Automatic Transportation Mode Classification Using a Deep Reinforcement Learning Approach With Smartphone Sensors
IEEE ACCESS(2024)
摘要
The increasing dependence on smartphones with advanced sensors has highlighted the imperative of precise transportation mode classification, pivotal for domains like health monitoring and urban planning. This research is motivated by the pressing demand to enhance transportation mode classification, leveraging the potential of smartphone sensors, notably the accelerometer, magnetometer, and gyroscope. In response to this challenge, we present a novel automated classification model rooted in deep reinforcement learning. Our model stands out for its innovative approach of harnessing enhanced features through artificial neural networks (ANNs) and visualizing the classification task as a structured series of decision-making events. Our model adopts an improved differential evolution (DE) algorithm for initializing weights, coupled with a specialized agent-environment relationship. Every correct classification earns the agent a reward, with additional emphasis on the accurate categorization of less frequent modes through a distinct reward strategy. The Upper Confidence Bound (UCB) technique is used for action selection, promoting deep-seated knowledge, and minimizing reliance on chance. A notable innovation in our work is the introduction of a cluster-centric mutation operation within the DE algorithm. This operation strategically identifies optimal clusters in the current DE population and forges potential solutions using a pioneering update mechanism. When assessed on the extensive HTC dataset, which includes 8311 hours of data gathered from 224 participants over two years. Noteworthy results spotlight an accuracy of 0.88 +/- 0.03 and an F-measure of 0.87 +/- 0.02, underscoring the efficacy of our approach for large-scale transportation mode classification tasks. This work introduces an innovative strategy in the realm of transportation mode classification, emphasizing both precision and reliability, addressing the pressing need for enhanced classification mechanisms in an ever-evolving digital landscape.
更多查看译文
关键词
Transportation mode,sensor,smart phone,artificial neural network,reinforcement learning,differential evolution
AI 理解论文
溯源树
样例
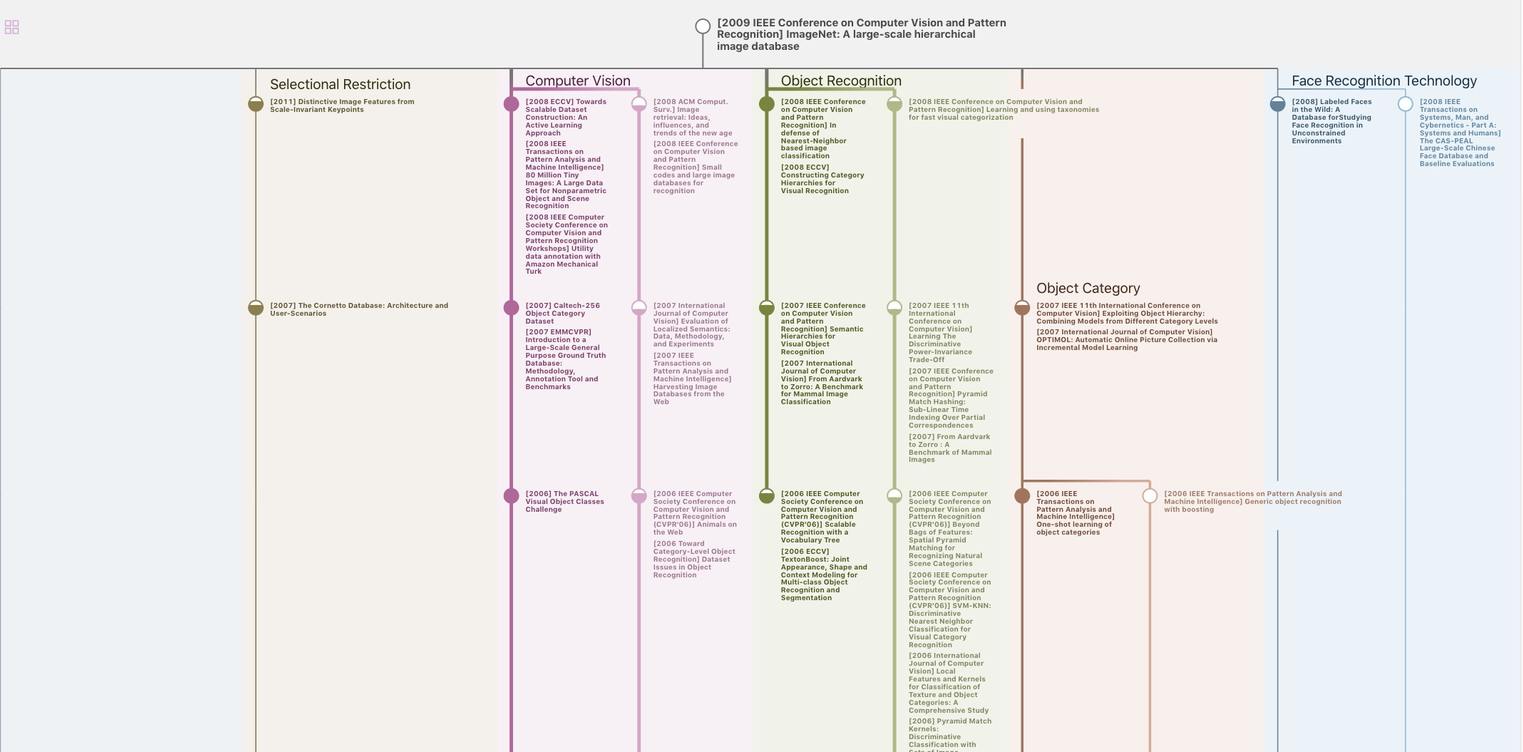
生成溯源树,研究论文发展脉络
Chat Paper
正在生成论文摘要