TrustAffinity: accurate, reliable and scalable out-of-distribution protein-ligand binding affinity prediction using trustworthy deep learning
biorxiv(2024)
摘要
Accurate, reliable and scalable predictions of protein-ligand binding affinity have a great potential to accelerate drug discovery. Despite considerable efforts, three challenges remain: out-of-distribution (OOD) generalizations for understudied proteins or compounds from unlabeled protein families or chemical scaffolds, uncertainty quantification of individual predictions, and scalability to billions of compounds. We propose a sequence-based deep learning framework, TrustAffinity, to address aforementioned challenges. TrustAffinity synthesizes a structure-informed protein language model, efficient uncertainty quantification based on residue-estimation and novel uncertainty regularized optimization. We extensively validate TrustAffinity in multiple OOD settings. TrustAffinity significantly outperforms state-of-the-art computational methods by a large margin. It achieves a Pearson's correlation between predicted and actual binding affinities above 0.9 with a high confidence and at least three orders of magnitude of faster than protein-ligand docking, highlighting its potential in real-world drug discovery. We further demonstrate TrustAffinity's practicality through an Opioid Use Disorder lead discovery case study.
### Competing Interest Statement
The authors have declared no competing interest.
更多查看译文
AI 理解论文
溯源树
样例
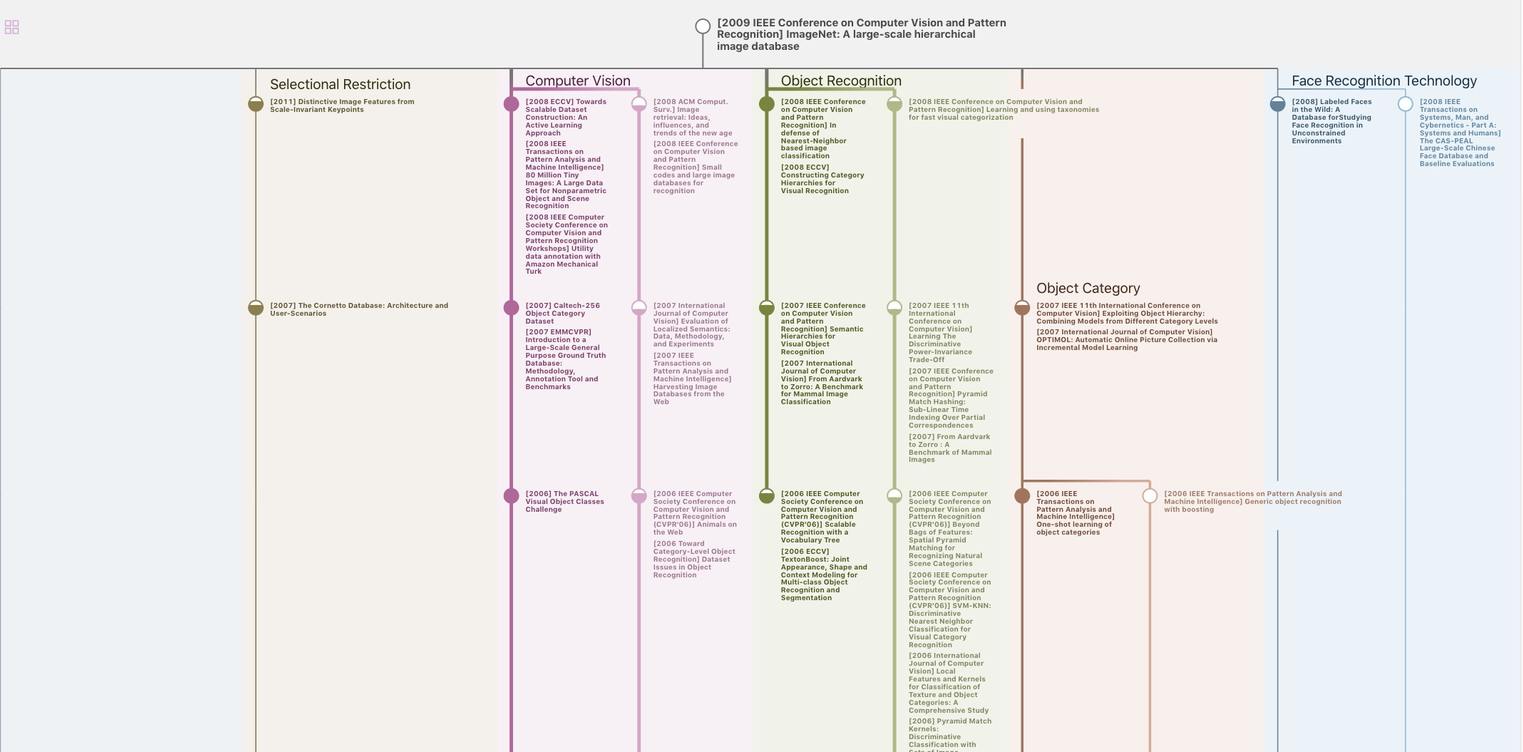
生成溯源树,研究论文发展脉络
Chat Paper
正在生成论文摘要