Privacy-preserving vertical federated broad learning system for artificial intelligence generated image content
Journal of Real-Time Image Processing(2024)
摘要
Artificial intelligence generated image content generates desired image content according to given instructions. It is widely applied in digital marketing, video game design, and movie production. How to efficiently detect the quality of the generated images is one of the key problems. A broad learning system is a feasible solution, because it requires no complex parameter calculation and depth expansion. However, the broad learning system is built by centralized learning, so it may not take advantage of isolated feature data, which prevents broad learning systems from detecting isolated AL-generated image content. To address the above problem, this paper proposes a privacy-preserving vertical federated broad learning system for artificial intelligence generated image content (PVF-BLS). In PVF-BLS, the vertical federated architecture is introduced into the broad learning system to utilize isolated feature data and interactive data are masked by matrix masks for security. To further improve the security of PVF-BLS, this paper proposes a secure incremental learning algorithm based on matrix masks (ILA-MM) to update PVF-BLS. In ILA-MM, the interaction data are processed by matrix masks, which makes PVF-BLS more secure. Experimental results show that PVF-BLS outperforms the traditional broad learning systems when the data are isolated.
更多查看译文
关键词
Broad learning system,Vertical federation,Incremental learning algorithm,Isolated data,Artificial intelligence generated content
AI 理解论文
溯源树
样例
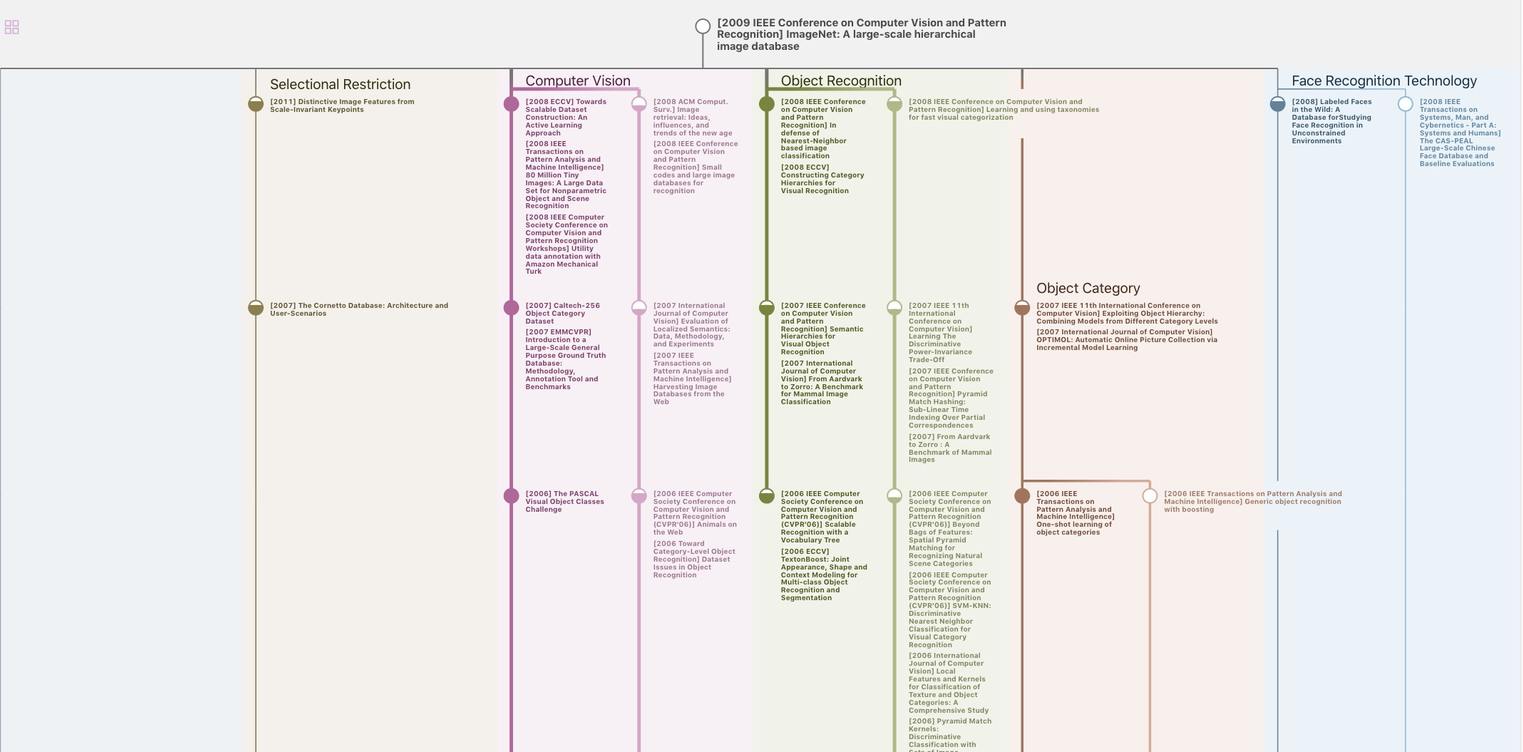
生成溯源树,研究论文发展脉络
Chat Paper
正在生成论文摘要