A Dynamic Memory for Reservoir Computing Utilizing Ion Migration in CuInP2S6
ADVANCED ELECTRONIC MATERIALS(2024)
摘要
Time-series analysis and forecasting play a vital role in the fields of economics and engineering. Neuromorphic computing, particularly recurrent neural networks (RNNs), has emerged as an effective approach to address these tasks. Reservoir computing (RC), a type of RNN, offers a powerful and efficient solution for handling nonlinear information in high-dimensional spaces and addressing temporal tasks. CuInP2S6 (CIPS), a van der Waals material with ion conductivity, shows promise for sequential task processing. Here, a synapse device based on CIPS is demonstrated that exhibits temporal dynamics under electrical stimulation. By controlling Cu+ ion migration, this study successfully emulates synaptic performance, including potentiation and depression characteristics, and RC. Migration of Cu+ ions is confirmed using piezoresponse and Kelvin probe force microscopy. The device achieves low normalized root mean square errors (NRMSE) of 0.04762 and 0.01402 for the Henon map and Mackey-Glass series tasks, respectively. For real-life time-series prediction based on the Jena temperature database, an overall NRMSE of 0.03339 is achieved. These results highlight the potential of CIPS ion conductivity for real-time signal processing in machine learning, expanding applications in neuromorphic computing.
更多查看译文
关键词
2D materials,copper ion conductivity,CuInP2S6,reservoir computing,temporal signal tasks
AI 理解论文
溯源树
样例
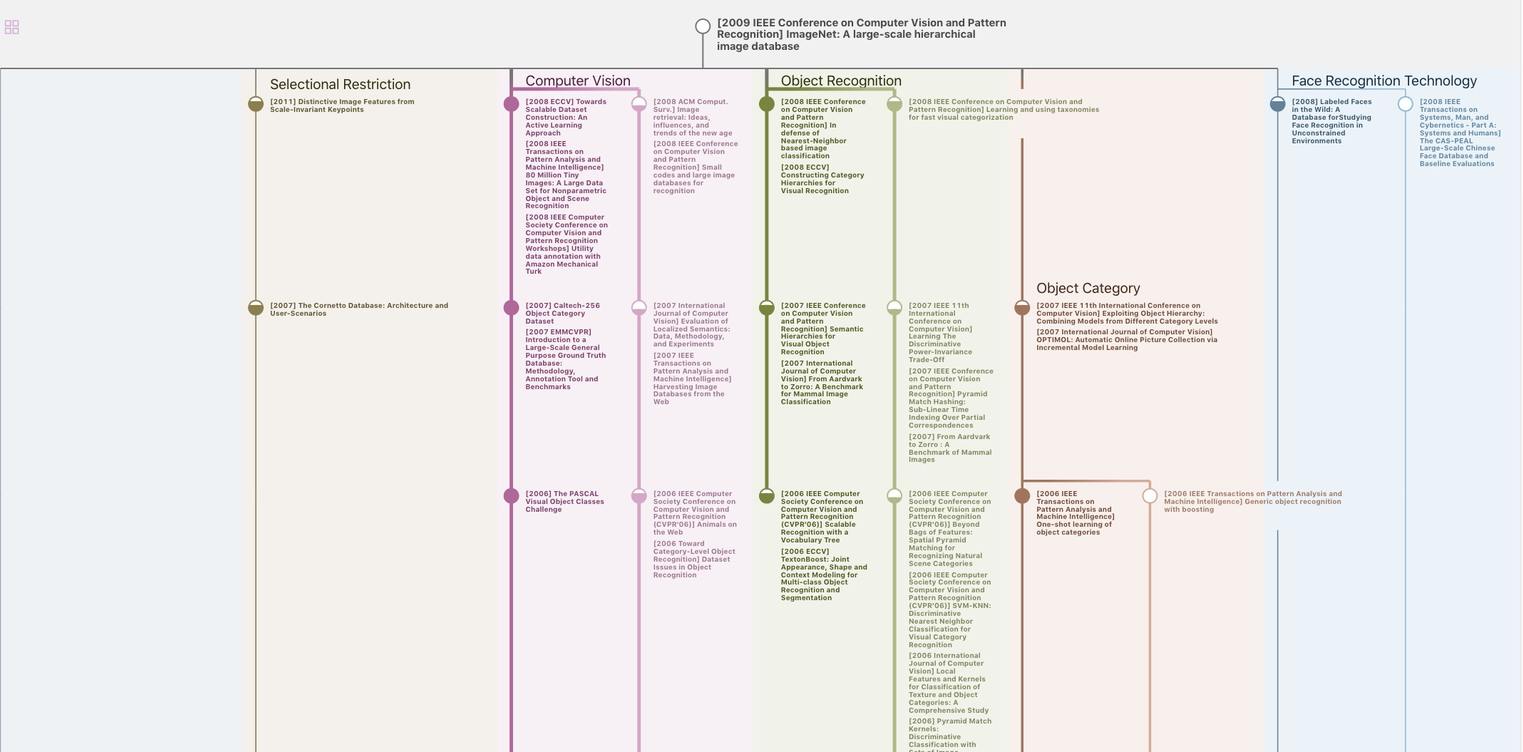
生成溯源树,研究论文发展脉络
Chat Paper
正在生成论文摘要