On the parameter combinations that matter and on those that do not: data-driven studies of parameter (non)identifiability.
PNAS nexus(2022)
摘要
We present a data-driven approach to characterizing nonidentifiability of a model's parameters and illustrate it through dynamic as well as steady kinetic models. By employing Diffusion Maps and their extensions, we discover the minimal combinations of parameters required to characterize the output behavior of a chemical system: a set of effective parameters for the model. Furthermore, we introduce and use a Conformal Autoencoder Neural Network technique, as well as a kernel-based Jointly Smooth Function technique, to disentangle the redundant parameter combinations that do not affect the output behavior from the ones that do. We discuss the interpretability of our data-driven effective parameters, and demonstrate the utility of the approach both for behavior prediction and parameter estimation. In the latter task, it becomes important to describe level sets in parameter space that are consistent with a particular output behavior. We validate our approach on a model of multisite phosphorylation, where a reduced set of effective parameters (nonlinear combinations of the physical ones) has previously been established analytically.
更多查看译文
关键词
parameter combinations,data-driven
AI 理解论文
溯源树
样例
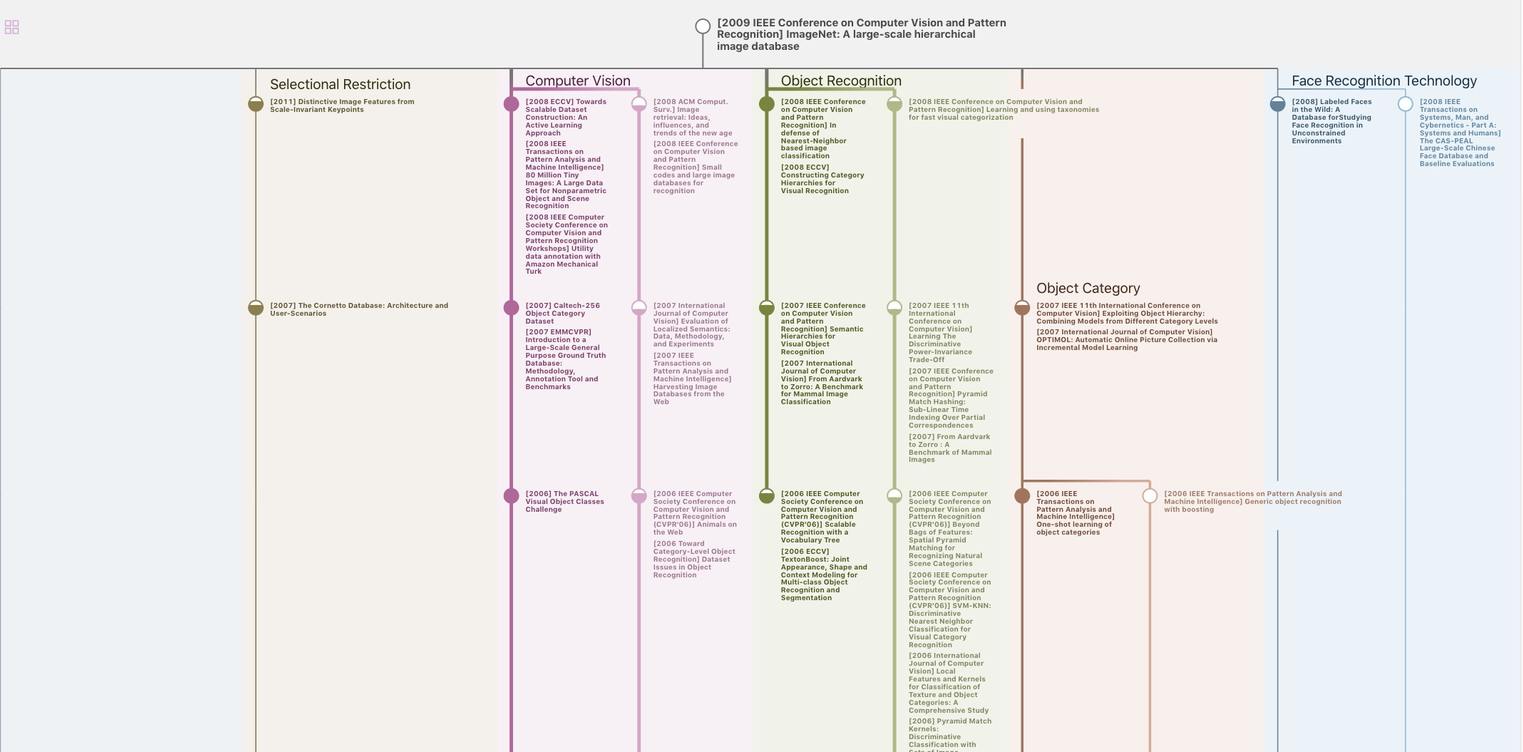
生成溯源树,研究论文发展脉络
Chat Paper
正在生成论文摘要