Analysis of Partition Methods for Dominated Solution Removal from Large Solution Sets.
2023 IEEE Symposium Series on Computational Intelligence (SSCI)(2023)
摘要
In evolutionary multi-objective optimization (EMO), one important issue is to efficiently remove dominated solutions from a large number of solutions examined by an EMO algorithm. An efficient approach to remove dominated solutions from a large solution set is to partition it into small subsets. Dominated solutions are removed from each subset independently. This partition method is fast but cannot guarantee to remove all dominated solutions. To further remove remaining dominated solutions, a simple idea is to iteratively perform this approach. In this paper, we first examine three partition methods (random, objective value-based and cosine similarity-based methods) and their iterative versions through computational experiments on artificial test problems (DTLZ and WFG) and real-world problems. Our results show that the choice of an appropriate partition method is problem dependent. This observation motivates us to use a hybrid approach where different partition methods are used in an iterative manner. The results show that all dominated solutions are removed by the hybrid approach in most cases. Then, we examine the effects of the following factors on the computation time and the removal performance: the number of objectives, the shape of the Pareto front, and the number of subsets in each partition method.
更多查看译文
AI 理解论文
溯源树
样例
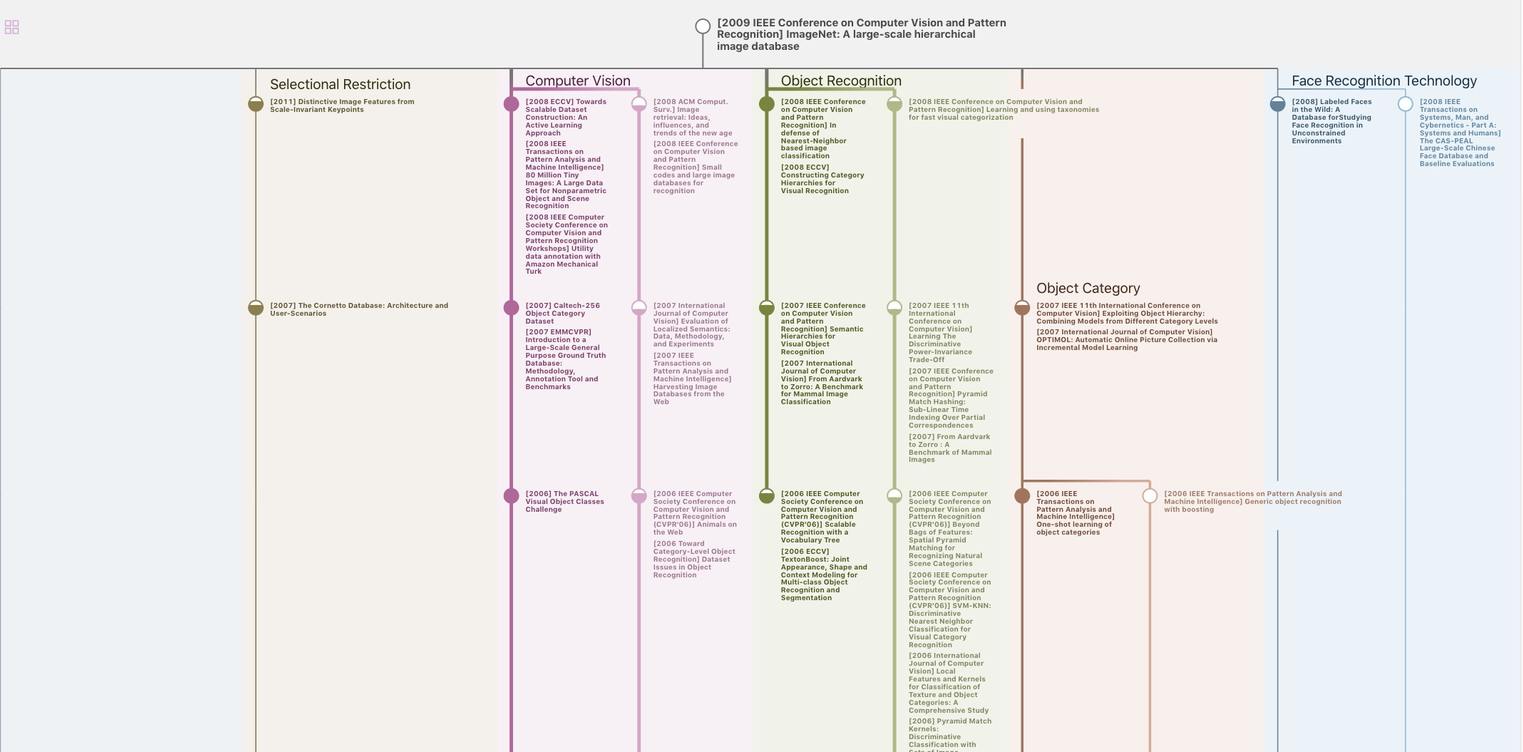
生成溯源树,研究论文发展脉络
Chat Paper
正在生成论文摘要