A Multi-class Graph Convolutional Neural Network for EEG Classification and Representation.
2023 22nd International Symposium on Communications and Information Technologies (ISCIT)(2023)
摘要
The classification of motor imagery based on EEG signals is critical for motor rehabilitation with a Brain-Computer Interface (BCI). The majority of the currently available works on this topic call for a step of subject-specific adaptation before they can be applied to a new user. Therefore, research that immediately extends a pre-trained model to new users is especially desirable. Since brain dynamics oscillate greatly between and across participants, it is difficult to build effective handcrafted features based on existing information. In an effort to fill the before-mentioned limitation, this paper offers a Graph-based Convolutional Neural Network Model (G-CNNM) to investigate EEG features across participants for motor imagery classification. Initially, a structure in the form of a graph is created with the intention of representing the location information of EEG nodes. The next step is for a convolutional neural network model to learn EEG features from both the spatial and temporal dimensions, with an emphasis placed on the temporal periods that are most distinguished. An evaluation of the proposed methodology was performed on a benchmark EEG datasetBCICIV2a- for motor imagery classification. Evidence from this study demonstrates that the G-CNNM outperforms the existing state-of-the-art approaches in terms of accuracy scoring 72.57%. In addition, the model is shown to be capable of successfully extracting detailed features through model interpretation, which also provides insights on the learning process of individual neural network components.
更多查看译文
关键词
Graph Convolutional Neural Network,Motor Imagery,EEG,Adjacency matrix
AI 理解论文
溯源树
样例
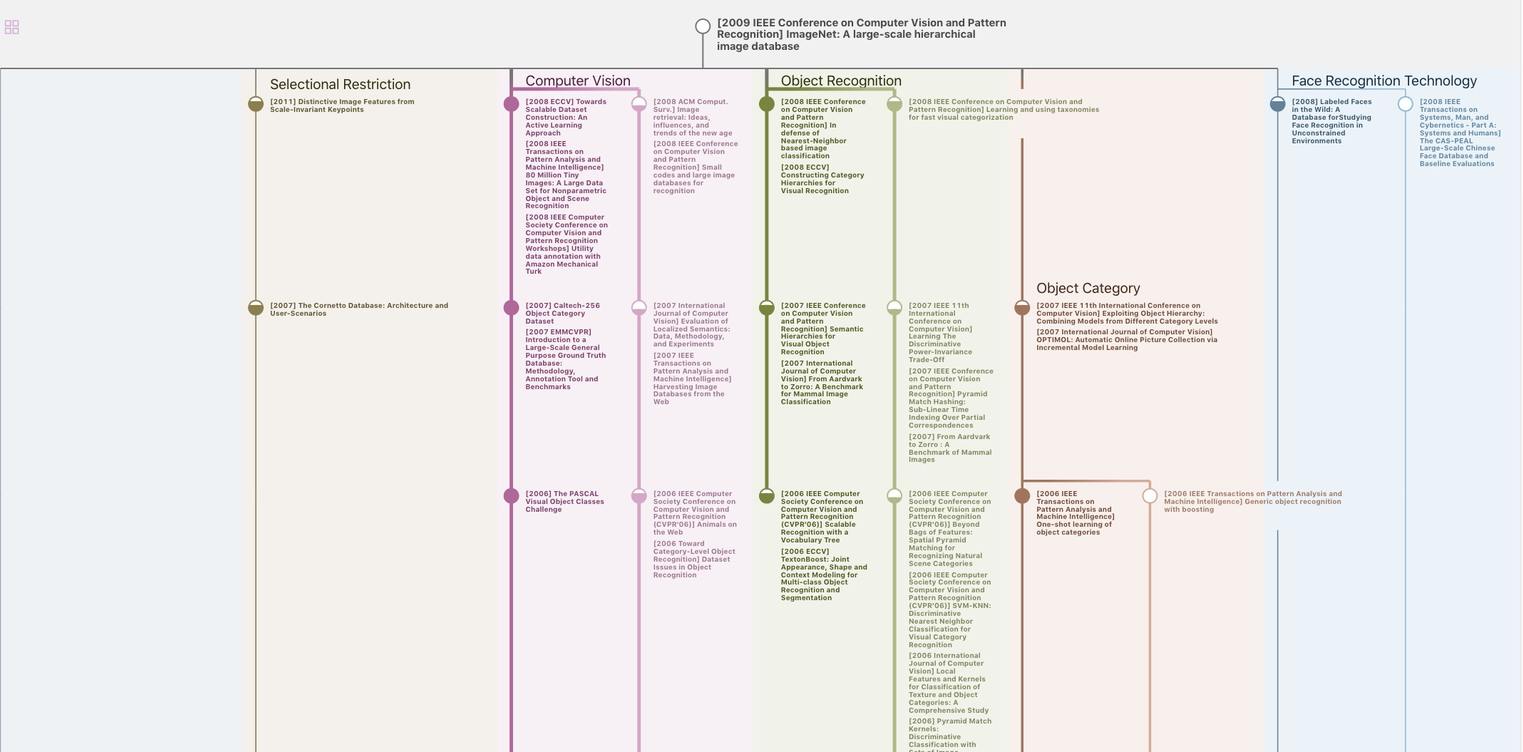
生成溯源树,研究论文发展脉络
Chat Paper
正在生成论文摘要