Bridging Machine Learning and Clinical Diagnosis: An Explainable Biomarker for ß-Amyloid PET Imaging
arxiv(2023)
摘要
[18F]-florbetaben positron emission tomography (PET) imaging is an
established marker of ß-Amyloid (Aß) that is being increasingly used to
assess Aß deposition in AD. This study presents a novel, explainable
machine learning-based biomarker for assessing Aß+ positivity based on
[18F]-florbetaben PET scans. We analyzed 163 scans acquired at our institution
as part of a retrospective analysis. Initially, we used the regional loadings
to train a Cubic Support Vector Machine (SVM) classifier and tested the model
using 5-fold cross-validation. To elucidate the model's decision-making process
in aggregate, we employed local interpretable model-agnostic explanations
(LIME), projecting the most influential features back onto the AAL atlas for
visualization. The Cubic SVM classifier demonstrated robust performance,
achieving 92.0
LIME analysis revealed that the model identified critical regions impacting
Aß+ status, with notable contributions from the inferior frontal cortex,
cuneus, olfactory cortex, postcentral gyrus, supramarginal gyrus, temporal
pole, thalamus, and pallidum. The model showcases high accuracy, paralleling
expert interpretation levels, and provides critical insights into the brain
regions most affected by amyloid deposition. By elucidating the relationship
between overall Aß+ status and specific brain regions, our approach holds
promise for novel insights into the correlation between the spatial
distribution of Aß and the clinical manifestations of AD.
更多查看译文
AI 理解论文
溯源树
样例
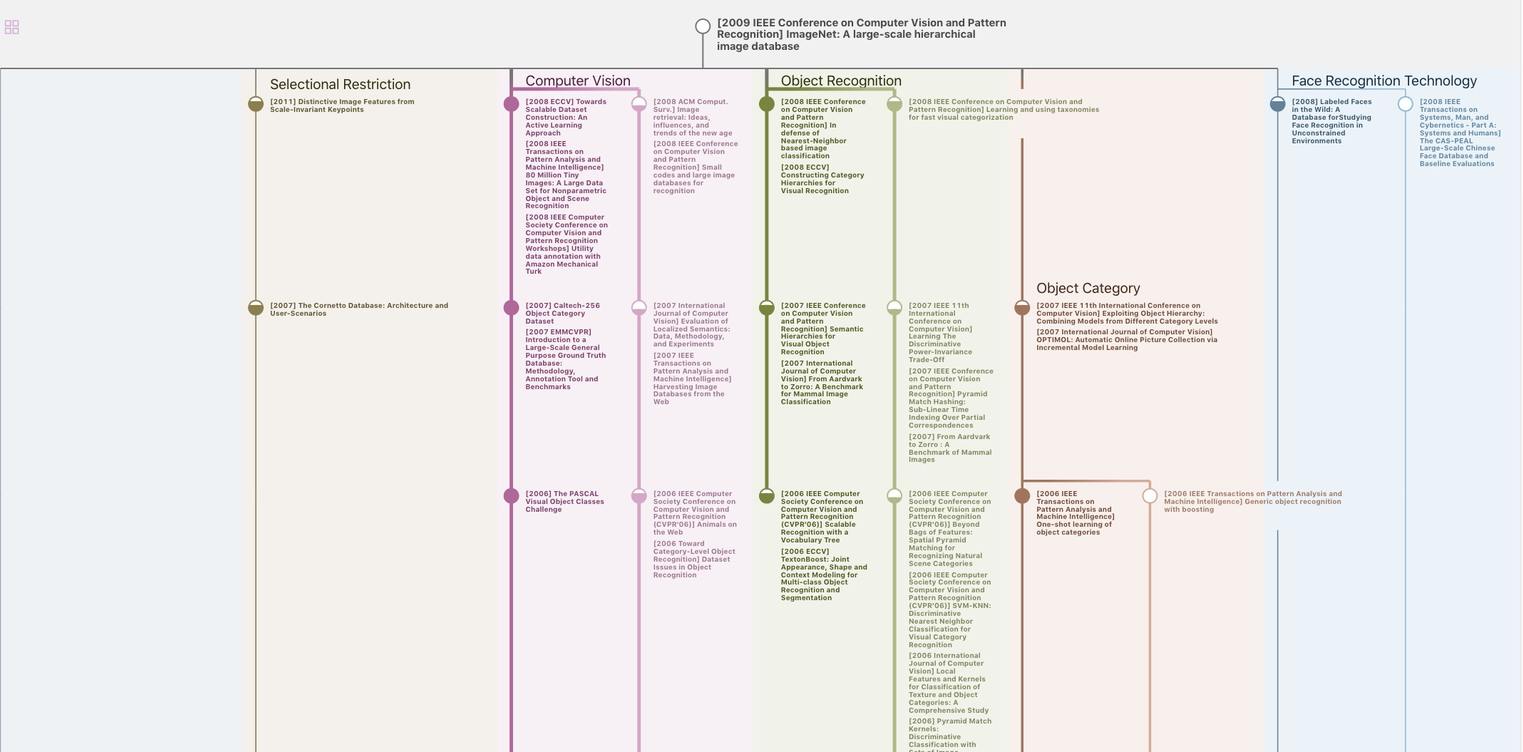
生成溯源树,研究论文发展脉络
Chat Paper
正在生成论文摘要