Fuzzy Detectors Against Adversarial Attacks.
2023 IEEE Symposium Series on Computational Intelligence (SSCI)(2023)
摘要
Deep learning-based methods have proved useful for adversarial attack detection. However, conventional detection algorithms exploit crisp set theory for classification boundary. Therefore, representing vague concepts is not available. Mo-tivated by the recent success in fuzzy systems, we propose a fuzzy rule-based neural network to improve adversarial attack detection accuracy. The pretrained ImageNet model is exploited to extract feature maps from clean and attacked images. Subsequently, the fuzzification network is used to obtain feature maps to produce fuzzy sets of difference degrees between clean and attacked images. The fuzzy rules control the intelligence that determines the detection boundaries. In the defuzzification layer, the fuzzy prediction from the in-telligence is mapped back into the crisp model predictions for images. The loss between the prediction and label controls the rules to train the fuzzy detector. We show that the fuzzy rule-based network learns rich feature information than binary outputs and offers to obtain an overall performance gain. Our experiments, conducted over a wide range of images, show that the proposed method consistently performs better than conventional crisp set training in adversarial attack detection with various fuzzy system-based neural networks. The source code of the proposed method is available at https://github.com/Yukino-3/Fuzzy.
更多查看译文
关键词
Deep learning,adversarial attack detection,classification boundary,fuzzy rule,fuzzy prediction
AI 理解论文
溯源树
样例
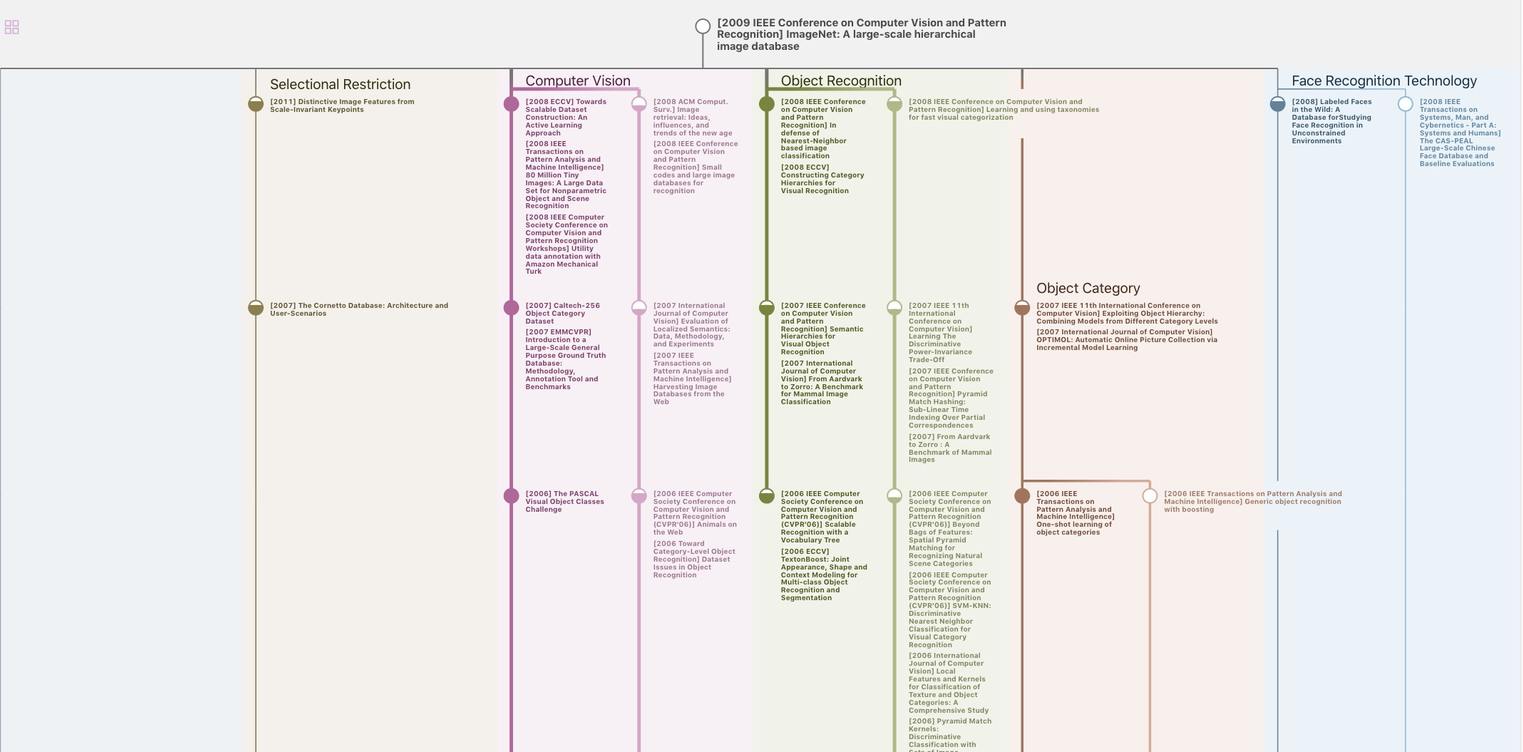
生成溯源树,研究论文发展脉络
Chat Paper
正在生成论文摘要