MSMA-Net: An Infrared Small Target Detection Network by Multiscale Super-Resolution Enhancement and Multilevel Attention Fusion
IEEE TRANSACTIONS ON GEOSCIENCE AND REMOTE SENSING(2024)
摘要
Infrared small target detection plays a crucial role in various domains like early warning, national defense, and monitoring. Although existing detection methods have achieved some good results, they only rely on the original size and information of small targets for detection and are faced with challenges, such as the small size and obscure feature information of small targets. To overcome these limitations, this article introduces a coarse-to-fine detection network named MSMA-Net. This network initially determines the rough location of targets through a coarse preliminary screening, aiming to reduce false alarms and improve computational efficiency. Simultaneously, to improve the discriminability of the features and enhance the spatial details and resolution of the targets, the network utilizes multiscale super-resolution to transform low-resolution feature maps into high-resolution representations, gradually refining and strengthening the feature representation. Finally, the network employs a multilevel feature fusion attention mechanism to facilitate effective information transmission and fusion in multiscale and multilevel feature representations. This attention mechanism enhances the accuracy as well as robustness of object detection, ultimately obtaining accurate detection results. Extensive experimental results demonstrate that compared with existing detection methods, our approach can effectively suppress false alarms and get better performance even when the target has a small size and obscure feature information.
更多查看译文
关键词
Feature extraction,Superresolution,Clutter,Object detection,Neural networks,Tensors,Data mining,Coarse to fine detection,deep learning,feature fusion,infrared small target,multiscale super-resolution (SR)
AI 理解论文
溯源树
样例
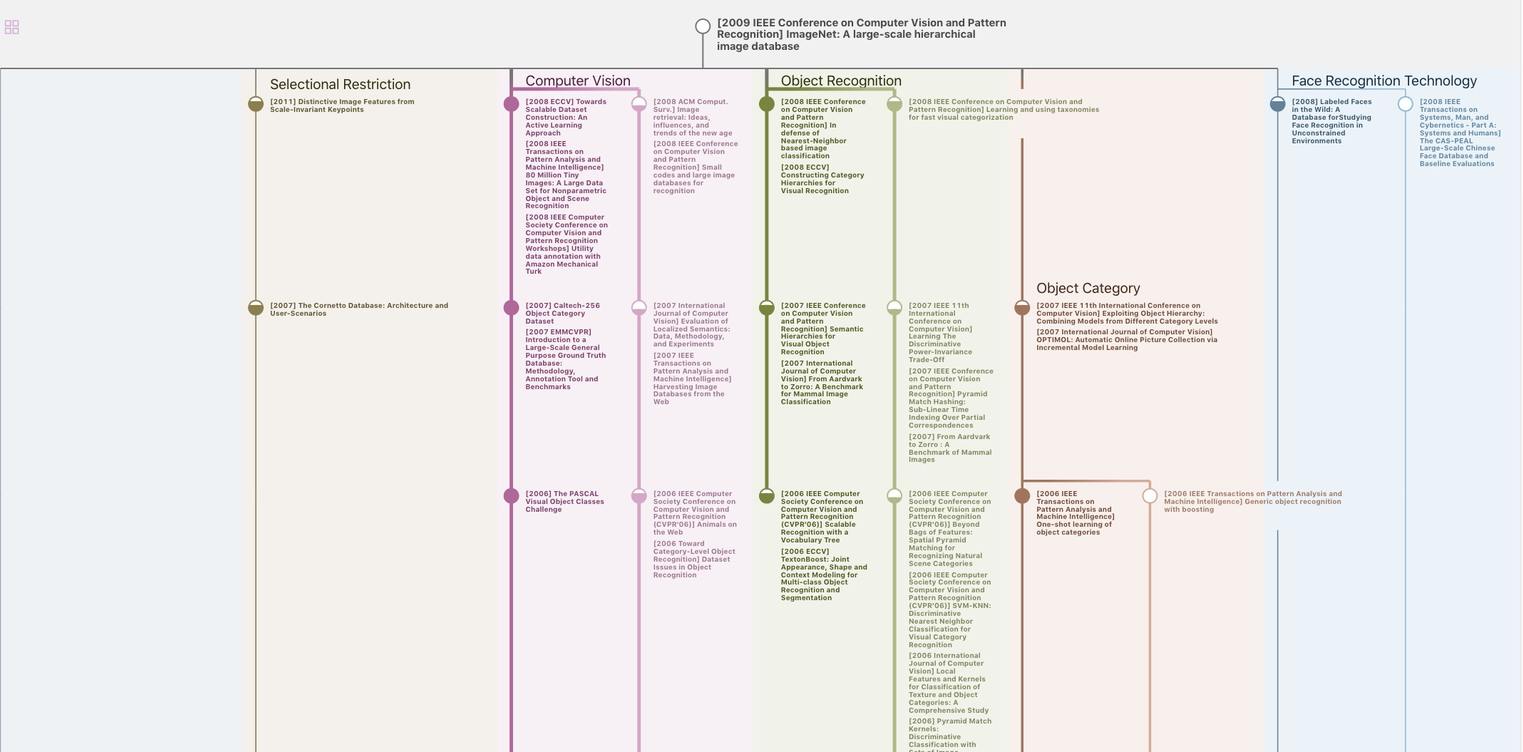
生成溯源树,研究论文发展脉络
Chat Paper
正在生成论文摘要