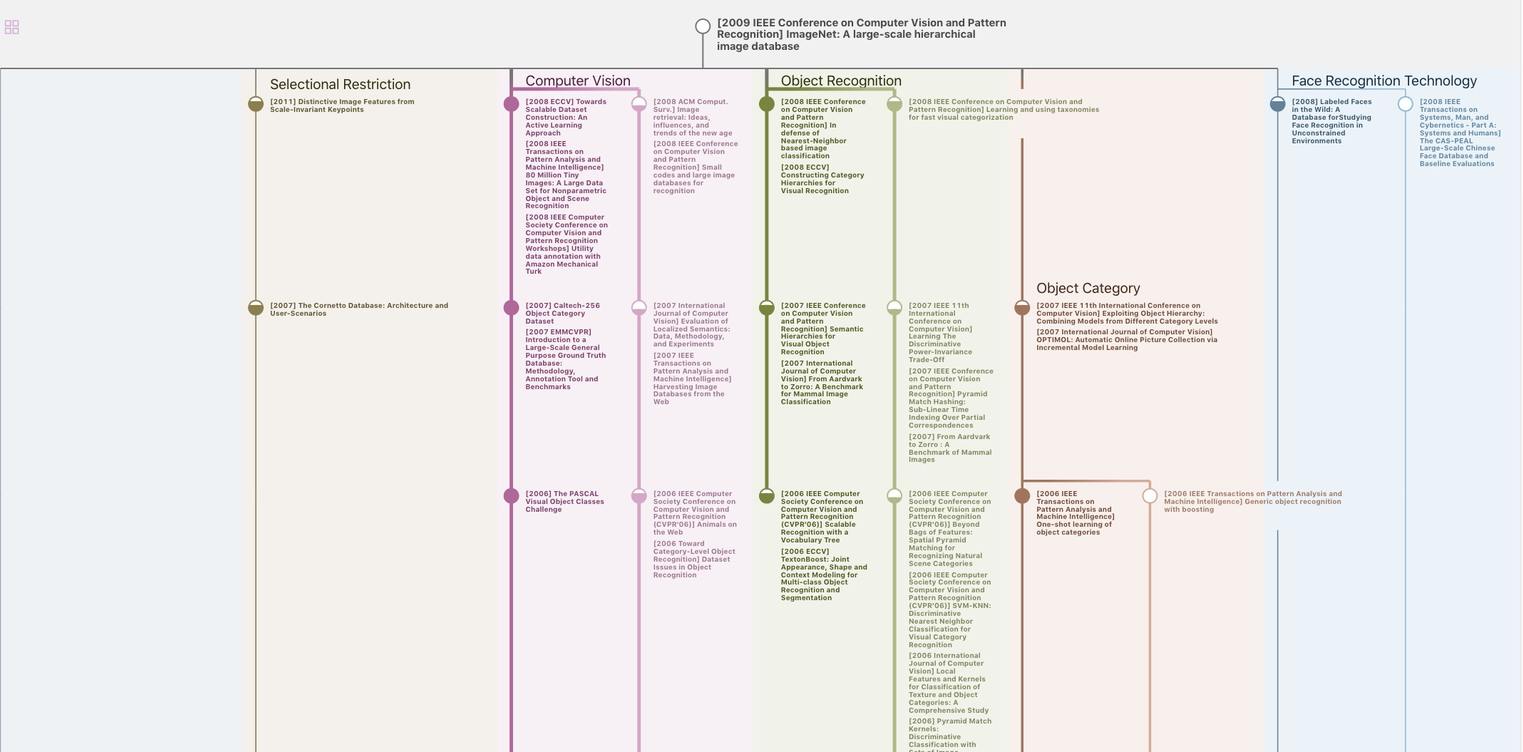
生成溯源树,研究论文发展脉络
Chat Paper
正在生成论文摘要