Predicting the structure of dynamic graphs
CoRR(2024)
摘要
Dynamic graph embeddings, inductive and incremental learning facilitate
predictive tasks such as node classification and link prediction. However,
predicting the structure of a graph at a future time step from a time series of
graphs, allowing for new nodes has not gained much attention. In this paper, we
present such an approach. We use time series methods to predict the node degree
at future time points and combine it with flux balance analysis – a linear
programming method used in biochemistry – to obtain the structure of future
graphs. Furthermore, we explore the predictive graph distribution for different
parameter values. We evaluate this method using synthetic and real datasets and
demonstrate its utility and applicability.
更多查看译文
AI 理解论文
溯源树
样例
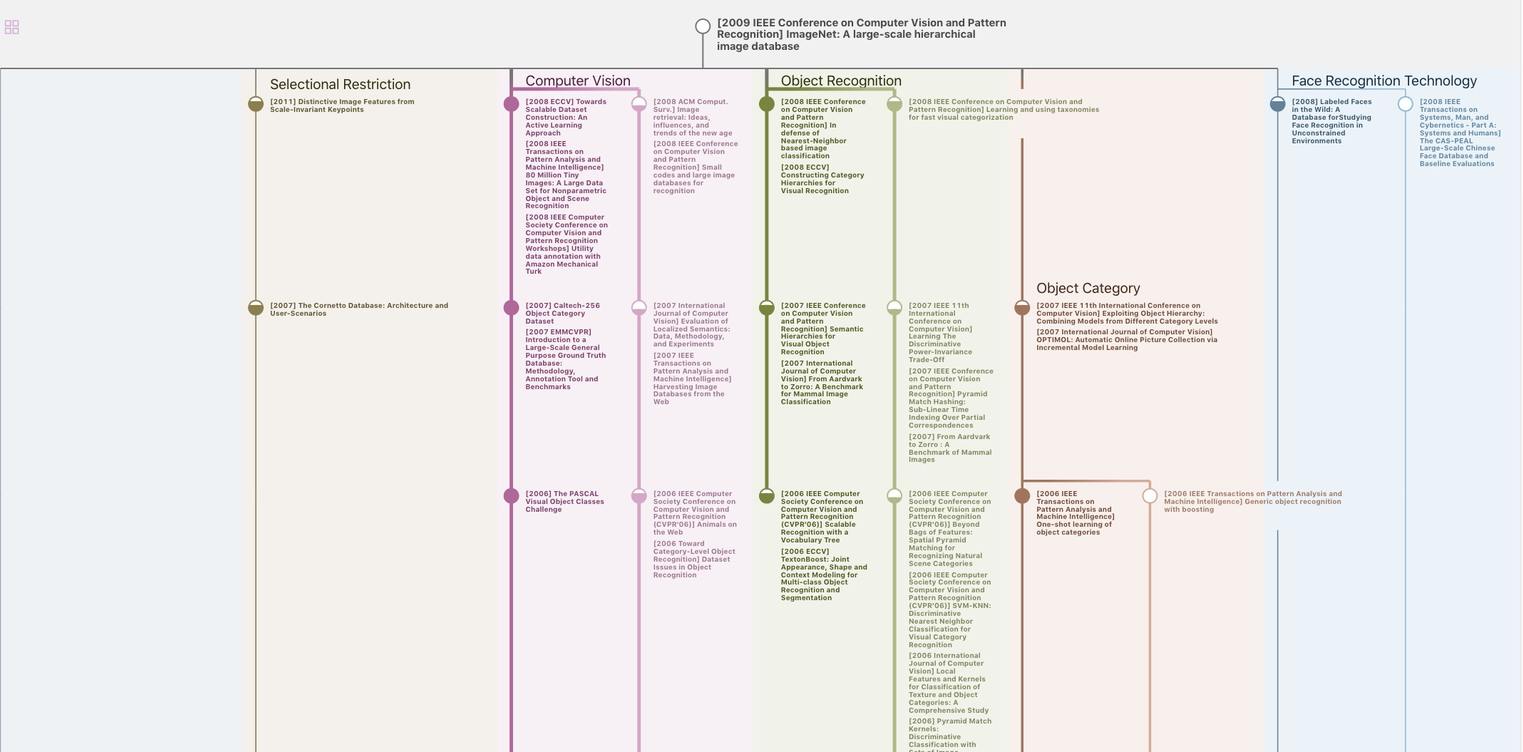
生成溯源树,研究论文发展脉络
Chat Paper
正在生成论文摘要