Towards fair and personalized federated recommendation
PATTERN RECOGNITION(2024)
摘要
Recommender systems have gained immense popularity in recent years for predicting users' interests by learning embeddings. The majority of existing recommendation approaches, represented by graph neural network-based recommendation algorithms, rely on centralized storage of user-item graphs for model learning, which raises privacy issues in the process of collecting and sharing user data. Federated recommendation can mitigate privacy concerns by preventing the server from collecting sensitive data from clients while there still exist unfairness and personalization issues. To address these challenges, we propose a novel framework named Fair and Personalized Federated Recommendation (FPFR). On the client-side, the soft attention mechanism is designed to learn the representation of user/item by combining interaction and attribute information, and the filter network is combined to better characterize user preferences. On the server-side, we cluster users into different groups and learn personalized models for each user. Then, we select representative users from each group to participate in the global model parameters update. Finally, the fairness of federated recommendation is implemented by adding the fairness constraint to recommendation loss. We conduct experiments on five real-world recommendation datasets, and the results demonstrate that the proposed FPFR not only balances group fairness and recommendation accuracy but also improves personalization.
更多查看译文
关键词
Federated learning,Fairness,Graph neural network,Personalized recommendation
AI 理解论文
溯源树
样例
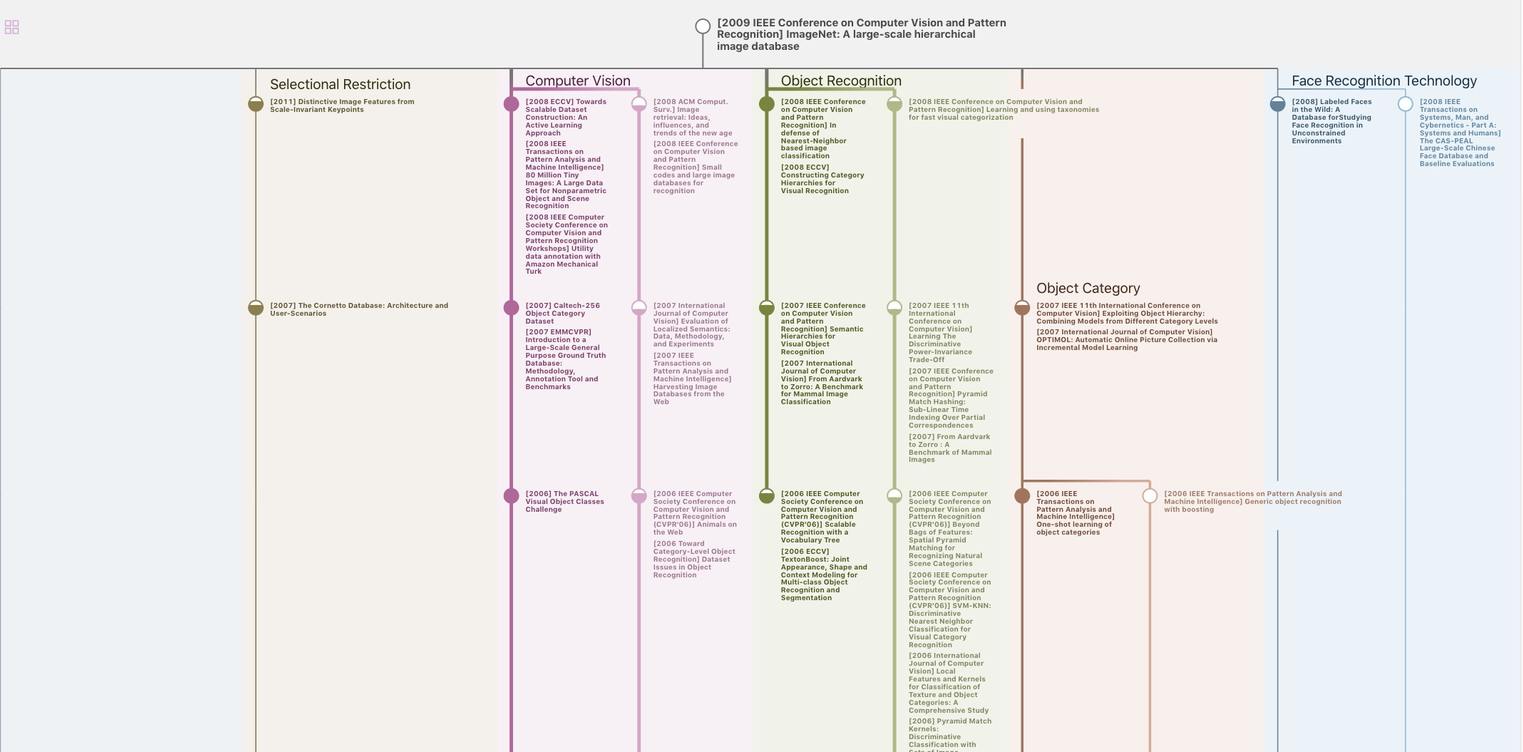
生成溯源树,研究论文发展脉络
Chat Paper
正在生成论文摘要