Carbon-efficient reaction optimization of nonoxidative direct methane conversion based on the integrated reactor system
CHEMICAL ENGINEERING JOURNAL(2024)
摘要
Methane to olefins, aromatics, and hydrogen (MTOAH) has received considerable attention because it can potentially provide an energy-efficient and environmentally friendly method for converting methane into valuable compounds. However, the optimization of the MTOAH process significantly hinders its economic feasibility because methane pyrolysis in the MTOAH process consumes considerable energy and carbon resources and requires several control factors. In this study, focusing on methane pyrolysis in the gas phase, a method for improving the MTOAH process with a hydrocarbon effect was successfully developed using metaheuristic optimization based on machine learning (ML) models. Because the trained ML models reasonably predicted various reaction outcomes, metaheuristic methods coupled with these ML models could optimize the reaction parameters to achieve the maximum hydrocarbon yield. By constructing score functions, various reaction conditions with maximum hydrocarbon yields and different coke selectivities were proposed. This provides key evidence for understanding the reaction mechanism and presents strategies for developing a sustainable cokeless catalytic reactor. Moreover, the importance of acetylene and the synergistic effect of hydrocarbon on the MTOAH process was verified using ML models. The optimal hydrocarbon content for cofeeding was determined based on the reaction temperature, but can be achieved at lower temperatures by loading a catalyst in a pre-gas phase reactor. Thus, our findings of the prioritization of the reaction parameters on the MTOAH mechanism will facilitate the development of a sustainable catalytic reactor under mild reaction conditions.
更多查看译文
关键词
Nonoxidative methane conversion,Methane pyrolysis,Ethylene,Aromatics,Machine learning,Metaheuristic optimization
AI 理解论文
溯源树
样例
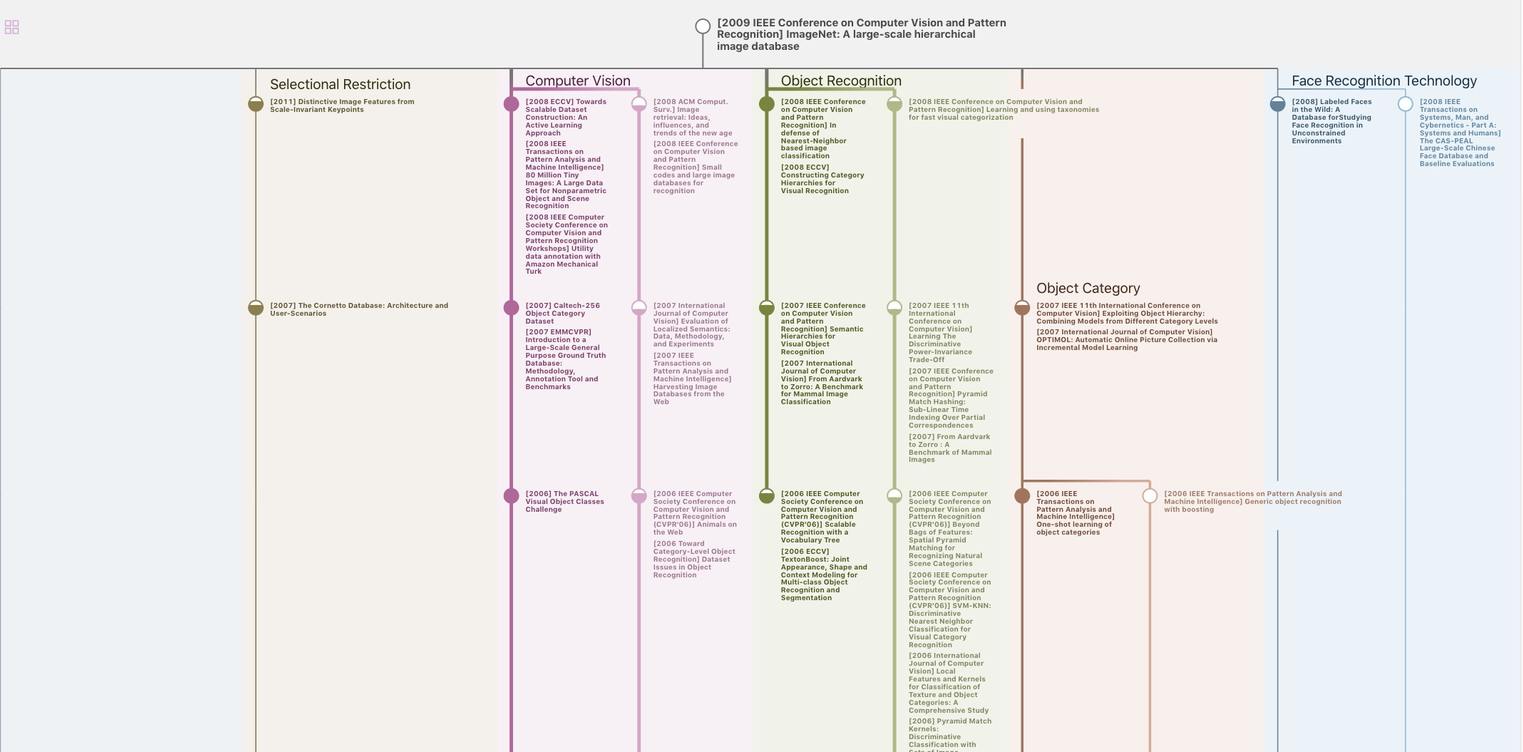
生成溯源树,研究论文发展脉络
Chat Paper
正在生成论文摘要