Adaptively Optimized Masking EMD for Separating Intrinsic Oscillatory Modes of Nonstationary Signals
IEEE SIGNAL PROCESSING LETTERS(2024)
摘要
Empirical mode decomposition (EMD) is a well-established technique to decompose nonstationary signals into intrinsic oscillatory modes, which is essential to catch crucial information hidden in nonstationary signals. However, when decomposing signals with intermittency or closely spaced frequency modes, EMD suffers from severe mode mixing problems (MMP). Therefore, we develop a novel adaptively optimized masking EMD (AOMEMD), a generalized, automated method to solve MMP. The work focuses on two aspects: i) Masking signals with different scales are adaptively iteratively generated based on Hilbert analytic solution and applied to raw data to assist EMD in solving MMP. ii) A universal mode mixing measure index (UMI) is proposed to quantitatively evaluate the extent of mode mixing. An optimization routine is then developed to optimize the amplitude and frequency of masking signals with the objective of minimizing the UMI to further improve the frequency separation performance of AOMEMD. Experiments on synthetic and real-world signals demonstrate that AOMEMD outperforms EMD and its variants in effectively addressing MMP, yielding more reasonable and reliable results. AOMEMD can separate modes with frequency ratios up to 0.9.
更多查看译文
关键词
Optimization,Indexes,Signal processing algorithms,Power system reliability,Oscillators,Flowcharts,Filtering,Nonstationary signals,EMD,mode mixing problem,masking signals,universal mode mixing measure index
AI 理解论文
溯源树
样例
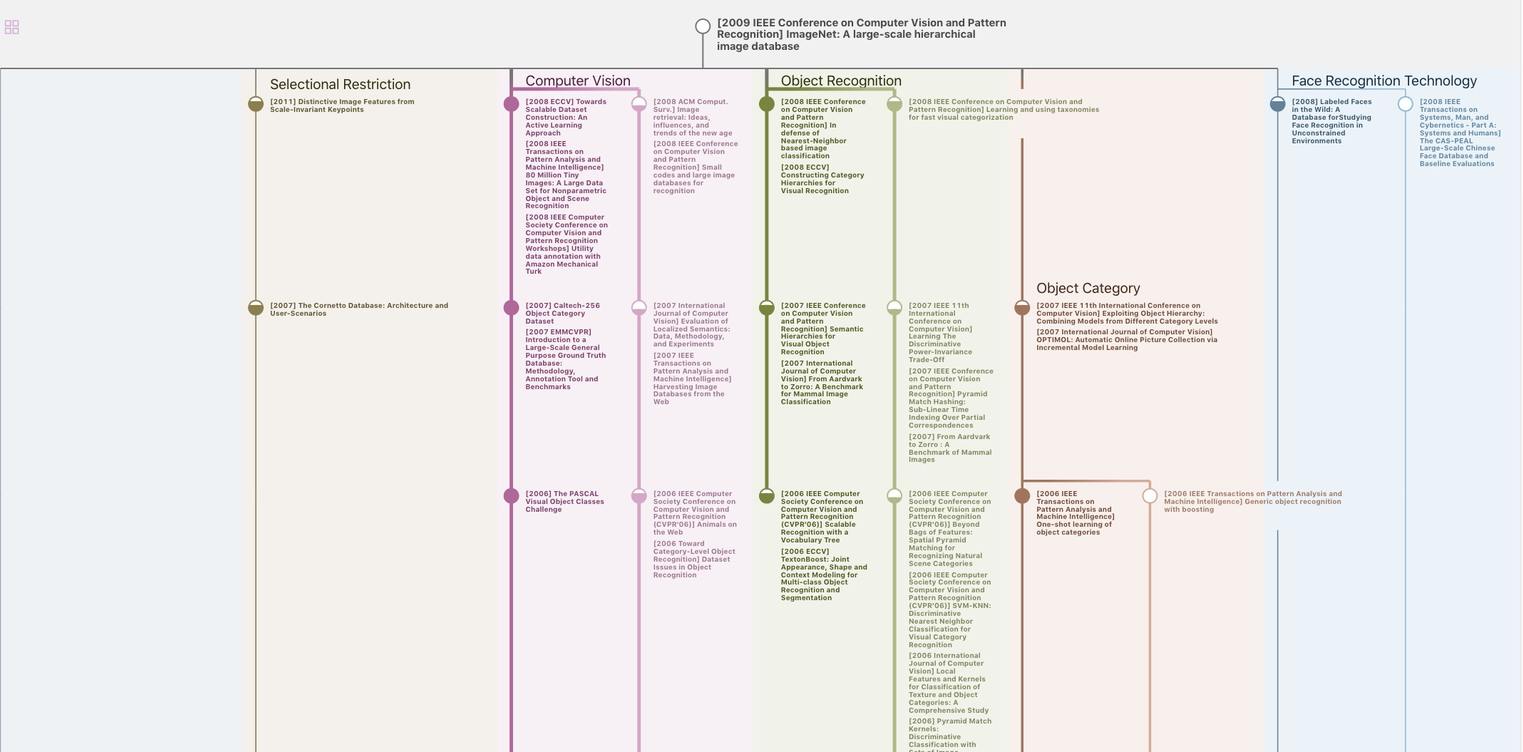
生成溯源树,研究论文发展脉络
Chat Paper
正在生成论文摘要