Shallow hybrid quantum-classical convolutional neural network model for image classification
Quantum Information Processing(2024)
摘要
Currently, quantum neural networks (QNNs) have achieved some success in image classification due to their strong computational capabilities. However, as the number of layers in QNNs increases, the training process becomes complex and the accuracy tends to be low, resulting in poor network stability. To address these challenges, this paper proposes a shallow hybrid quantum-classical convolutional neural network (SHQCNN) model. Specifically, the SHQCNN model is built on an enhanced variational quantum method that is effective for image classification. In the input layer, the model uses a kernel coding method to make data easier to distinguish. In the hidden layer, a variational quantum circuit is designed to reduce the depth and complexity of the network. The output layer uses a mini-batch gradient descent algorithm for parameter training, which improves learning speed by performing weight updates more frequently. The SHQCNN model can achieve high stability and accuracy in image classification, which effectively solves the issue of gradient disappearance caused by too many layers in the network. Its feasibility and practicality are verified by comparing it with the classical neural network (CNN) model. Its generalization and stability as well as its noise immunity are verified on various datasets with different amounts of noise. The experimental results show the superior performance of the proposed SHQCNN model. For example, compared with CNN, its accuracy rate is improved by 6.66%, reaching 99.58%.
更多查看译文
关键词
Variational quantum,Shallow neural network,Quantum convolutional,Image classification
AI 理解论文
溯源树
样例
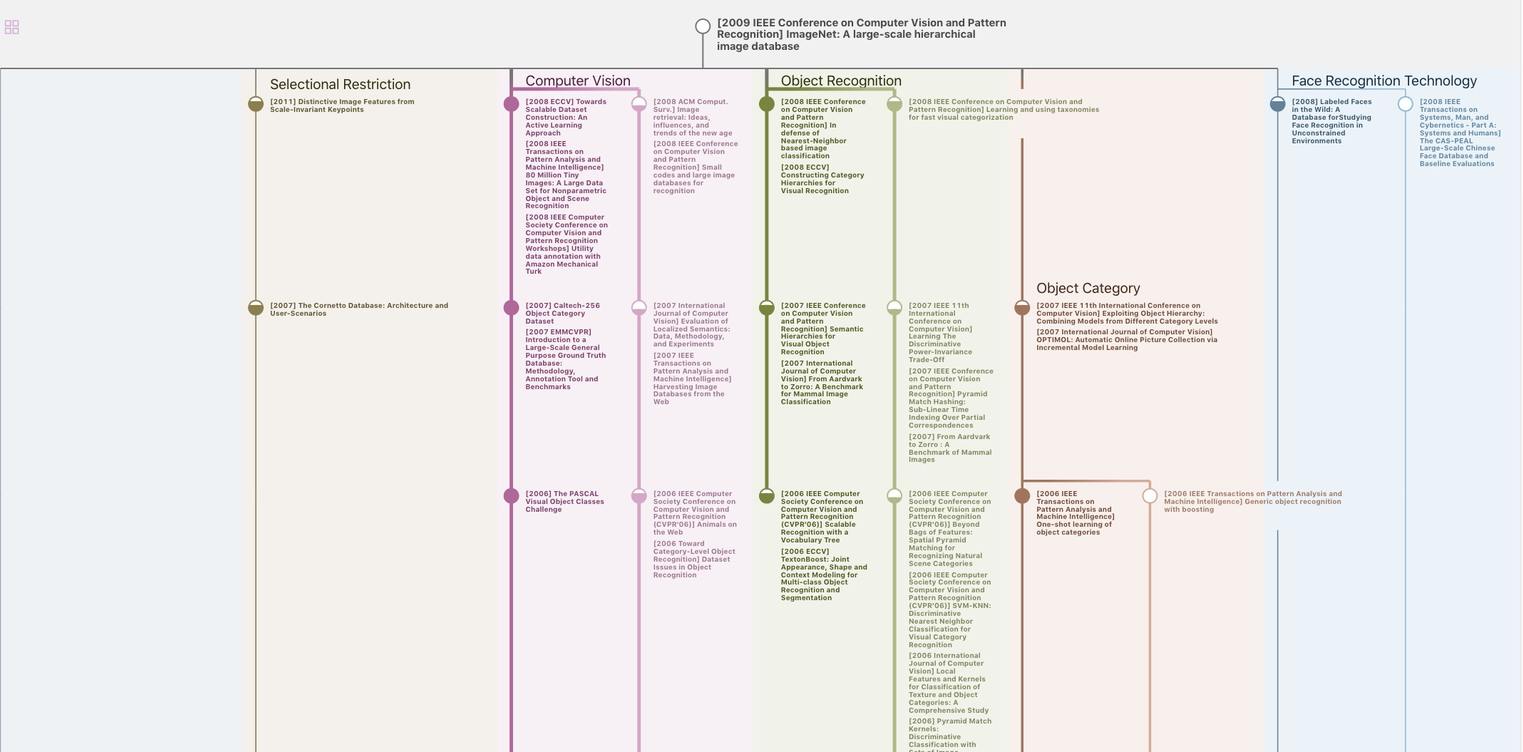
生成溯源树,研究论文发展脉络
Chat Paper
正在生成论文摘要