Federated Self-Supervised Learning for Intrusion Detection.
2023 IEEE Symposium Series on Computational Intelligence (SSCI)(2023)
摘要
Deep learning and federated learning show significant success in cybersecurity for Intrusion Detection Systems (IDS). This paper presents the Federated Self-Supervised Learning (FSSL) framework proposed for IDSs. FSSL combines Self-Supervised Learning (SSL) with federated learning to obtain a global model. SSL works at the client level, where only unlabeled data is available, and thus it enables the learning from these data. This knowledge enhances the training of the target model. Therefore, FSSL follows a federated learning approach, where private data from multiple clients help to create a global model. Each client learns an unsupervised model, which is then transmitted to a server and combined into a single model. The communication between clients and the server aims to improve model performance and convergence. Conducted experiments compare FSSL with a baseline approach using limited data and a deep learning model. FSSL utilizes an autoencoder to learn a representational model on unlabeled data and transfers knowledge by initializing deep learning model weights with the encoder layers. Results show that FSSL significantly improves the F1-Score of detection systems across three well-known datasets (NSL-KDD, TonIoT, and BotIoT). Moreover, the proposed model demonstrated a noteworthy capability to detect previously unidentified attacks when compared to the baseline.
更多查看译文
关键词
Federated Learning,Self-Supervised Learning,Intrusion Detection Systems
AI 理解论文
溯源树
样例
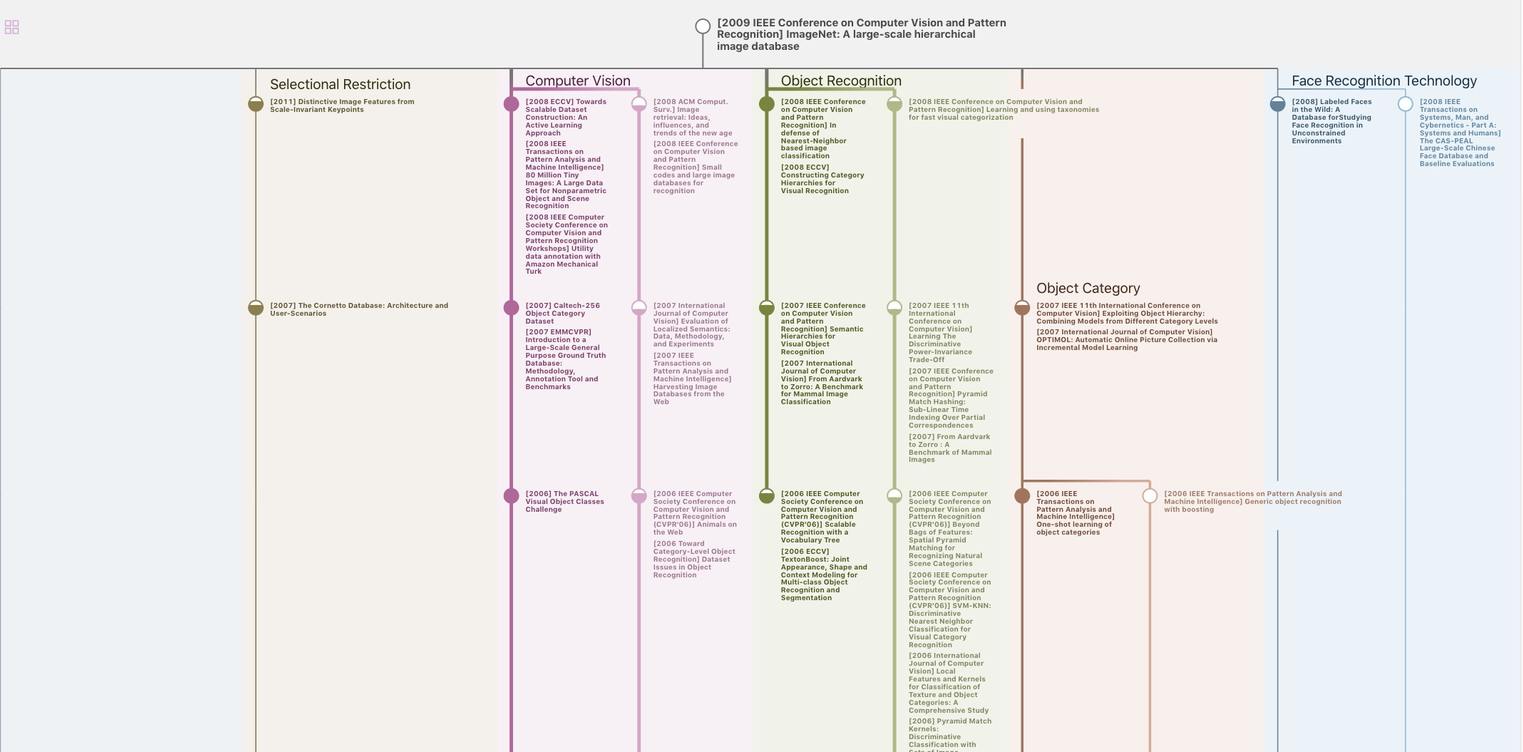
生成溯源树,研究论文发展脉络
Chat Paper
正在生成论文摘要