Power Transmission Line Component Detection using YOLO V3 on Raspberry Pi.
2023 22nd International Symposium on Communications and Information Technologies (ISCIT)(2023)
摘要
To transmit high-voltage electric power efficiently and continuously, Power Transmission Line (PTL) systems require routine inspections for early damage detection and maintenance. Previously, these inspections have been performed using line crawling, inspection robots, and a helicopter. However, these conventional solutions are sluggish, expensive, and risky. The recent development of drones, high-resolution cameras, edge computing, and deep learning technology enables the use of drones for PTL inspection. In this paper, we report the initial study of our research project in PTL inspection using an autonomous drone and deep learning. The PTL component detection is conducted in this paper. The original dataset of PTL in Tokyo is created in this research. In this research, we evaluate how large the training datasets are required to achieve a certain detection performance We also evaluate the system performance with the Raspberry Pi4 platform. To achieve those objectives, YOLOv3, with 5 different data training sizes, is evaluated in this research. The YOLOv3 model was also evaluated in Raspberry PI and notebook PC to evaluate the detection time and resource consumption performance. The simulation results show that our proposed model can achieve 96.98% precision, 91.13% recall, 93.97% F1 score, with 7.86 second detection time on Raspberry Pi4.
更多查看译文
关键词
Power line inspection,drone,Raspberry Pi,Deep learning,YOLOv3
AI 理解论文
溯源树
样例
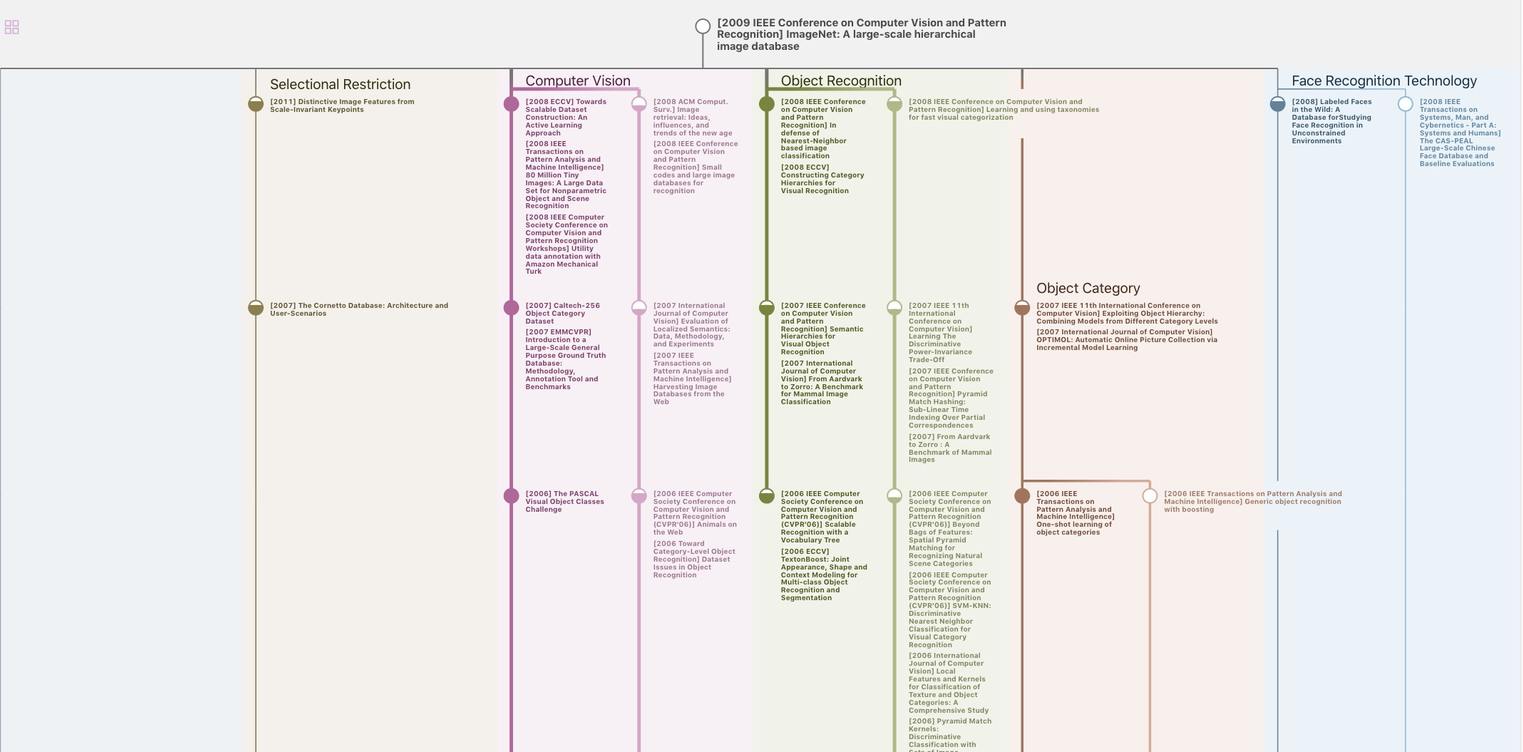
生成溯源树,研究论文发展脉络
Chat Paper
正在生成论文摘要