Physics Informed Data Driven Techniques for Power Flow Analysis.
2023 IEEE Symposium Series on Computational Intelligence (SSCI)(2023)
摘要
The last decade has seen significant changes in the power grid complexity due to the increased integration of multiple heterogeneous distributed energy resources. Accurate and fast power flow analysis tools have then become essential to guarantee grid stability, reliable operation, strategic planning, and market strategies. State-of-the-art approaches to power flow analysis are based on iterative numerical techniques which exhibit high accuracy but slow-, or even no-, convergence. For this reason, researchers have investigated the use of data-driven techniques that, while exhibiting lower accuracy with respect to iterative numerical ones, have the advantage of being extremely fast. To address the lack of accuracy, physics-informed data-driven techniques, i.e., techniques that leverage both the data and domain knowledge to generate simultaneously fast and accurate models, have been proposed. Nevertheless, these works exhibit two main limitations: i) they do not fully leverage the physical knowledge, and ii) they do not fairly compare the different approaches. In this paper, we propose a novel physics informed data-driven model able to address both limitations by fully leveraging the physical knowledge into the data-driven, i.e., constraining the model and augmenting the available data, and proposing a framework able to fairly compare the different approaches proving the actual effectiveness of the proposal. Results on the IEEE 57 realistic power network will support the proposal.
更多查看译文
关键词
Physics Informed,Machine Learning,Power Systems,Power Flow Analysis
AI 理解论文
溯源树
样例
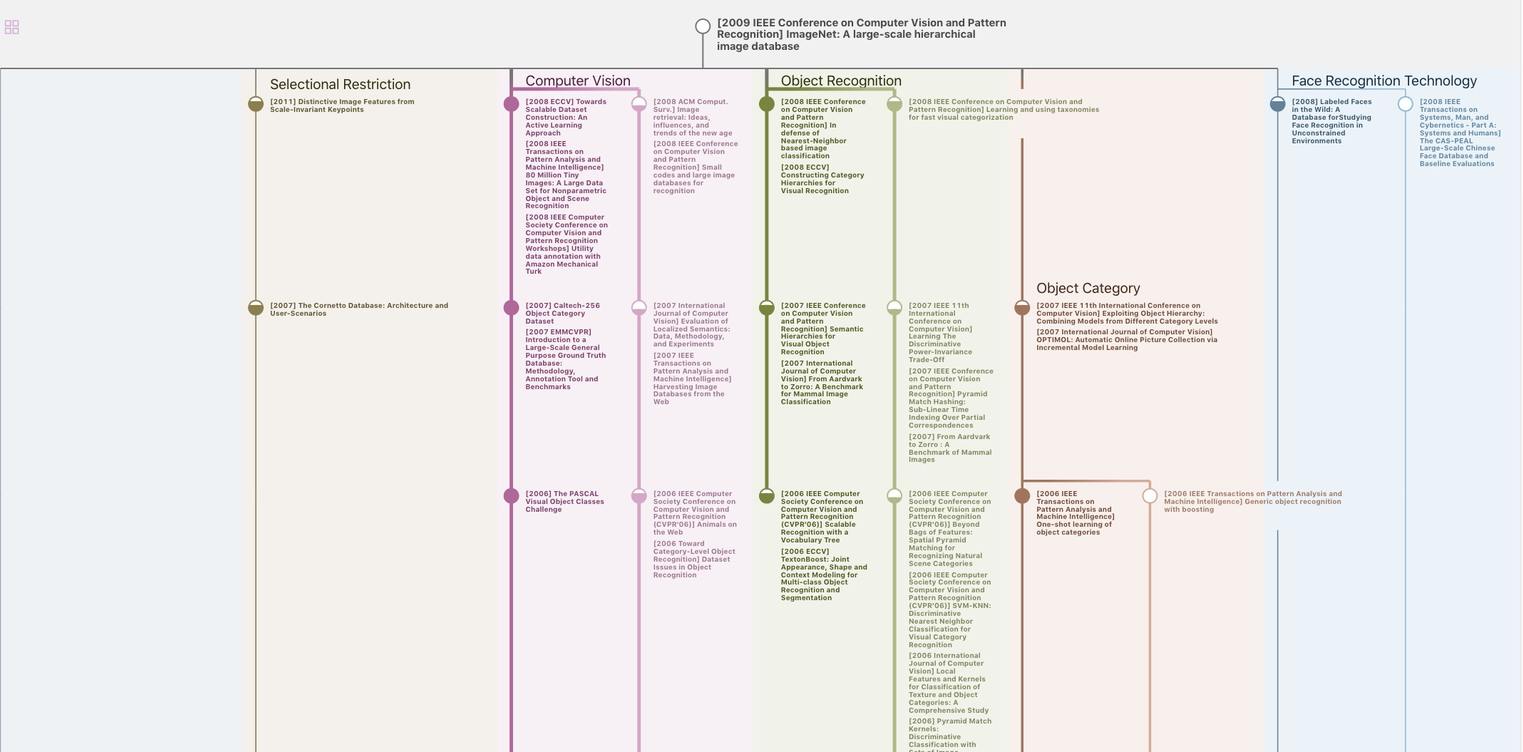
生成溯源树,研究论文发展脉络
Chat Paper
正在生成论文摘要