Cascading Machine Learning to Monitor Volcanic Thermal Activity Using Orbital Infrared Data: From Detection to Quantitative Evaluation
REMOTE SENSING(2024)
摘要
Several satellite missions are currently available to provide thermal infrared data at different spatial resolutions and revisit time. Furthermore, new missions are planned thus enabling to keep a nearly continuous 'eye' on thermal volcanic activity around the world. This massive volume of data requires the development of artificial intelligence (AI) techniques for the automatic processing of satellite data in order to extract significant information about volcano conditions in a short time. Here, we propose a robust machine learning approach to accurately detect, recognize and quantify high-temperature volcanic features using Sentinel-2 MultiSpectral Instrument (S2-MSI) imagery. We use the entire archive of high spatial resolution satellite data containing more than 6000 S2-MSI scenes at ten different volcanoes around the world. Combining a 'top-down' cascading architecture, two different machine learning models, a scene classifier (SqueezeNet) and a pixel-based segmentation model (random forest), we achieved a very high accuracy, namely 95%. These results show that the cascading approach can be applied in near-real time to any available satellite image, providing a full description of the scene, with an important contribution to the monitoring, mapping and characterization of volcanic thermal features.
更多查看译文
关键词
volcano,satellite remote sensing,cascading ensemble technique,transfer learning,Sentinel 2-MSI
AI 理解论文
溯源树
样例
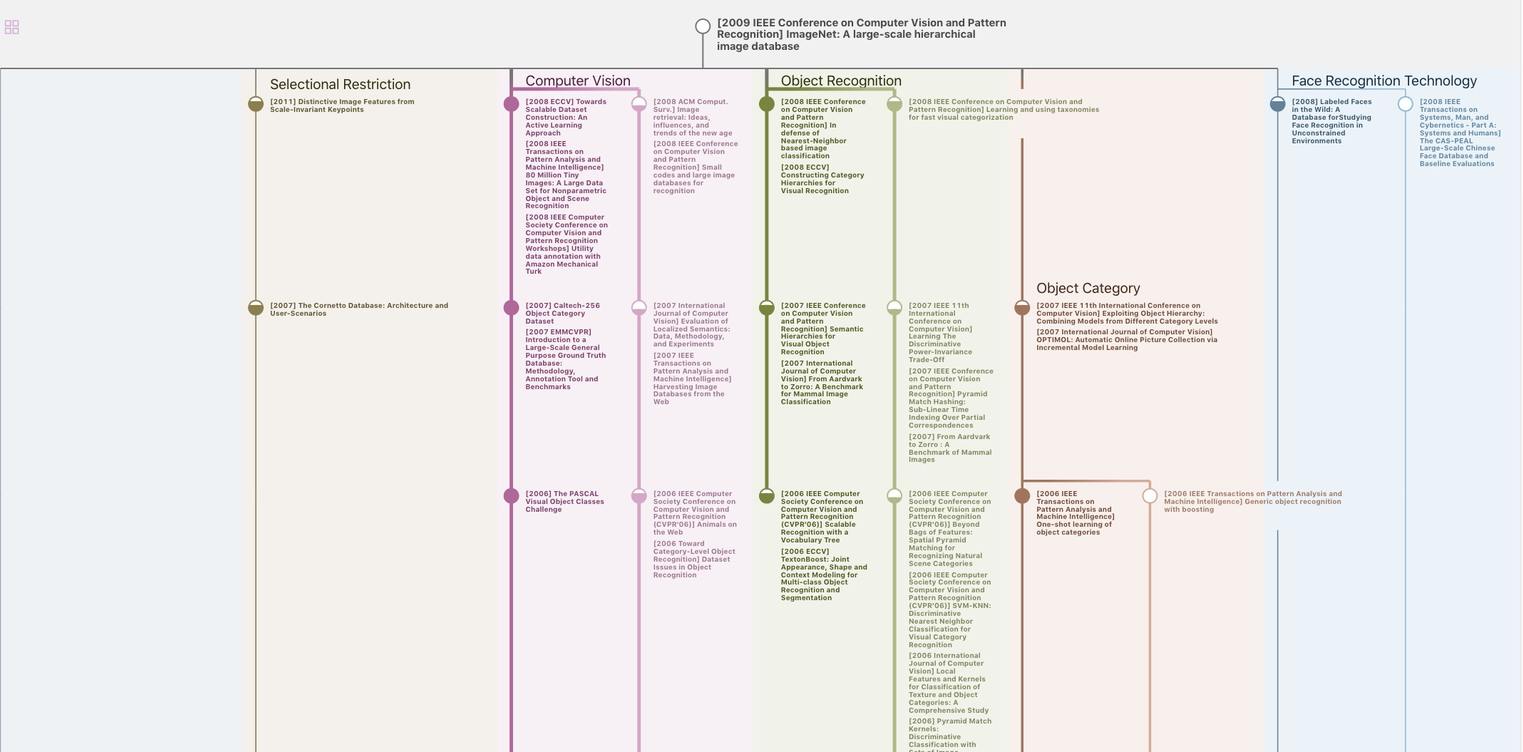
生成溯源树,研究论文发展脉络
Chat Paper
正在生成论文摘要