VeNoM: Approximate Subgraph Matching with Enhanced Neighbourhood Structural Information
PROCEEDINGS OF 7TH JOINT INTERNATIONAL CONFERENCE ON DATA SCIENCE AND MANAGEMENT OF DATA, CODS-COMAD 2024(2024)
摘要
Subgraph matching is an important research problem in the area of graph mining. Over the years, researchers have made significant headway in this direction with many state-of-the-art algorithms aimed at providing an efficient solution for approximate subgraph matching (ASM). Although many studies have been conducted to compare these and highlight their respective advantages and differences, little analysis has been done on how varying the different aspects of an ASM approach including the depth and breadth of neighborhood affect the performance. In this paper, we propose VeNoM, a variant of a state-of-the-art ASM algorithm VELSET, and present different extensions of it by parameterizing the breadth and depth of the neighborhood considered. We discuss the effects of these neighborhood parameters and other graph parameters on performance through empirical results over diverse datasets. We also compare the VeNoM instances against VerSaChI, a two-hop neighborhood similarity based ASM approach. The empirical results suggest that increasing the depth of a neighborhood can increase the accuracy of ASM significantly, although it requires a much longer running time. The breadth of the neighborhood for a vertex does not matter much as long as it is more than a single edge.
更多查看译文
关键词
Subgraph Similarity,Approximate Matching,Statistical Significance,Chi-Square,Labeled Graph
AI 理解论文
溯源树
样例
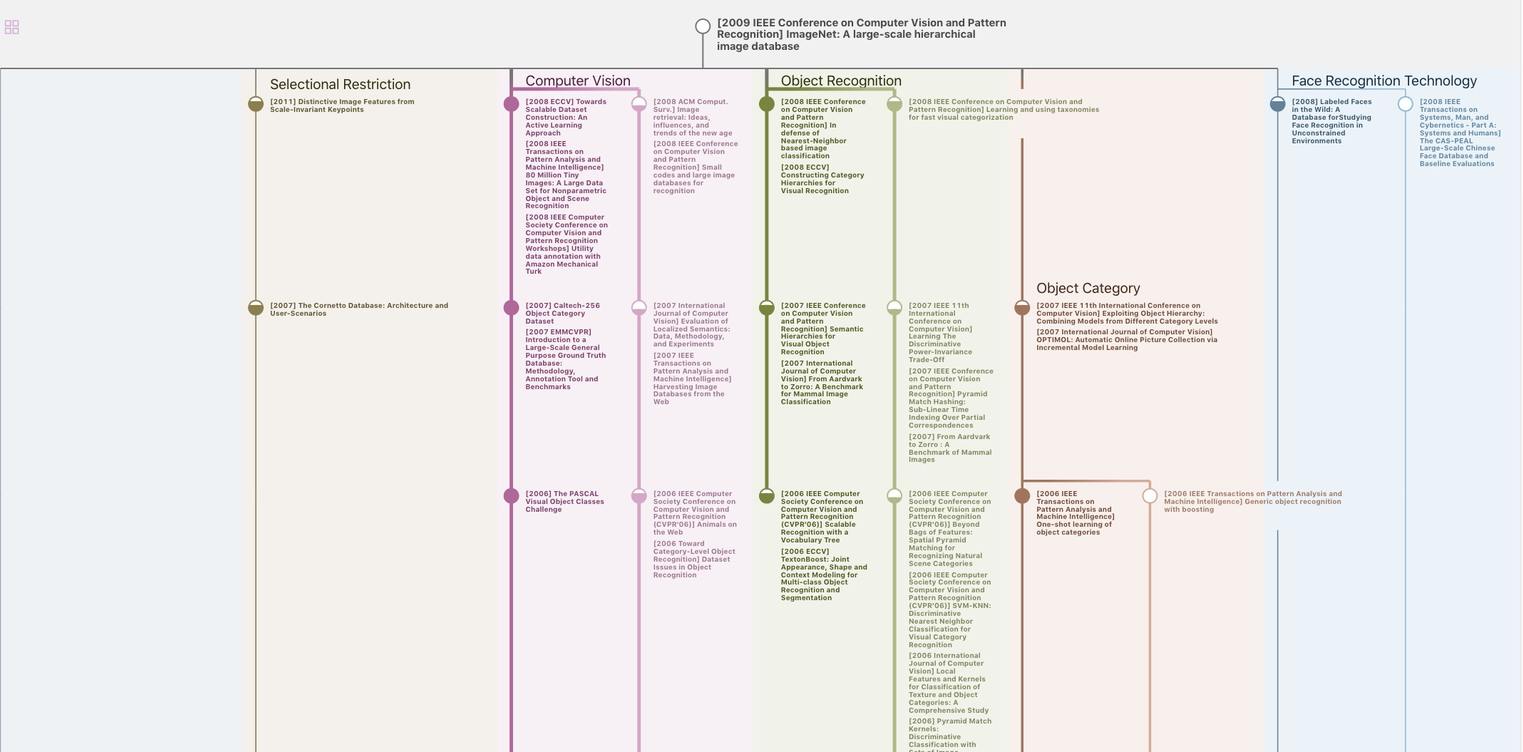
生成溯源树,研究论文发展脉络
Chat Paper
正在生成论文摘要