A Closer look at Consistency Regularization for Semi-Supervised Learning
PROCEEDINGS OF 7TH JOINT INTERNATIONAL CONFERENCE ON DATA SCIENCE AND MANAGEMENT OF DATA, CODS-COMAD 2024(2024)
摘要
Several state-of-the-art deep learning models have utilized consistency regularization by augmenting data during training. In addition to contributing to the generalizability of a model, data augmentation techniques have also been used in semi-supervised learning where a trained network is used to pseudolabel unlabelled data. During this process a supervised model is utilized to assign pseudolabels which are generated from augmented variations of the unlabelled data. This allows the model to look at different prediction vectors over such augmented versions each unlabelled data sample. However, some of these augmentations are stronger than others depending on the challenges they pose for a supervised model which has been trained on very limited data. We present a thorough study of data augmentation techniques and show that like previous semi-supervised methods only using the mean response of the model on augmentations may not be the best idea for pseudolabelling in the context of such a weakly-supervised paradigm of learning. In particular, for this work, we study consistency regularization from the perspective of pseudolabelling data for a self-training based student-teacher learning framework.
更多查看译文
AI 理解论文
溯源树
样例
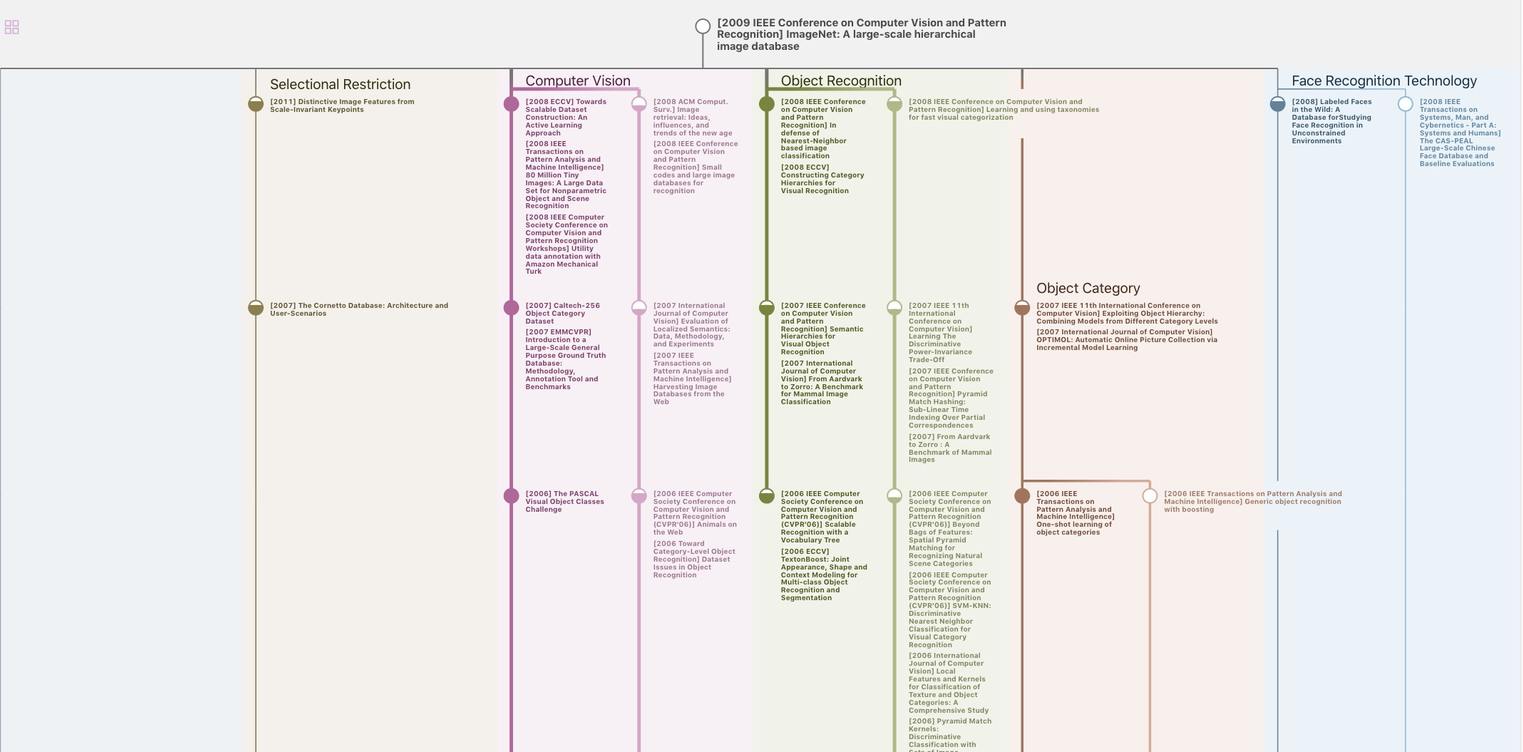
生成溯源树,研究论文发展脉络
Chat Paper
正在生成论文摘要