Artificial neural networks coupled with numerical approach for the stability prediction of non-uniform functionally graded microscale cylindrical structures
STRUCTURES(2024)
摘要
Functionally graded materials (FGMs) have garnered significant attention in research due to their potential for creating innovative materials with diverse applications. This study focuses on investigating the behavior of steelconcrete functionally graded structures under axial loading, specifically exploring nonlinear buckling. The research considers cylindrical structures with simply-supported boundary conditions and analyzes four different configurations with varying thickness. The governing equations are derived using the modified couple stress theory, incorporating assumptions of nonlinear von-K ' arm ' an theory coupled with classical beam theory. Numerical solutions are obtained using the general differential quadrature (GDQ) method. A notable aspect of this work is the use of Artificial Neural Networks (ANN) to predict nonlinear buckling forces, greatly enhancing the accuracy and efficiency of the predictions. This approach offers a novel method for analyzing concrete structures. The results are validated by comparing them with data from other published works, using dimensionless parameters for consistent comparisons. The findings provide a comprehensive analysis of various parameters, including wall thickness function and porosity, on the buckling stability of the cylindrical structure. Both numerical and ANN approaches are employed to report the effects. The study concludes that the uniform thickness cylinder exhibits the highest resistance to axial loading, resulting in buckling.
更多查看译文
关键词
Concrete materials,Steel-concrete structures,Nonlinear buckling,Artificial Neural Network (ANN),Functionally graded materials
AI 理解论文
溯源树
样例
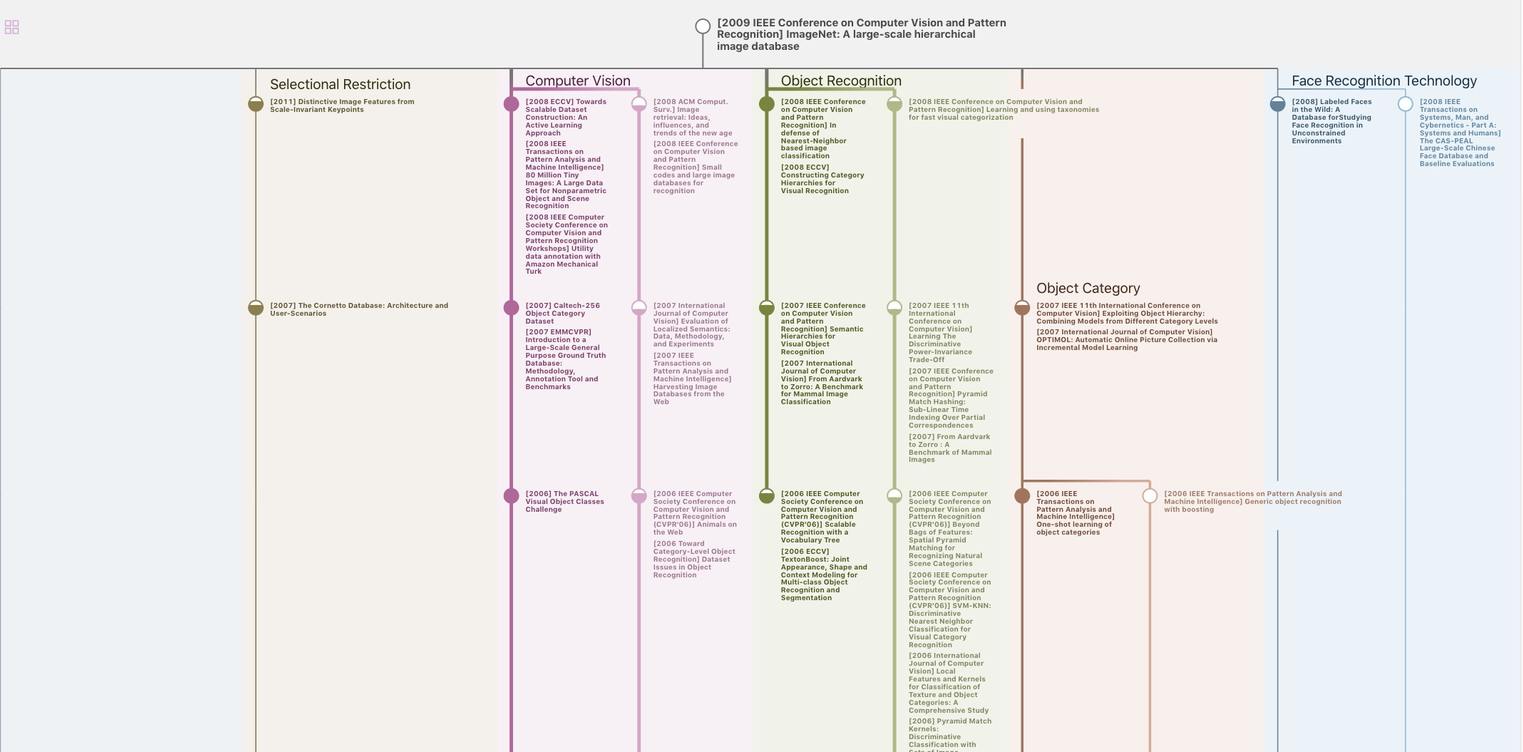
生成溯源树,研究论文发展脉络
Chat Paper
正在生成论文摘要