Towards explainability for AI-based edge wireless signal automatic modulation classification
Journal of Cloud Computing(2024)
摘要
With the development of artificial intelligence technology and edge computing technology, deep learning-based automatic modulation classification (AI-based AMC) deployed at edge devices using centralised or distributed learning methods for optimisation has emerged in recent years, and has made great progress in the recognition accuracy and recognisable range of wireless signals. However, the lack of sufficient explanation of these models leads to low accuracy and training efficiency of model training, and their applications and further improvements are limited. Researchers have started to propose interpretable methods for technical analysis of deep learning-based AMC. In this paper, based on the research and application development of interpretable methods in recent years, we review the applicable methods and existing research challenges of interpretable automatic modulation classification. And an interpretable AI-based automatic modulation classification framework is proposed to map the interpretability of automatic modulation classification results by obtaining the contribution of wireless signal features to deep learning network training. Experimental results show that the proposed method possesses the ability to explore the classification mechanism of non-transparent auto-modulated classification networks and has the potential to help edge devices train networks with lower energy consumption and higher accuracy.
更多查看译文
关键词
Automatic modulation classification,Explainable methods,Deep learning
AI 理解论文
溯源树
样例
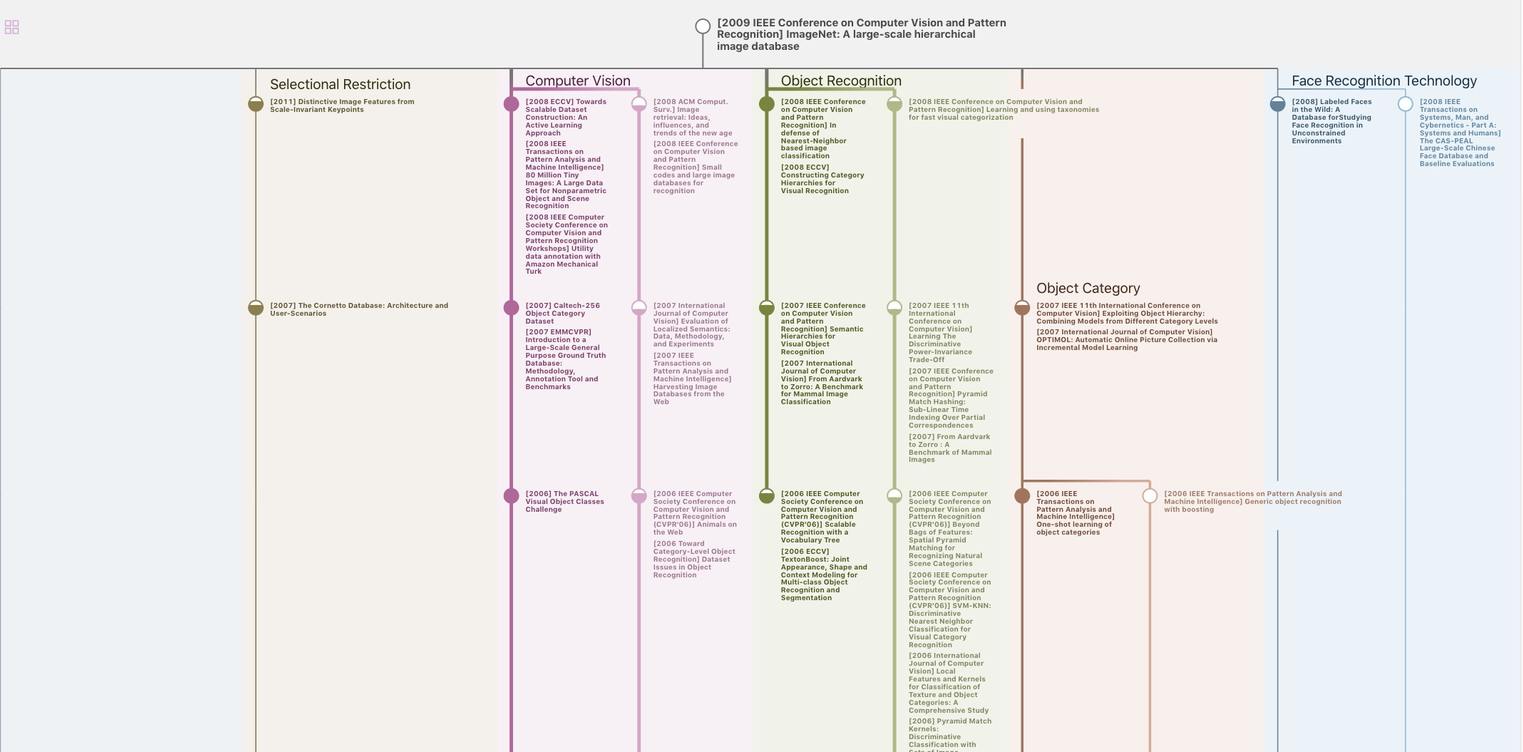
生成溯源树,研究论文发展脉络
Chat Paper
正在生成论文摘要