FedACT: an adaptive chained training approach for federated learning in computing power networks
Digital Communications and Networks(2024)
摘要
Federated Learning (FL) is a novel distributed machine learning methodology that addresses large-scale parallel computing challenges while safeguarding data security. However, the traditional FL model in communication scenarios, whether for uplink or downlink communications, may give rise to several network problems, such as bandwidth occupation, additional network latency, and bandwidth fragmentation. In this paper, we propose an adaptive chained training approach (FedACT) for FL in computing power networks. First, a Computation-driven Clustering Strategy (CCS) is designed. The server clusters clients by task processing delays to minimize waiting delays at the central server. Second, we propose a Genetic-Algorithm-based Sorting (GAS) method to optimize the order of clients participating in training. Finally, based on the table lookup and forwarding rules of the Segment Routing over IPv6 (SRv6) protocol, the sorting results of GAS are written into the SRv6 packet header, to control the order in which clients participate in model training. We conduct extensive experiments on two datasets of CIFAR-10 and MNIST, and the results demonstrate that the proposed algorithm offers improved accuracy, diminished communication costs, and reduced network delays.
更多查看译文
关键词
Computing power network (CPN),Federated learning (FL),Segment routing IPv6 (SRv6),Communication overheads,Model accuracy
AI 理解论文
溯源树
样例
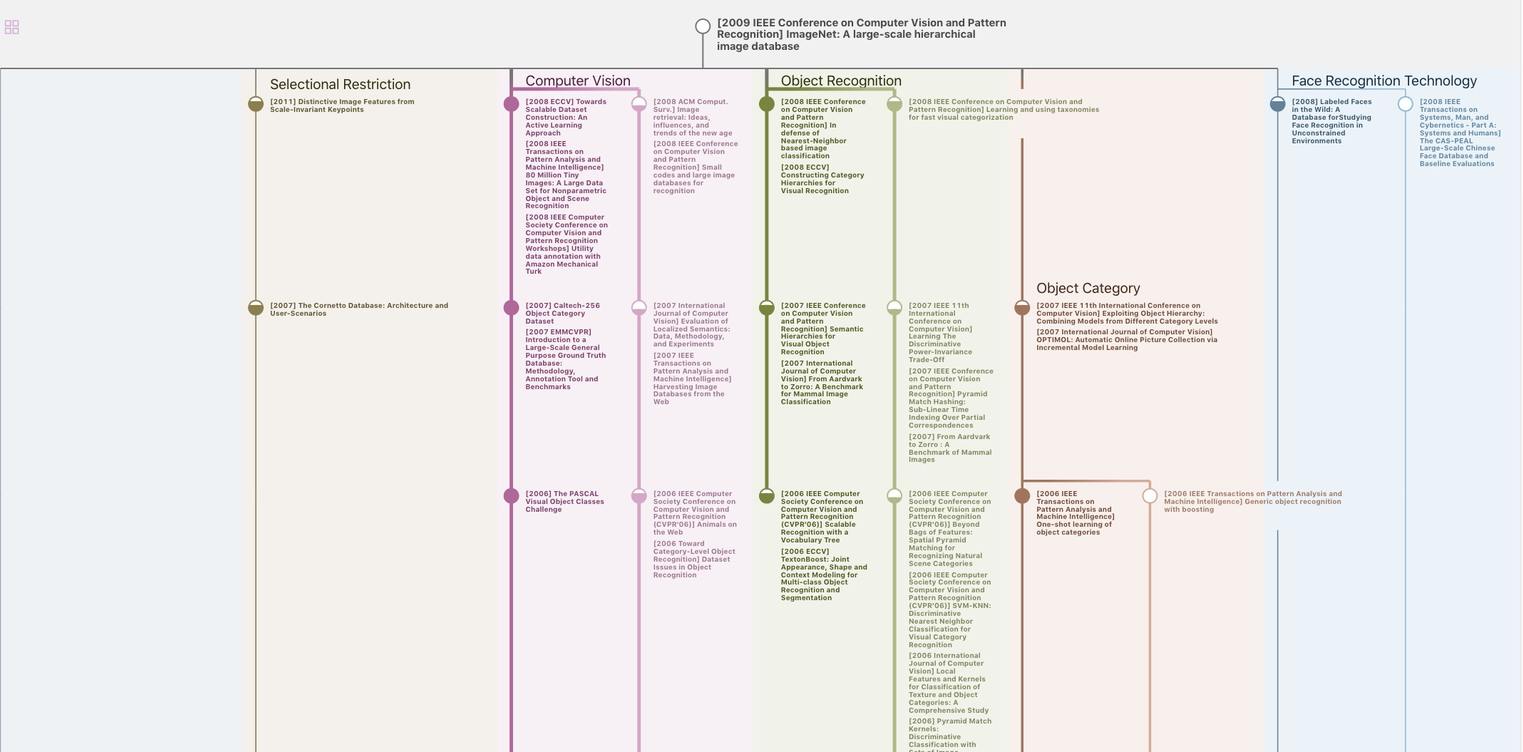
生成溯源树,研究论文发展脉络
Chat Paper
正在生成论文摘要