Freeze the backbones: A Parameter-Efficient Contrastive Approach to Robust Medical Vision-Language Pre-training
CoRR(2024)
摘要
Modern healthcare often utilises radiographic images alongside textual
reports for diagnostics, encouraging the use of Vision-Language Self-Supervised
Learning (VL-SSL) with large pre-trained models to learn versatile medical
vision representations. However, most existing VL-SSL frameworks are trained
end-to-end, which is computation-heavy and can lose vital prior information
embedded in pre-trained encoders. To address both issues, we introduce the
backbone-agnostic Adaptor framework, which preserves medical knowledge in
pre-trained image and text encoders by keeping them frozen, and employs a
lightweight Adaptor module for cross-modal learning. Experiments on medical
image classification and segmentation tasks across three datasets reveal that
our framework delivers competitive performance while cutting trainable
parameters by over 90
when fine-tuned with just 1
Transformer-based methods trained on full datasets in medical image
segmentation.
更多查看译文
关键词
Vision-Language Pre-training,SelfSupervised Learning,Medical Visual Representation Learning
AI 理解论文
溯源树
样例
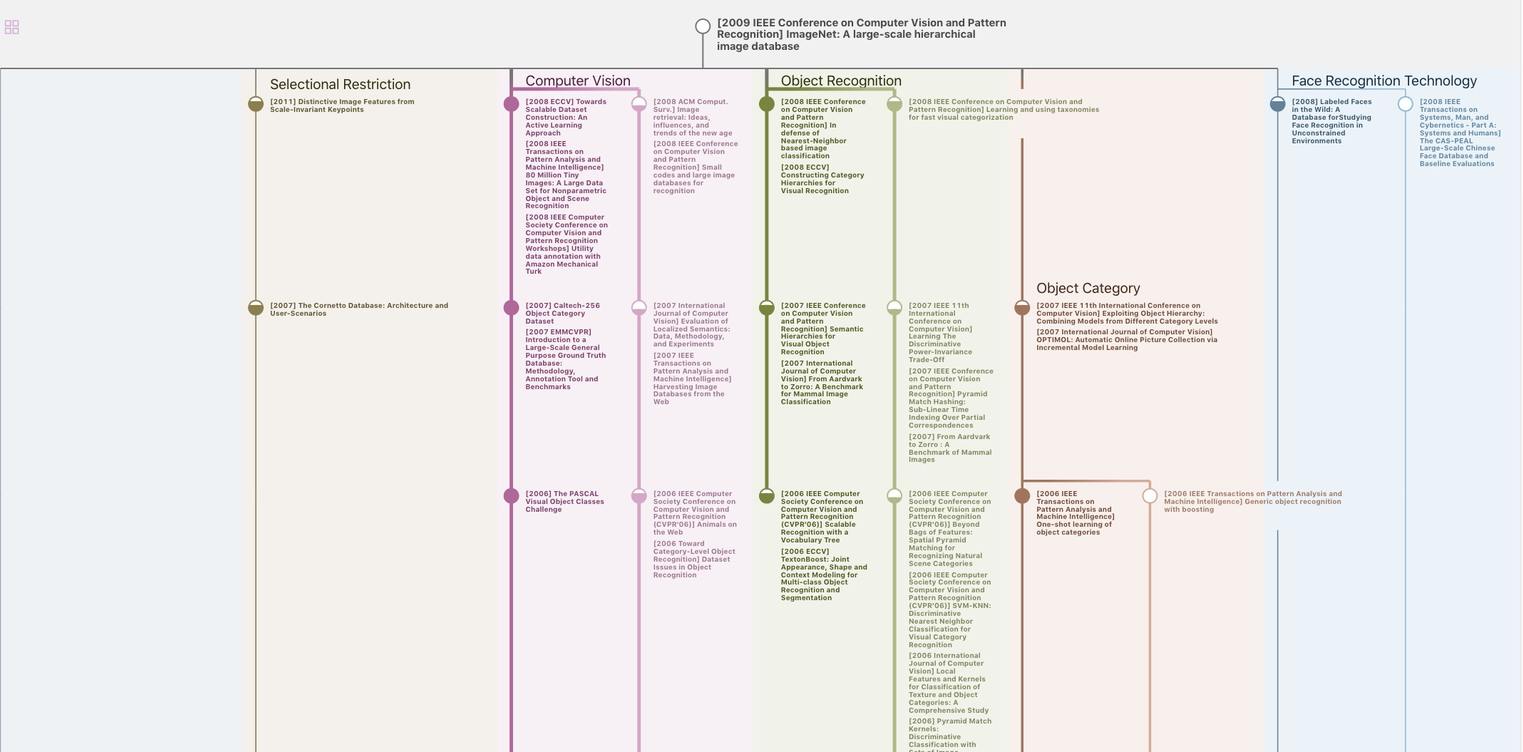
生成溯源树,研究论文发展脉络
Chat Paper
正在生成论文摘要