Multiple machine learning modeling on near mid-air collisions: An approach towards probabilistic reasoning
RELIABILITY ENGINEERING & SYSTEM SAFETY(2024)
摘要
This work presents a mathematical model to predict and explain Near Mid-Air Collisions (NMACs) based on the NASA Aviation Safety Reporting System (ASRS) database. The ASRS database contains more than 200,000 aviation incidents, which are used to learn how the combination of risk influencing factors (RIFs), such as crew size and component fatigue, affects the safety of airspace operations. Bayesian Networks (BNs) combine theory of probabilities with theory of graphs and are considered one of the most effective theoretical models in the fields of knowledge representation and reasoning with uncertainty. The resulting model allows to calculate the posterior probabilities of some targeted outputs, therefore providing a mathematically consistent framework to quantify and to compute with uncertainty the likelihood of incident occurrence over time when some factors are known. Furthermore, the bidirectional reasoning technology of BNs can calculate the posterior probabilities of its var- iables under the system incident condition, and find out the most likely combination that caused a NMAC. Finally, the resulting probabilistic models are compared with sixteen Machine Learning Algorithms, and ad- vantageous properties were critically evaluated, such as a white-box reasoning and probability as a measure of certainty about the state of unobserved variables.
更多查看译文
关键词
Aviation safety,Bayesian Network,Probabilistic knowledge-based system,Machine learning
AI 理解论文
溯源树
样例
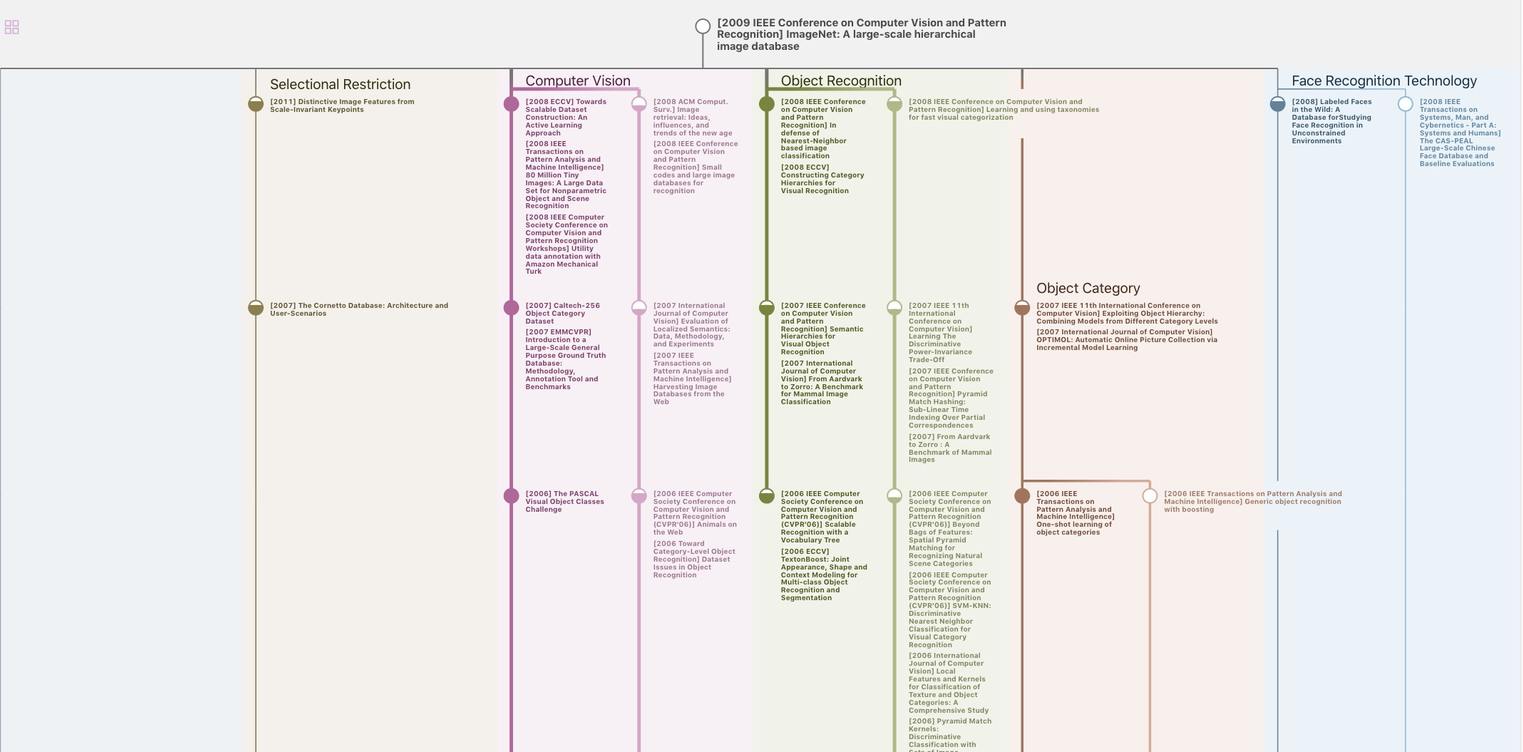
生成溯源树,研究论文发展脉络
Chat Paper
正在生成论文摘要