Residual Diffusion Modeling for Km-scale Atmospheric Downscaling
crossref(2024)
摘要
Abstract Predictions of weather hazard require expensive km-scale simulations driven by coarser global inputs. Here, a cost-effective stochastic downscaling model is trained from a high-resolution 2-km weather model over Taiwan conditioned on 25-km ERA5 reanalysis. To address the multi-scale machine learning challenges of weather data, we employ a two-step approach Corrector Diffusion (CorrDiff), where a UNet prediction of the mean is corrected by a diffusion step. Akin to Reynolds decomposition in fluid dynamics, this isolates generative learning to the stochastic scales. CorrDiff exhibits skillful RMSE and CRPS and faithfully recovers spectra and distributions even for extremes. Case studies of coherent weather phenomena reveal appropriate multivariate relationships reminiscent of learnt physics: the collocation of intense rainfall and sharp gradients in fronts and extreme winds and rainfall bands near the eyewall of typhoons. Downscaling global forecasts successfully retains many of these benefits, foreshadowing the potential of end-to-end, global-to- km-scales machine learning weather predictions.
更多查看译文
AI 理解论文
溯源树
样例
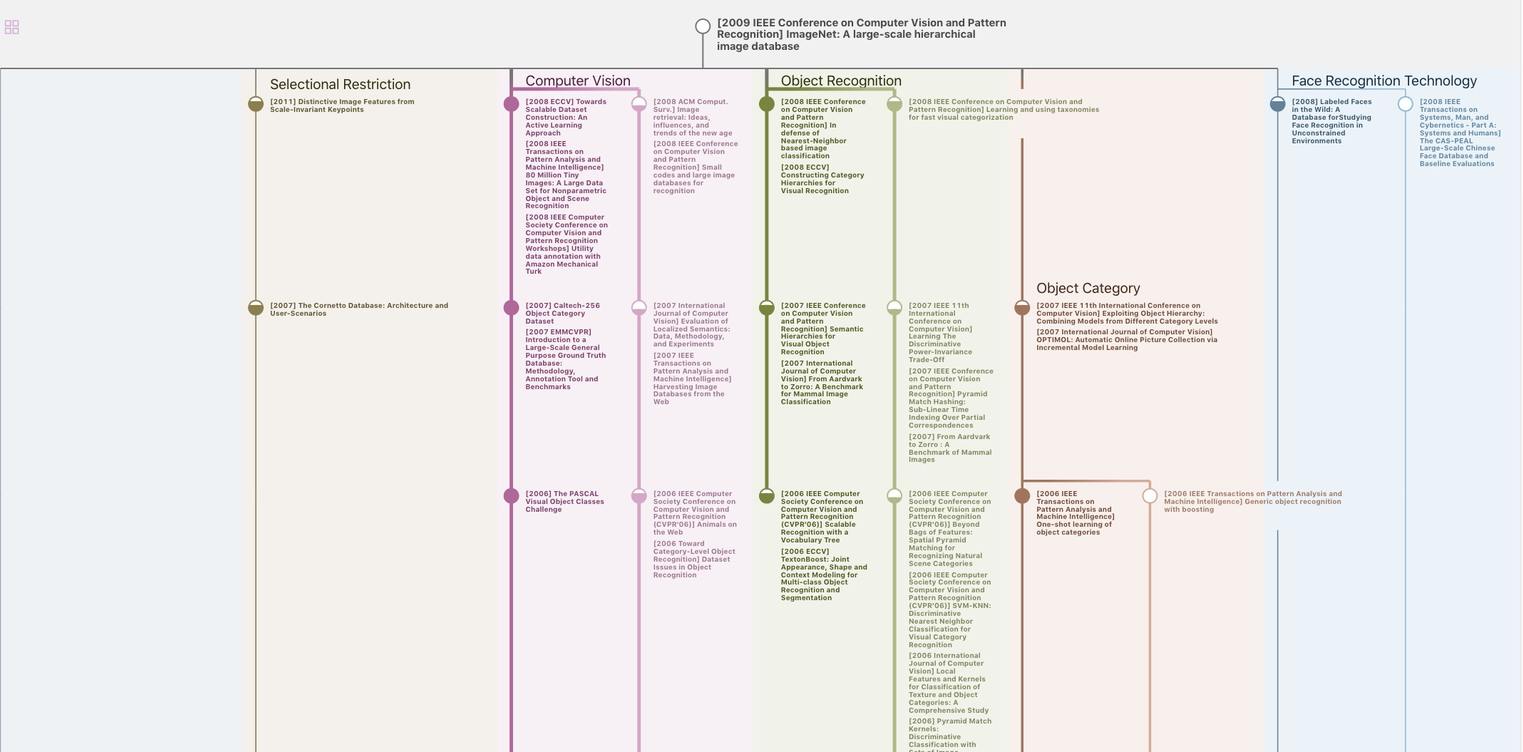
生成溯源树,研究论文发展脉络
Chat Paper
正在生成论文摘要