Tobias: A Random CNN Sees Objects
IEEE TRANSACTIONS ON PATTERN ANALYSIS AND MACHINE INTELLIGENCE(2024)
摘要
This paper starts by revealing a surprising finding: without any learning, a randomly initialized CNN can localize objects surprisingly well. That is, a CNN has an inductive bias to naturally focus on objects, named as Tobias ("The object is at sight") in this paper. This empirical inductive bias is further theoretically analyzed and empirically verified, and successfully applied to self-supervised learning as well as supervised learning. For self-supervised learning, a CNN is encouraged to learn representations that focus on the foreground object, by transforming every image into various versions with different backgrounds, where the foreground and background separation is guided by Tobias. Experimental results show that the proposed Tobias significantly improves downstream tasks, especially for object detection. This paper also shows that Tobias has consistent improvements on training sets of different sizes, and is more resilient to changes in image augmentations. Furthermore, we apply Tobias to supervised image classification by letting the average pooling layer focus on foreground regions, which achieves improved performance on various benchmarks.
更多查看译文
关键词
Convolutional neural networks,randomly initialized networks,object localization,self-supervised learning
AI 理解论文
溯源树
样例
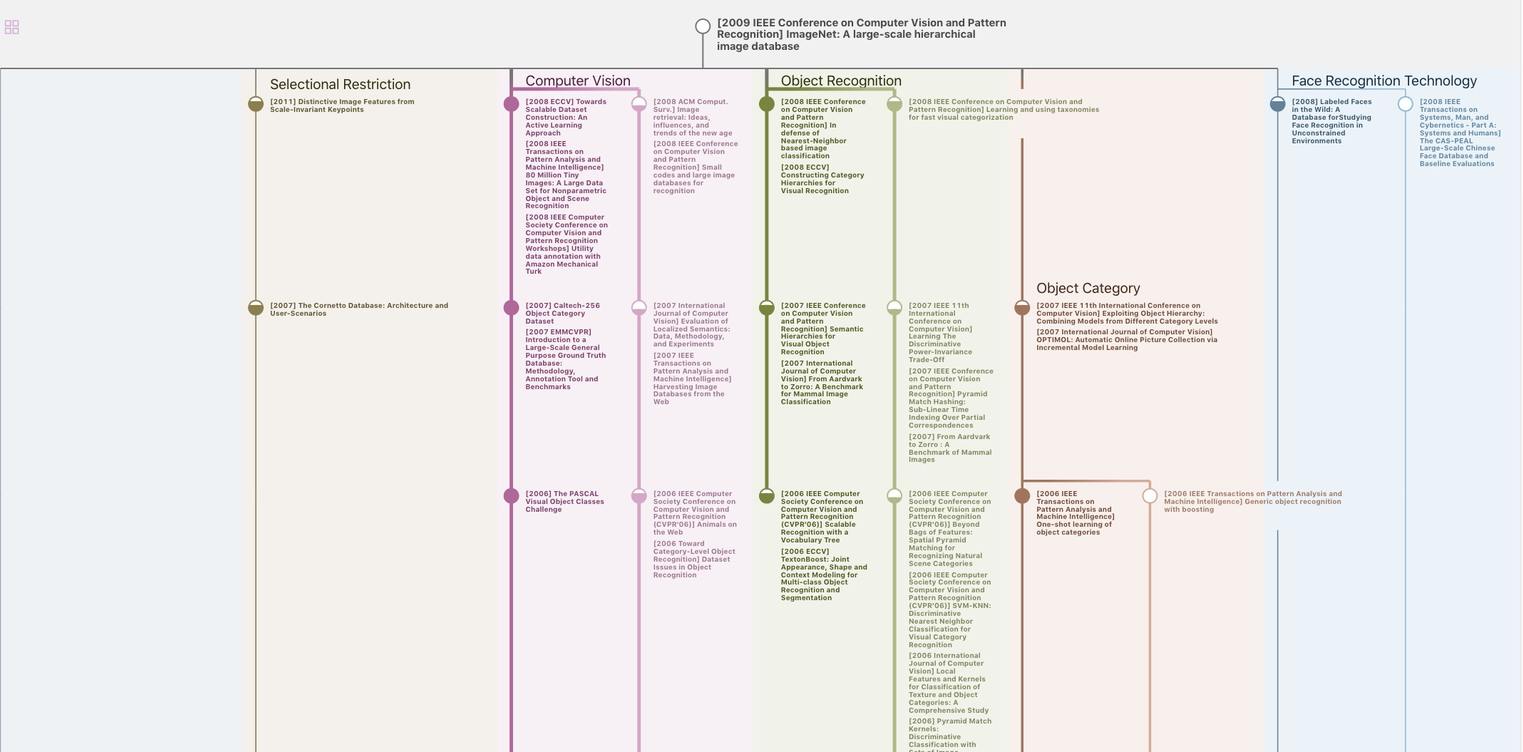
生成溯源树,研究论文发展脉络
Chat Paper
正在生成论文摘要