Beyond Fidelity: Explaining Vulnerability Localization of Learning-based Detectors
CoRR(2024)
摘要
Vulnerability detectors based on deep learning (DL) models have proven their
effectiveness in recent years. However, the shroud of opacity surrounding the
decision-making process of these detectors makes it difficult for security
analysts to comprehend. To address this, various explanation approaches have
been proposed to explain the predictions by highlighting important features,
which have been demonstrated effective in other domains such as computer vision
and natural language processing. Unfortunately, an in-depth evaluation of
vulnerability-critical features, such as fine-grained vulnerability-related
code lines, learned and understood by these explanation approaches remains
lacking. In this study, we first evaluate the performance of ten explanation
approaches for vulnerability detectors based on graph and sequence
representations, measured by two quantitative metrics including fidelity and
vulnerability line coverage rate. Our results show that fidelity alone is not
sufficient for evaluating these approaches, as fidelity incurs significant
fluctuations across different datasets and detectors. We subsequently check the
precision of the vulnerability-related code lines reported by the explanation
approaches, and find poor accuracy in this task among all of them. This can be
attributed to the inefficiency of explainers in selecting important features
and the presence of irrelevant artifacts learned by DL-based detectors.
更多查看译文
关键词
Vulnerability Detection,Explanation Approaches,Fidelity,Coverage Rate
AI 理解论文
溯源树
样例
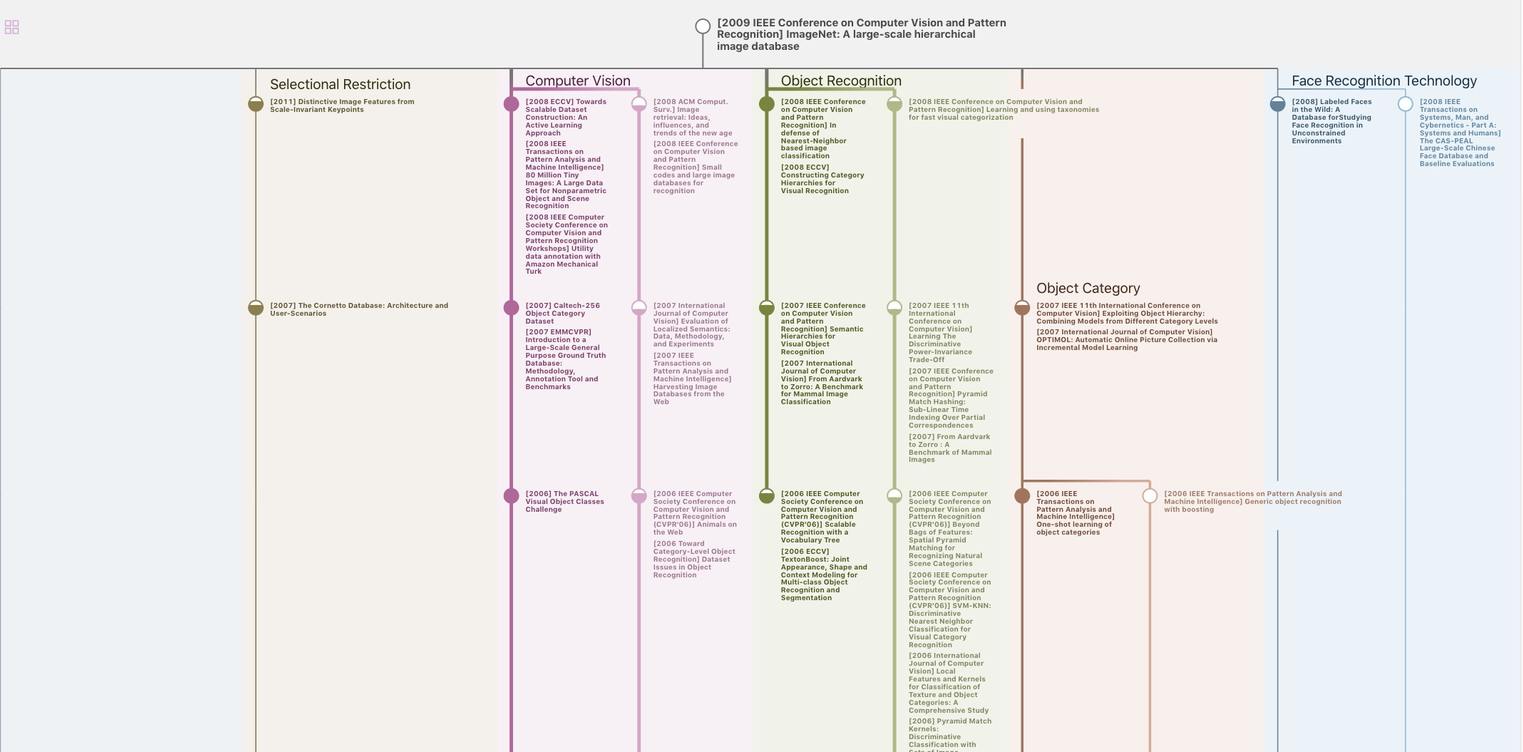
生成溯源树,研究论文发展脉络
Chat Paper
正在生成论文摘要