Causality and tractable probabilistic models
FRONTIERS IN COMPUTER SCIENCE(2024)
摘要
Causal assertions stem from an asymmetric relation between some variable's causes and effects, i.e., they imply the existence of a function decomposition of a model where the effects are a function of the causes without implying that the causes are functions of the effects. In structural causal models, information is encoded in the compositions of functions that define variables because that information is used to constraint how an intervention that changes the definition of a variable influences the rest of the variables. Current probabilistic models with tractable marginalization also imply a function decomposition but with the purpose of allowing easy marginalization of variables. In this article, structural causal models are extended so that the information implicitly stored in their structure is made explicit in an input-output mapping in higher dimensional representation where we get to define the cause-effect relationships as constraints over a function space. Using the cause-effect relationships as constraints over a space of functions, the existing methodologies for handling causality with tractable probabilistic models are unified under a single framework and generalized.
更多查看译文
关键词
causality,tractable probabilistic models,structural causal models,function decompositions,probabilistic models
AI 理解论文
溯源树
样例
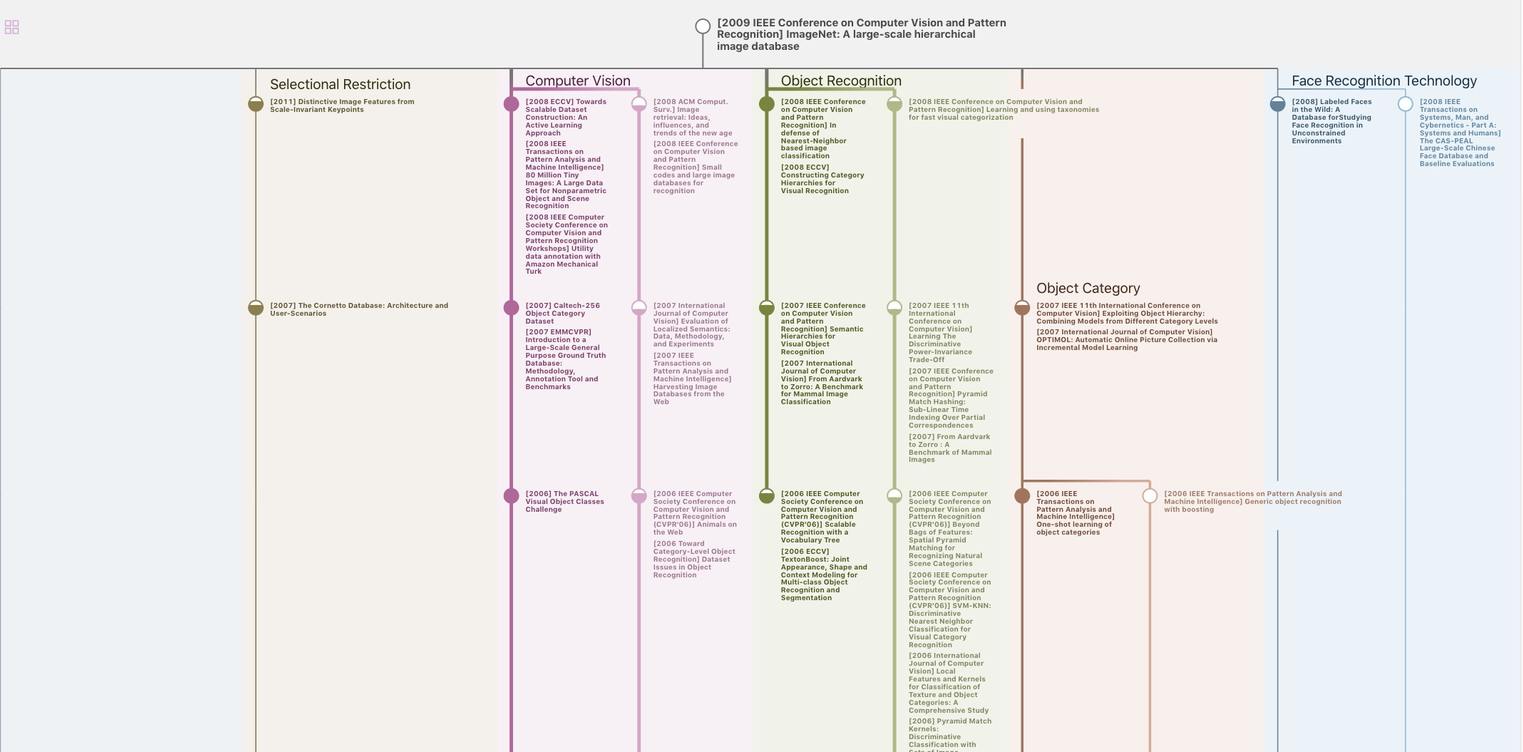
生成溯源树,研究论文发展脉络
Chat Paper
正在生成论文摘要