Deep Learning Models for Diagnosis of Schizophrenia Using EEG Signals: Emerging Trends, Challenges, and Prospects
Archives of Computational Methods in Engineering(2024)
摘要
Schizophrenia (ScZ) is a chronic neuropsychiatric disorder characterized by disruptions in cognitive, perceptual, social, emotional, and behavioral functions. In the traditional approach, the diagnosis of ScZ primarily relies on the subject’s response and the psychiatrist’s experience, making it highly subjective, prejudiced, and time-consuming. In recent medical research, incorporating deep learning (DL) into the diagnostic process improves performance by reducing inter-observer variation and providing qualitative and quantitative support for clinical decisions. Compared with other modalities, such as magnetic resonance images (MRI) or computed tomography (CT) scans, electroencephalogram (EEG) signals give better insights into the underlying neural mechanisms and brain biomarkers of ScZ. Deep learning models show promising results but the utilization of EEG signals as an effective biomarker for ScZ is still under research. Numerous deep learning models have recently been developed for automated ScZ diagnosis with EEG signals exclusively, yet a comprehensive assessment of these approaches still does not exist in the literature. To fill this gap, we comprehensively review the current advancements in deep learning-based schizophrenia diagnosis using EEG signals. This review is intended to provide systematic details of prominent components: deep learning models, ScZ EEG datasets, data preprocessing approaches, input data formulations for DL, chronological DL methodology advancement in ScZ diagnosis, and design trends of DL architecture. Finally, few challenges in both clinical and technical aspects that create hindrances in achieving the full potential of DL models in EEG-based ScZ diagnosis are expounded along with future outlooks.
更多查看译文
AI 理解论文
溯源树
样例
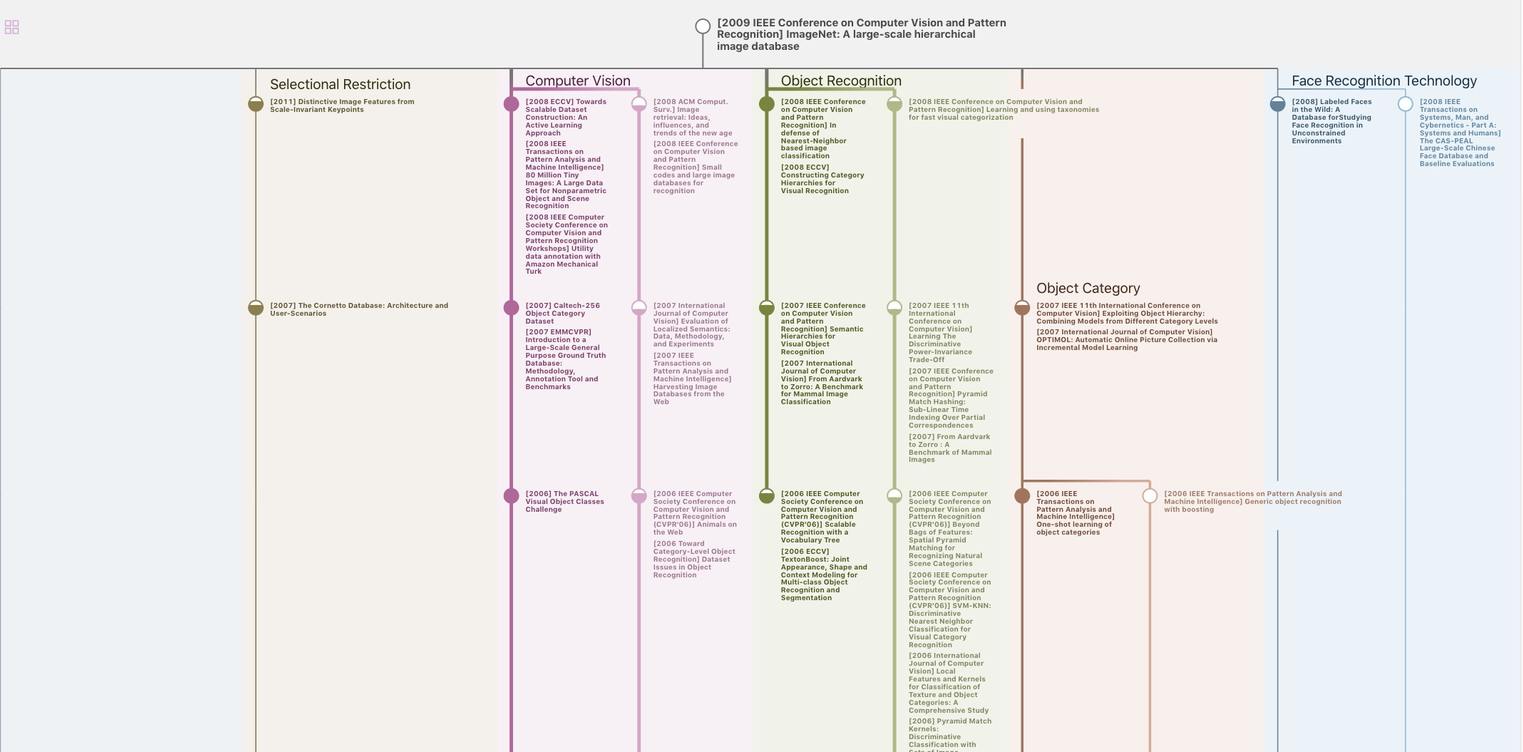
生成溯源树,研究论文发展脉络
Chat Paper
正在生成论文摘要