Machine Learning Approaches: Detecting the Disease Variants in Human-Exhaled Breath Biomarkers
ACS Omega(2023)
摘要
In recent days, the development of sensor-based medical devices has been found to be very effective for the prediction and analysis of the onset of diseases. The instigation of an electronic nose (eNose) device is profound and very useful in diverse applications. The analysis of exhaled breath biomarkers using eNose sensors has attained wider attention among researchers, and the prediction of multiple disease variants using the same is still an open research problem. In this work, an enhanced XGBooster classifier-based prediction mechanism was introduced to identify the disease variants based on the responses of commercially available metal oxide-based Figaro (Japan) sensors including TGS826, TGS822, TGS2600, and TGS2602. The implemented model secured 98.36% prediction accuracy in multiclass disease prediction and classification. The homemade one-dimensional metal oxide sensing elements such as ZnO, Cr-doped ZnO, and ZnO/NiO were integrated with the aforementioned sensor array for the specific detection of the three biomarkers of interest. This model has attained a classification accuracy of 99.77, 94.91, and 96.56% toward ammonia, ethanol, and acetone, respectively. And the multiclass disease biomarker classification accuracy of the readymade and homemade eNose prototype models was compared, and the results are summarized.
更多查看译文
AI 理解论文
溯源树
样例
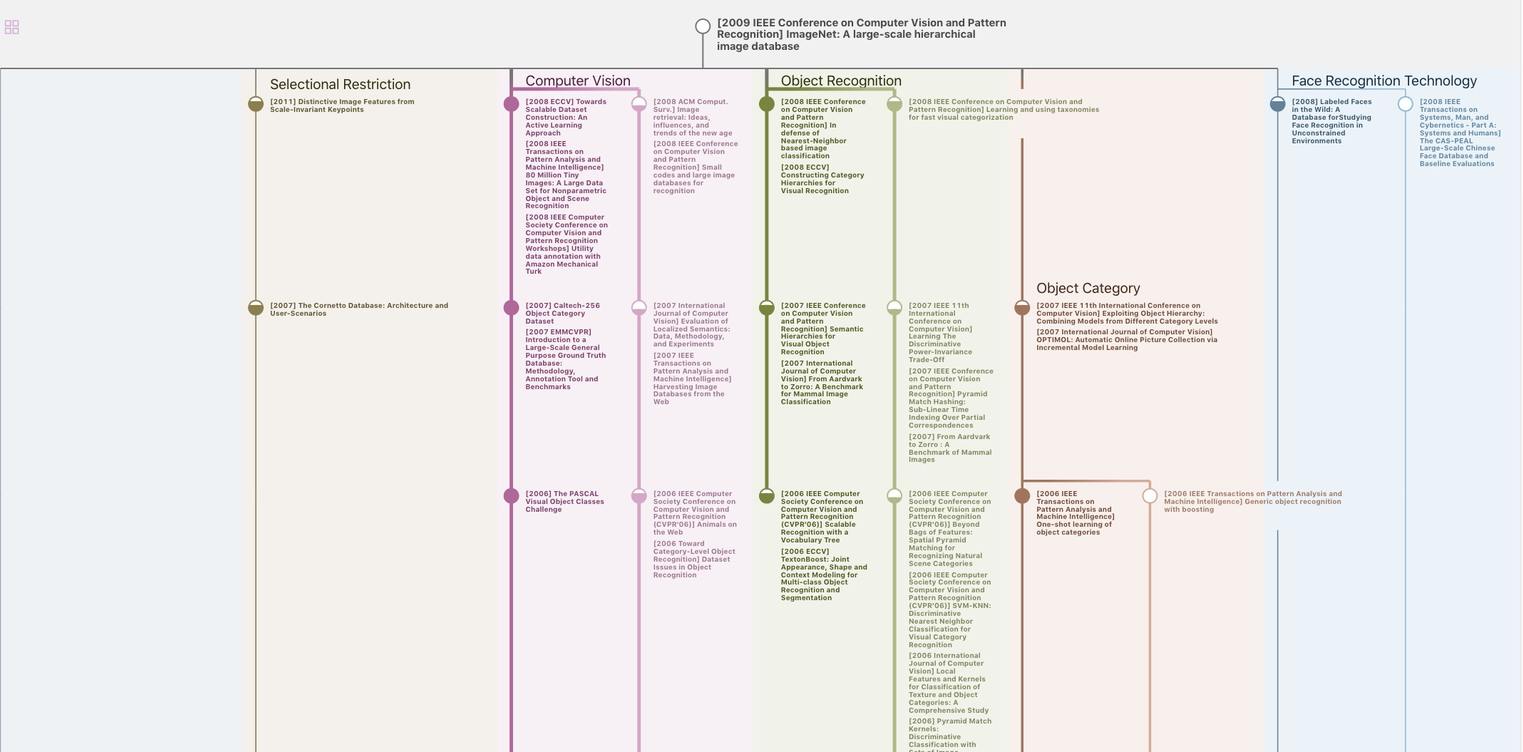
生成溯源树,研究论文发展脉络
Chat Paper
正在生成论文摘要