SACAT: Student-Adaptive Computerized Adaptive Testing
2023 5TH INTERNATIONAL CONFERENCE ON DISTRIBUTED ARTIFICIAL INTELLIGENCE, DAI 2023(2023)
摘要
Computerized Adaptive Testing refers to an online student ability testing system. It consists of Selection Algorithm and Cognitive Diagnosis Module (CDM). The Selection Algorithm aims to carefully select a small set of questions for the newly arrived student to answer, and the CDM aims to infer the ability of the newly arrived student based on his answers to the selected questions. The CDM faces the challenge of inferring student ability based on the short question-answer sequence. The state-of-the-art approach employs a meta-learning framework to solve this challenge. Specifically, in the outer-layer of the meta-learning framework, it learns an average student ability from all students as the initial ability for the newly arrived student, while in the inner-layer of the meta-learning framework, it infers the newly arrived student's ability from the short sequence. However, this approach suffers from two major limitations: Firstly, collaborative information between the newly arrived student and all students was not utilized. Secondly, the meta-learning framework has a slow convergence. This paper introduces Student-Adaptive Computerized Adaptive Testing (SACAT), which addresses these issues by: Firstly, use the short sequence of the newly arrived student to retrieve similar students from all students. Secondly, design a fast adaptive function that can adaptively generate an ability representation suitable for the newly arrived student from abilities of similar students. Through extensive experiments on three real-world student response datasets, we validate that SACAT can outperform existing CAT methods. Furthermore, through the fast adaptation, we show that SACAT achieves fast model convergence.
更多查看译文
关键词
few-shot learning,fast adaption,Computerized Adaptive Testing
AI 理解论文
溯源树
样例
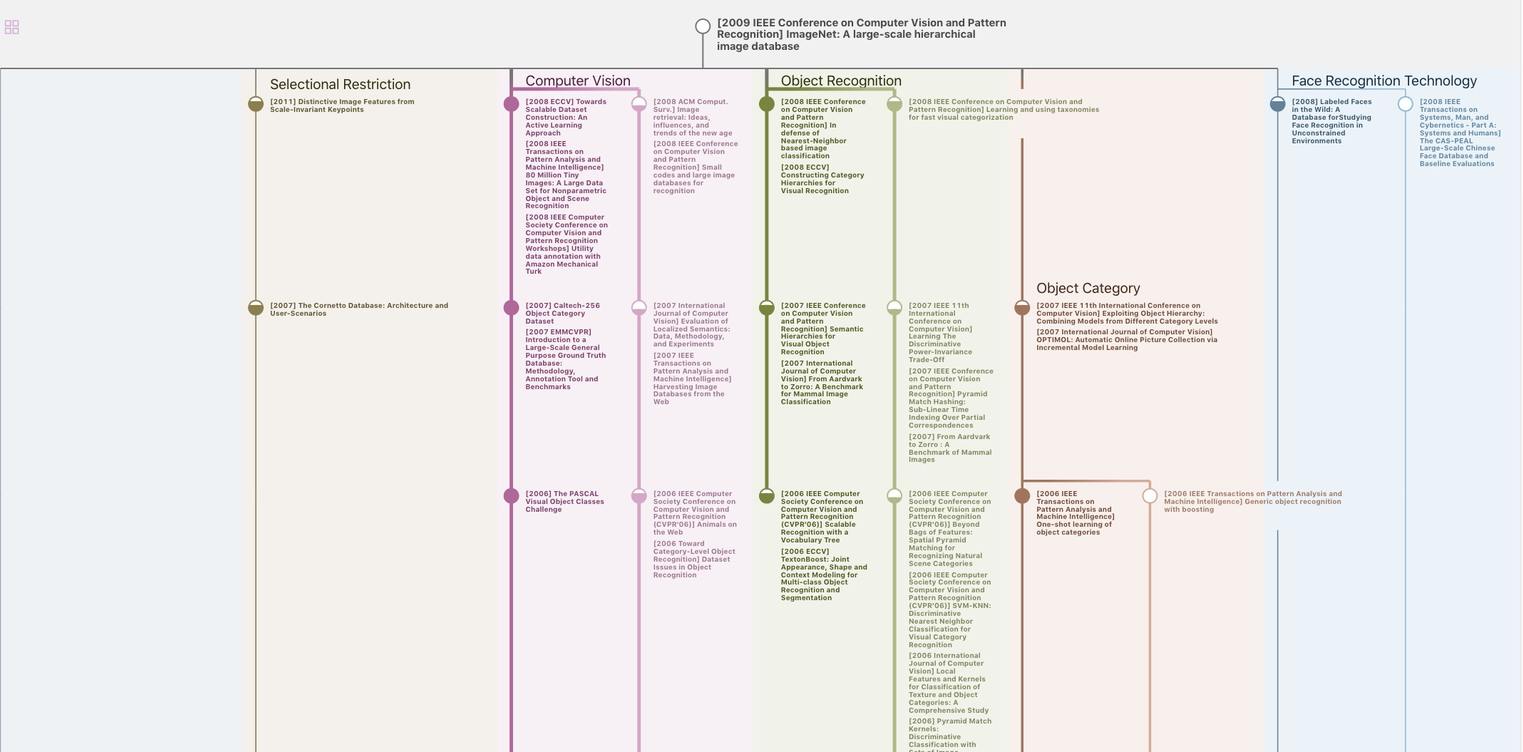
生成溯源树,研究论文发展脉络
Chat Paper
正在生成论文摘要