Machine Learning Approaches for the Solution of the Riemann Problem in Fluid Dynamics: a Case Study
Communications on Applied Mathematics and Computation(2024)
摘要
We present our results by using a machine learning (ML) approach for the solution of the Riemann problem for the Euler equations of fluid dynamics. The Riemann problem is an initial-value problem with piecewise-constant initial data and it represents a mathematical model of the shock tube. The solution of the Riemann problem is the building block for many numerical algorithms in computational fluid dynamics, such as finite-volume or discontinuous Galerkin methods. Therefore, a fast and accurate approximation of the solution of the Riemann problem and construction of the associated numerical fluxes is of crucial importance. The exact solution of the shock tube problem is fully described by the intermediate pressure and mathematically reduces to finding a solution of a nonlinear equation. Prior to delving into the complexities of ML for the Riemann problem, we consider a much simpler formulation, yet very informative, problem of learning roots of quadratic equations based on their coefficients. We compare two approaches: (i) Gaussian process (GP) regressions, and (ii) neural network (NN) approximations. Among these approaches, NNs prove to be more robust and efficient, although GP can be appreciably more accurate (about 30% ). We then use our experience with the quadratic equation to apply the GP and NN approaches to learn the exact solution of the Riemann problem from the initial data or coefficients of the gas equation of state (EOS). We compare GP and NN approximations in both regression and classification analysis and discuss the potential benefits and drawbacks of the ML approach.
更多查看译文
关键词
Machine learning (ML),Neural network (NN),Gaussian process (GP),Riemann problem,Numerical fluxes,Finite-volume method
AI 理解论文
溯源树
样例
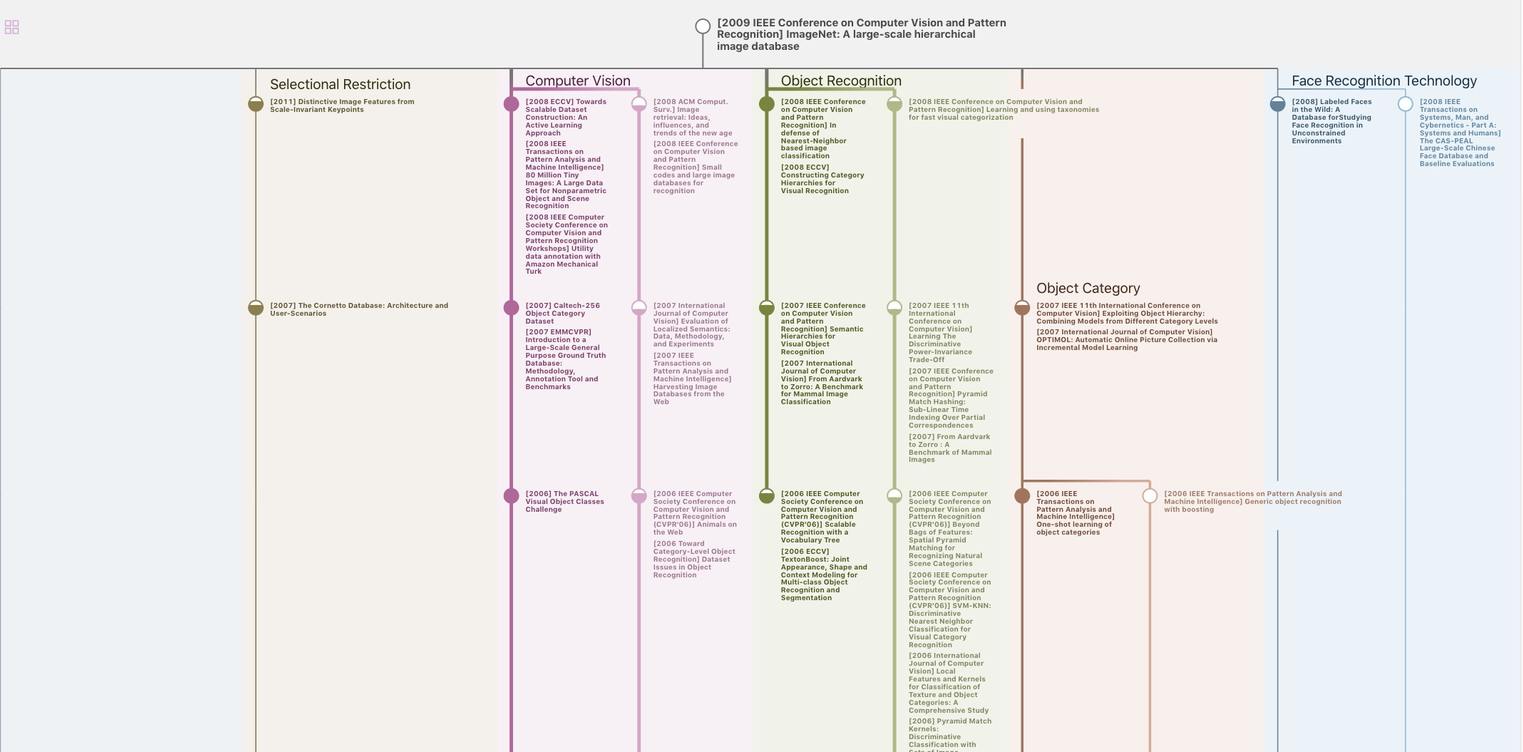
生成溯源树,研究论文发展脉络
Chat Paper
正在生成论文摘要