RudolfV: A Foundation Model by Pathologists for Pathologists
arxiv(2024)
摘要
Histopathology plays a central role in clinical medicine and biomedical
research. While artificial intelligence shows promising results on many
pathological tasks, generalization and dealing with rare diseases, where
training data is scarce, remains a challenge. Distilling knowledge from
unlabelled data into a foundation model before learning from, potentially
limited, labelled data provides a viable path to address these challenges. In
this work, we extend the state of the art of foundation models for digital
pathology whole slide images by semi-automated data curation and incorporating
pathologist domain knowledge. Specifically, we combine computational and
pathologist domain knowledge (1) to curate a diverse dataset of 133k slides
corresponding to 1.2 billion image patches covering data from different
fixation, staining, and scanning protocols as well as data from different
indications and labs across the EU and US, (2) for grouping semantically
similar slides and tissue patches, and (3) to augment the input images during
training. We evaluate the resulting model on a set of public and internal
benchmarks and show that although our foundation model is trained with an order
of magnitude less slides, it performs on par or better than competing models.
We expect that scaling our approach to more data and larger models will further
increase its performance and capacity to deal with increasingly complex real
world tasks in diagnostics and biomedical research.
更多查看译文
AI 理解论文
溯源树
样例
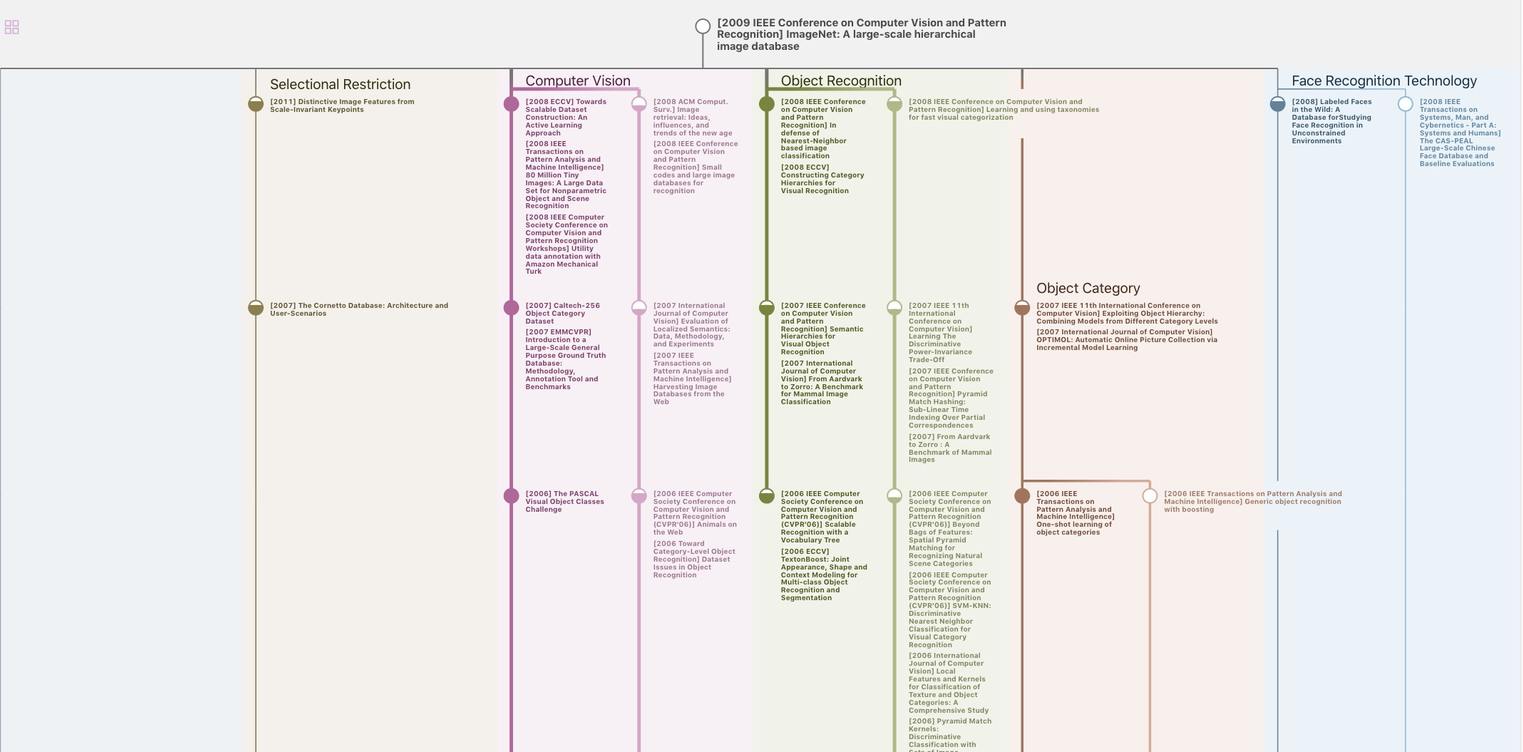
生成溯源树,研究论文发展脉络
Chat Paper
正在生成论文摘要