Water cut estimation using electrical submersible pump mechanical vibrations and convolutional neural networks.
2023 8th International Conference on Frontiers of Signal Processing (ICFSP)(2023)
摘要
In oil production, the water content within the oil typically increases as wells mature. The Electrical Submersible Pumps (ESPs) have been extensively used in these wells. This research aims to establish a correlation between vibration signals from ESPs and the water fraction in oil-water mixtures, different from the conventional focus on fault diagnosis. Using an eight-stage ESP equipped with an accelerometer at its inlet, tests were conducted at varying flow rates and water cut percentages. The study adopted a data-driven approach, implementing a Convolutional Neural Network (CNN) via the PyTorch library. The convex hull was used as a data preprocessing technique to obtain a more uniform dataset. Additionally, the CNN underwent a hyperparameter optimization using Optuna. The trained CNN exhibited a coefficient of determination (R
2
) of 0.952, with 73.44% of its predictions residing within an error margin of ±5% for an independent test dataset. While the current approach is promising, it underscores the need for more extensive experimental datasets. Future research might explore alternative neural network architectures, such as the Fourier convolutional neural networks (FCNN) and various pump datasets.
更多查看译文
关键词
Electrical submersible pump,Vibration,Convolutional neural networks,Two-phase flow
AI 理解论文
溯源树
样例
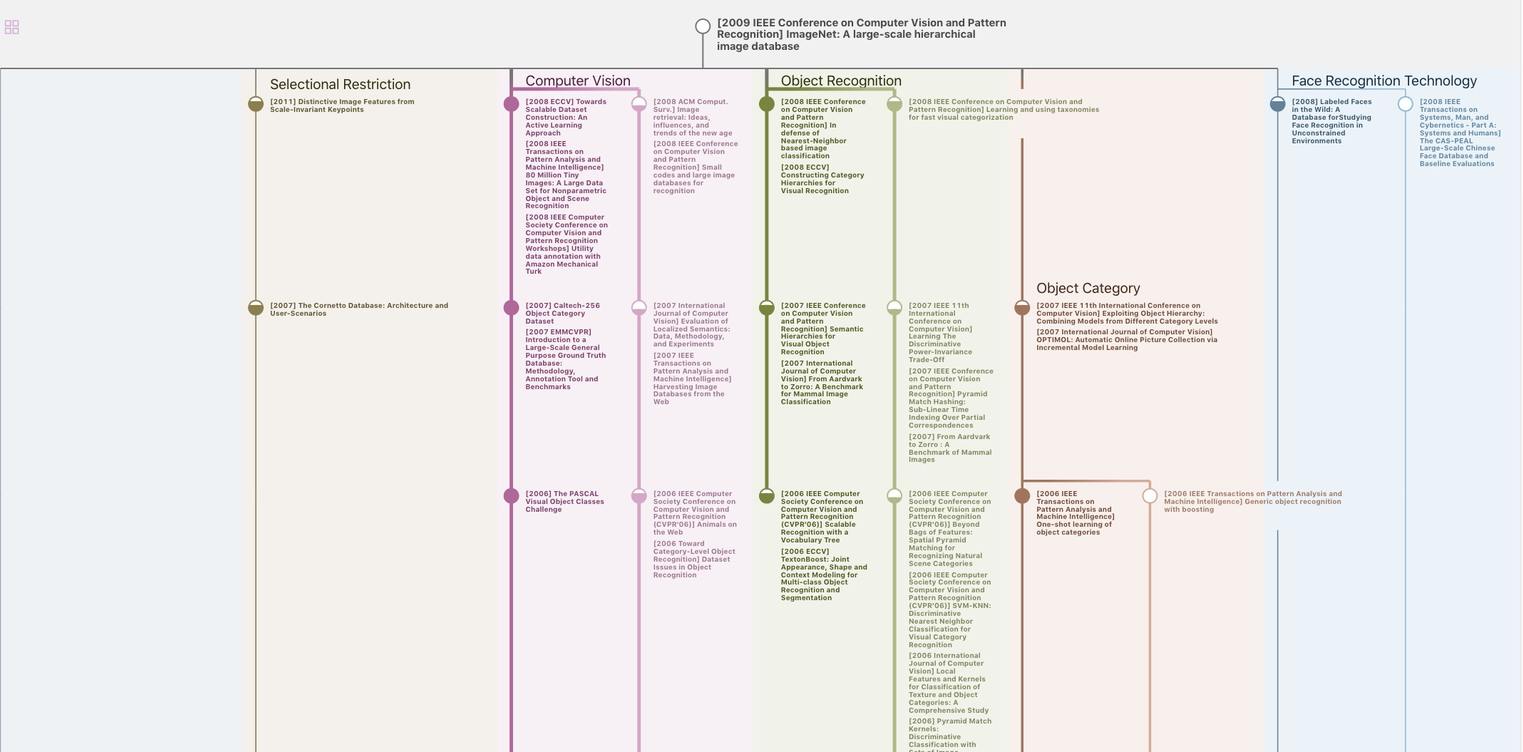
生成溯源树,研究论文发展脉络
Chat Paper
正在生成论文摘要