Deep Blind Chest X-Ray Image Quality Assessment With Region-of-Interest-Guided Attention
IEEE MULTIMEDIA(2023)
摘要
The image quality assessment (IQA) for medical images has been challenging due to their usage for diagnostic purposes. Traditional convolutional-neural-network-based IQA models usually assess the image quality on a global scale, which is unsuitable for inferring the local quality of medical images from the diagnostic attention perspective. To alleviate this problem, we design and introduce a region-of-interest (ROI)-guided attention mechanism in medical IQA and propose a novel dual attention IQA model. In addition, we have also constructed a diagnosis-oriented medical image dataset containing 1132 images, all rated by experienced radiologists subjectively, to allow effective training for the proposed model. Our experimental results show that the proposed ROI-guided dual attention IQA model can well reflect radiologists' attention for diagnostic purposes. Compared with the state-of-the-art IQA models, the IQA output of the proposed model is significantly more consistent with subjective evaluation by experienced radiologists.
更多查看译文
关键词
Medical diagnostic imaging,Image quality,Feature extraction,Convolutional neural networks,Training,Computational modeling,X-ray imaging
AI 理解论文
溯源树
样例
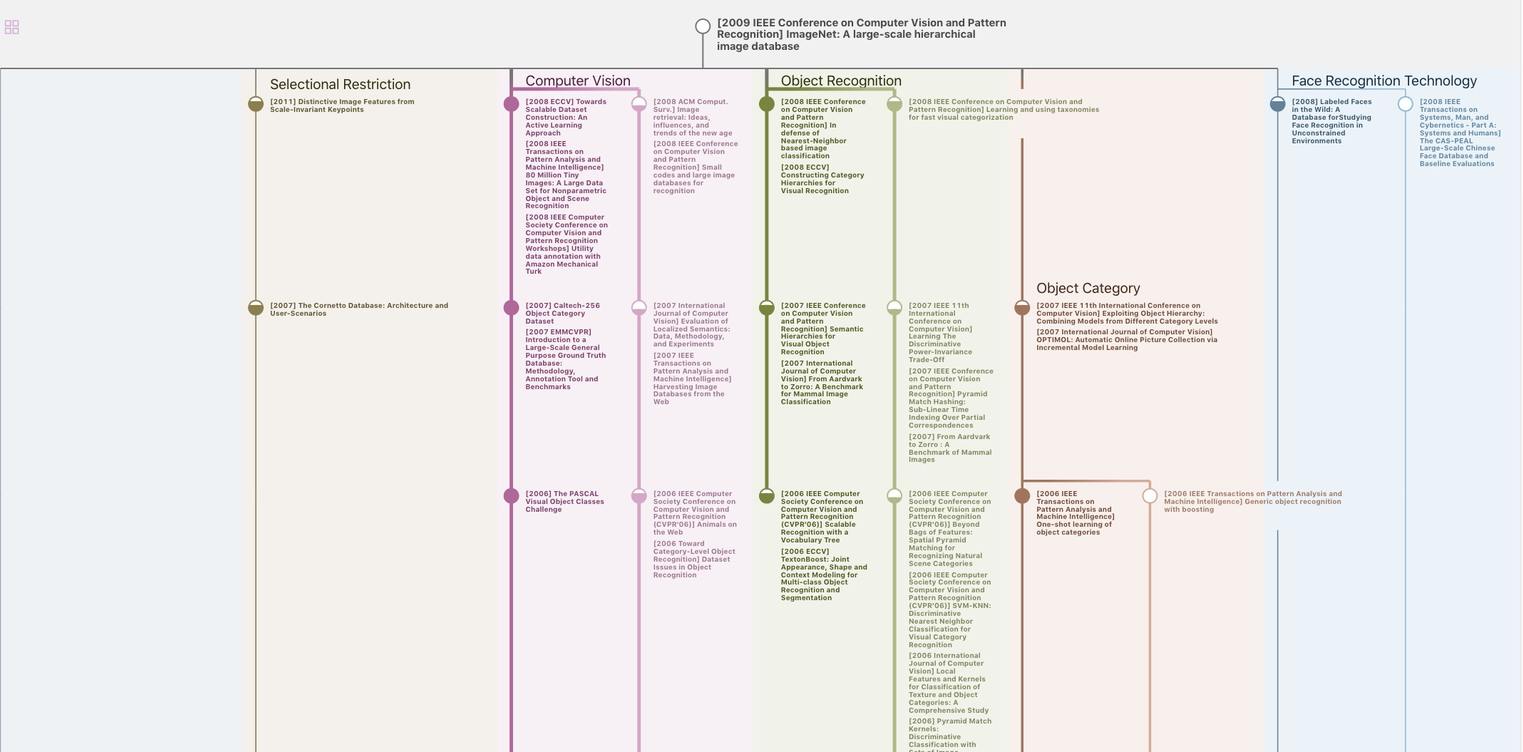
生成溯源树,研究论文发展脉络
Chat Paper
正在生成论文摘要