Assessing the robustness of a machine-learning model for early detection of pancreatic adenocarcinoma (PDA): evaluating resilience to variations in image acquisition and radiomics workflow using image perturbation methods
Abdominal Radiology(2024)
摘要
Purpose To evaluate robustness of a radiomics-based support vector machine (SVM) model for detection of visually occult PDA on pre-diagnostic CTs by simulating common variations in image acquisition and radiomics workflow using image perturbation methods. Methods Eighteen algorithmically generated-perturbations, which simulated variations in image noise levels ( σ , 2 σ , 3 σ , 5 σ ), image rotation [both CT image and the corresponding pancreas segmentation mask by 45° and 90° in axial plane], voxel resampling (isotropic and anisotropic), gray-level discretization [bin width (BW) 32 and 64)], and pancreas segmentation (sequential erosions by 3, 4, 6, and 8 pixels and dilations by 3, 4, and 6 pixels from the boundary), were introduced to the original (unperturbed) test subset ( n = 128; 45 pre-diagnostic CTs, 83 control CTs with normal pancreas). Radiomic features were extracted from pancreas masks of these additional test subsets, and the model's performance was compared vis-a-vis the unperturbed test subset. Results The model correctly classified 43 out of 45 pre-diagnostic CTs and 75 out of 83 control CTs in the unperturbed test subset, achieving 92.2% accuracy and 0.98 AUC. Model's performance was unaffected by a three-fold increase in noise level except for sensitivity declining to 80% at 3 σ ( p = 0.02). Performance remained comparable vis-a-vis the unperturbed test subset despite variations in image rotation ( p = 0.99), voxel resampling ( p = 0.25–0.31), change in gray-level BW to 32 ( p = 0.31–0.99), and erosions/dilations up to 4 pixels from the pancreas boundary ( p = 0.12–0.34). Conclusion The model’s high performance for detection of visually occult PDA was robust within a broad range of clinically relevant variations in image acquisition and radiomics workflow.
更多查看译文
关键词
Pancreas,Machine-learning,Radiomics,Pancreatic ductal carcinoma,X-ray computed tomography
AI 理解论文
溯源树
样例
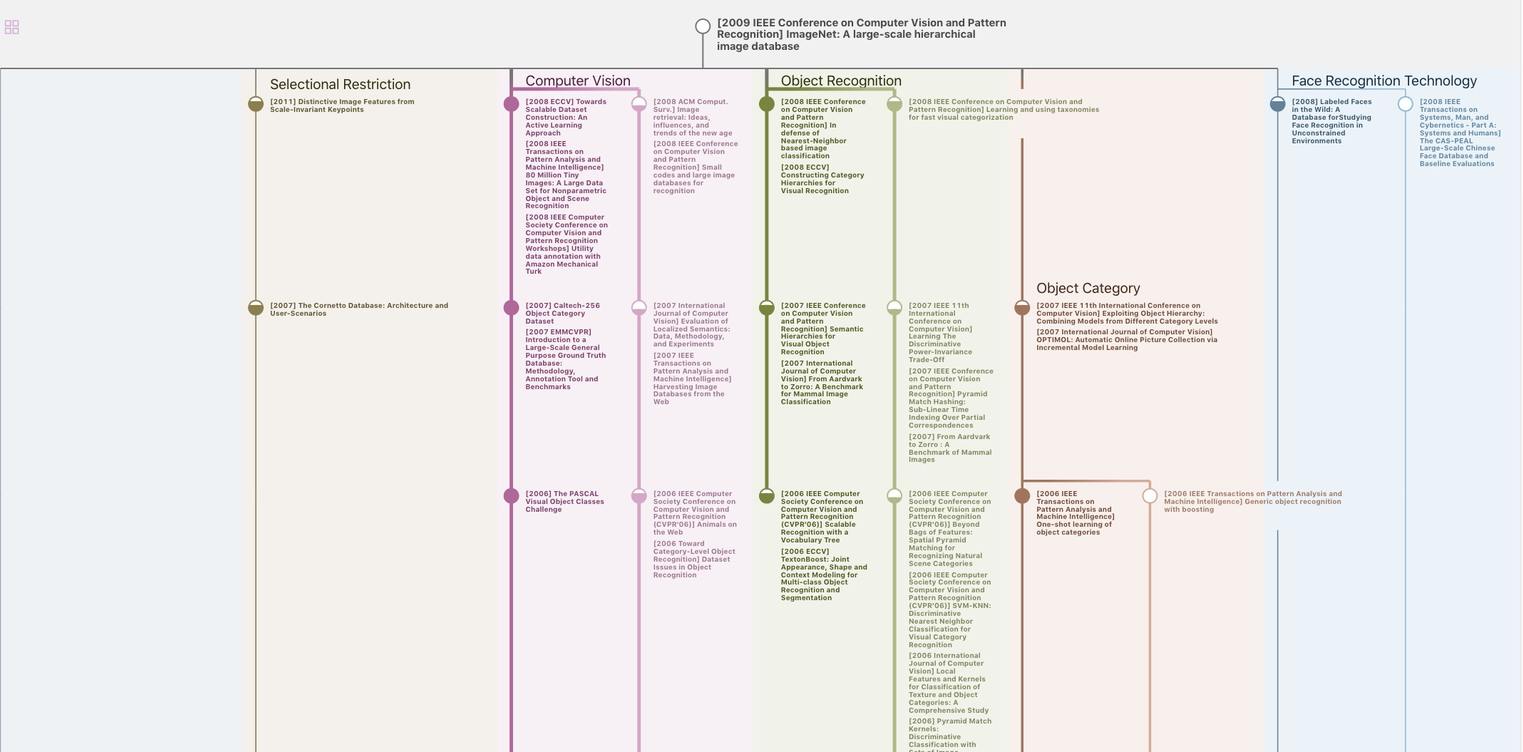
生成溯源树,研究论文发展脉络
Chat Paper
正在生成论文摘要